Information Theoretic Learning-Enhanced Dual-Generative Adversarial Networks With Causal Representation for Robust OOD Generalization
IEEE TRANSACTIONS ON NEURAL NETWORKS AND LEARNING SYSTEMS(2023)
摘要
Recently, machine/deep learning techniques are achieving remarkable success in a variety of intelligent control and management systems, promising to change the future of artificial intelligence (AI) scenarios. However, they still suffer from some intractable difficulty or limitations for model training, such as the out-of-distribution (OOD) issue, in modern smart manufacturing or intelligent transportation systems (ITSs). In this study, we newly design and introduce a deep generative model framework, which seamlessly incorporates the information theoretic learning (ITL) and causal representation learning (CRL) in a dual-generative adversarial network (Dual-GAN) architecture, aiming to enhance the robust OOD generalization in modern machine learning (ML) paradigms. In particular, an ITL-and CRL-enhanced Dual-GAN (ITCRL-DGAN) model is presented, which includes an autoencoder with CRL (AE-CRL) structure to aid the dual-adversarial training with causality-inspired feature representations and a Dual-GAN structure to improve the data augmentation in both feature and data levels. Following a newly designed feature separation strategy, a causal graph is built and improved based on the information theory, which can enhance the causally related factors among the separated core features and further enrich the feature representation with the counterfactual features via interventions based on the refined causal relationships. The ITL is incorporated to improve the extraction of low-dimensional feature representations and learn the optimized causal representations based on the idea of "information flow." A dual-adversarial training mechanism is then developed, which not only enables the generator to expand the boundary of feature distribution in accordance with the optimized feature representation from AE-CRL, but also allows the discriminator to further verify and improve the quality of the augmented data for OOD generalization. Experiment and evaluation results based on an open-source dataset demonstrate the outstanding learning efficiency and classification performance of our proposed model for robust OOD generalization in modern smart applications compared with three baseline methods.
更多查看译文
关键词
Autoencoder (AE),causal representation learning (CRL),deep learning,generative adversarial network (GAN),information theoretic learning (ITL),out-of-distribution (OOD)
AI 理解论文
溯源树
样例
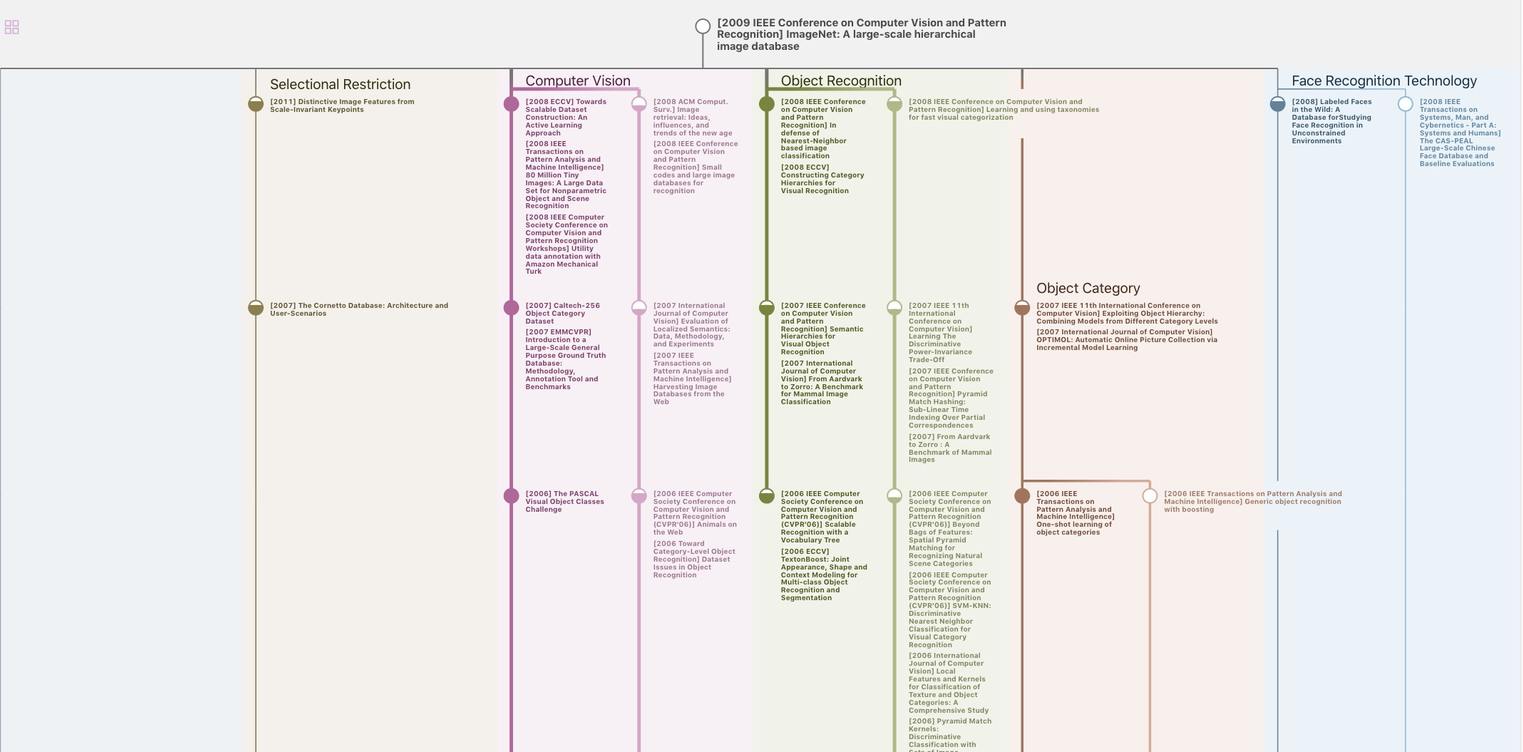
生成溯源树,研究论文发展脉络
Chat Paper
正在生成论文摘要