ad 2s
Computer Communications(2023)
摘要
With the widespread use of Internet applications, ensuring the quality and reliability of online services has become increasingly important. Therefore, anomaly detection methods play a critical role in identifying potential anomalies in the data streams of infrastructure systems and service applications. However, most of known detection methods have an underlying assumption that the data streams are continuous. In practice, we learn that many real-world data streams can be sporadic. It incurs particular challenges for the task of anomaly detection, for which the common preprocessing of downsampling on sporadic data can omit potential anomalies and delay alarms. In this paper, we propose an ensemble learning-based anomaly detection method on sporadic data streams named AD 2 S. It consists of two modules: a monitor module to continuously and adaptively determine the measure windows for observations, and a detection module that utilizes an isolation partition strategy to estimate the anomaly degree of each incoming observation. Based on experimental results on eight synthetic and public real-world datasets, our method outperforms other state-of-the-art methods with an average AUC score of 0.923. Additionally, our analysis demonstrates that the proposed method has constant amortized time and space complexity, enabling once detection within an average of 9.9 ms and maximum memory usage of 26.14 KB. The code of AD 2 S is open-sourced for further research.
更多查看译文
关键词
Anomaly detection,Sporadic data,Data streams,Quality of service
AI 理解论文
溯源树
样例
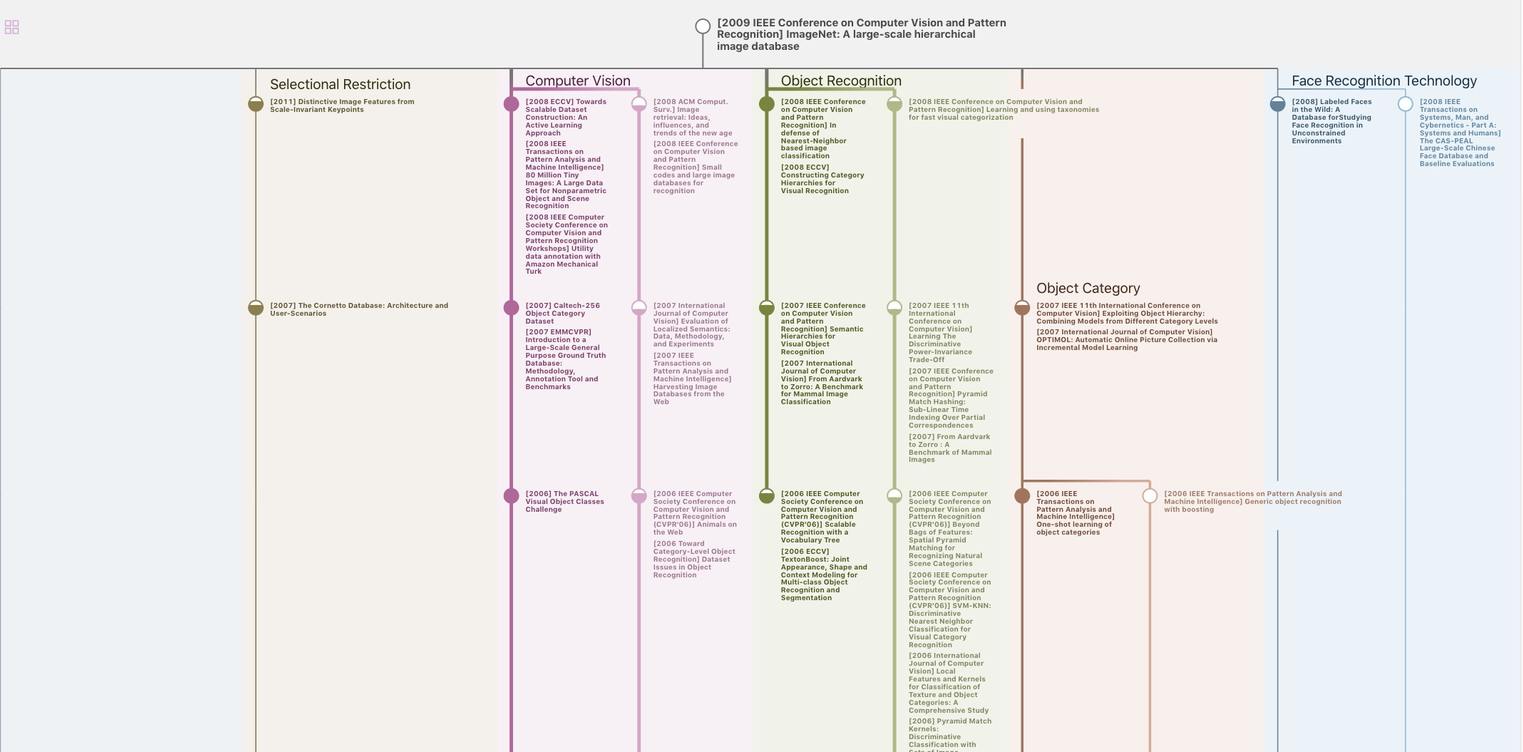
生成溯源树,研究论文发展脉络
Chat Paper
正在生成论文摘要