Reliable knowledge graph fact prediction via reinforcement learning
VISUAL COMPUTING FOR INDUSTRY BIOMEDICINE AND ART(2023)
摘要
Knowledge graph (KG) fact prediction aims to complete a KG by determining the truthfulness of predicted triples. Reinforcement learning (RL)-based approaches have been widely used for fact prediction. However, the existing approaches largely suffer from unreliable calculations on rule confidences owing to a limited number of obtained reasoning paths, thereby resulting in unreliable decisions on prediction triples. Hence, we propose a new RL-based approach named EvoPath in this study. EvoPath features a new reward mechanism based on entity heterogeneity, facilitating an agent to obtain effective reasoning paths during random walks. EvoPath also incorporates a new postwalking mechanism to leverage easily overlooked but valuable reasoning paths during RL. Both mechanisms provide sufficient reasoning paths to facilitate the reliable calculations of rule confidences, enabling EvoPath to make precise judgments about the truthfulness of prediction triples. Experiments demonstrate that EvoPath can achieve more accurate fact predictions than existing approaches.
更多查看译文
关键词
Knowledge graph,Fact prediction,Reinforcement learning,Entity heterogeneity,Postwalking mechanism
AI 理解论文
溯源树
样例
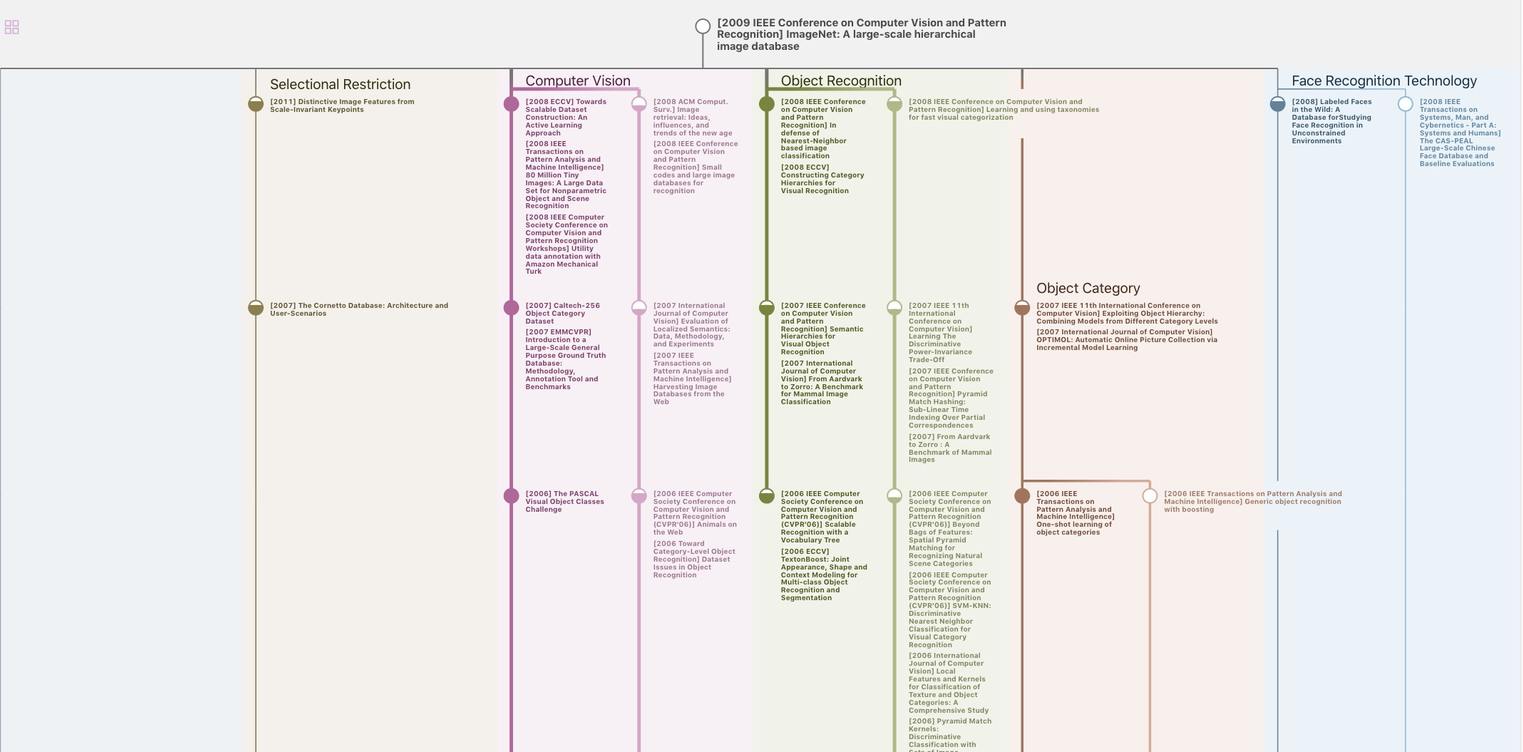
生成溯源树,研究论文发展脉络
Chat Paper
正在生成论文摘要