Applying Machine Learning Analysis Based on Proximal Femur of Abdominal Computed Tomography to Screen for Abnormal Bone Mass in Femur
Academic radiology(2023)
摘要
RATIONALE AND OBJECTIVES:To evaluate the performance of machine learning analysis based on proximal femur of abdominal computed tomography (CT) scans in screening for abnormal bone mass in femur.
MATERIALS AND METHODS:222 patients aged 50 years or older who underwent abdominal CT and dual-energy X-ray absorptiometry scans within 14 days were retrospectively enrolled. The patients were randomly assigned to a training cohort (n = 155) and a testing cohort (n = 67) in a ratio of 7:3. A total of 2288 candidate radiomic features were extracted from the volume region of interest - the left proximal femur of the abdominal CT scans. The most valuable radiomic features were selected using minimum-Redundancy Maximum-Relevancy and the least absolute shrinkage and selection operator to construct the radiomics model. The predictive performance was assessed with receiver operating characteristic curve.
RESULTS:13 features were chosen to establish the radiomics model. The radiomics model using logistic regression displayed excellent prediction performance in distinguishing normal bone mass and abnormal bone mass, with the area under the curve (AUC), accuracy, sensitivity and specificity of 0.917 (95% CI, 0.867-0.967), 0.826, 0.935 and 0.780 in the training cohort. The testing cohort indicated a better performance with AUC, accuracy, sensitivity and specificity of 0.963 (95% CI, 0.919-0.999), 0.851, 0.923 and 0.889.
CONCLUSION:The radiomics model based on proximal femur of abdominal CT scans had a high predictive performance to identify abnormal bone mass in femur, which can be used as a tool for opportunistic osteoporosis screening.
更多查看译文
关键词
Radiomics,Machine learning,Osteoporosis,Abdominal CT,DXA
AI 理解论文
溯源树
样例
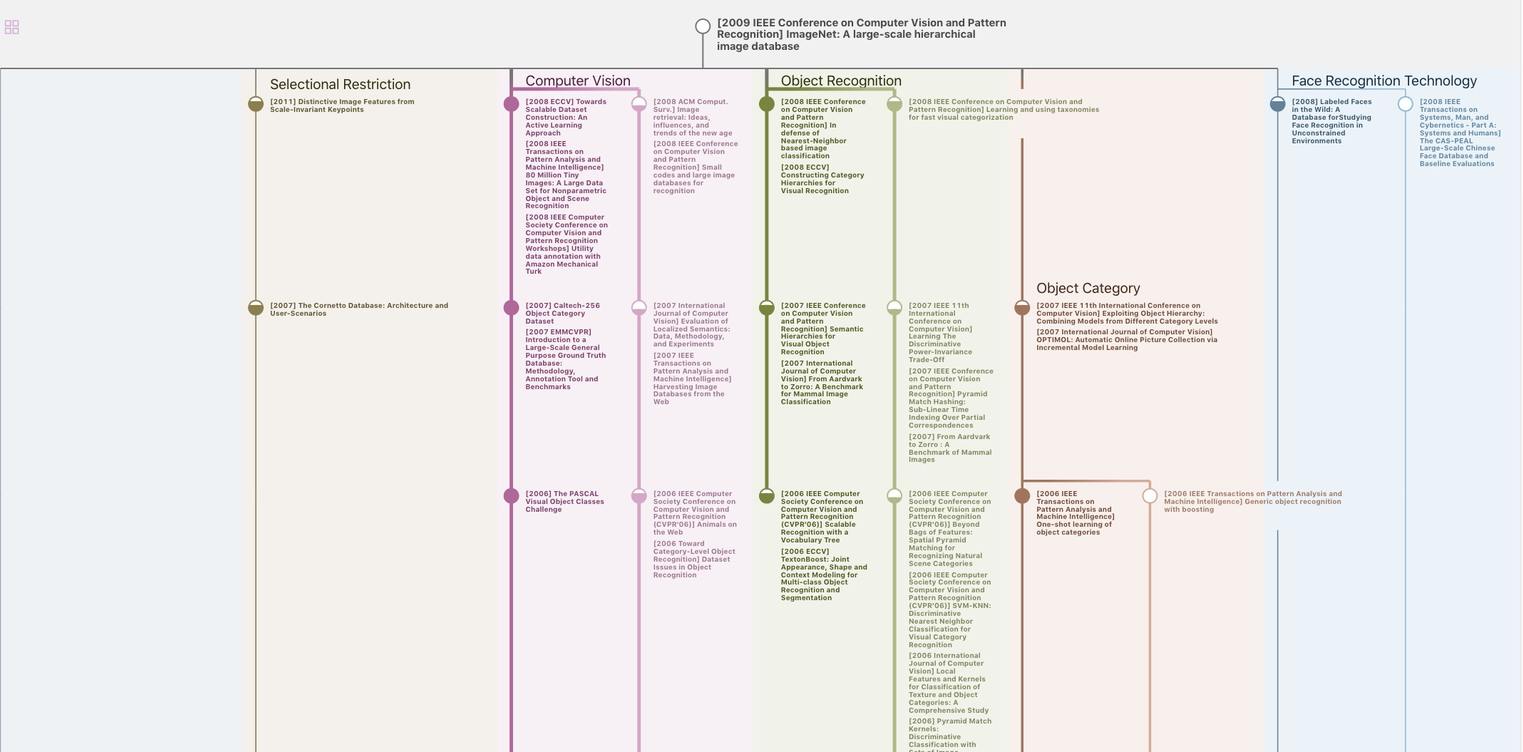
生成溯源树,研究论文发展脉络
Chat Paper
正在生成论文摘要