Developing deep learning-based strategies to predict the risk of hepatocellular carcinoma among patients with nonalcoholic fatty liver disease from electronic health records
Journal of Biomedical Informatics(2024)
摘要
Objective
The accuracy of deep learning models for many disease prediction problems is affected by time-varying covariates, rare incidence, covariate imbalance and delayed diagnosis when using structured electronic health records data. The situation is further exasperated when predicting the risk of one disease on condition of another disease, such as the hepatocellular carcinoma risk among patients with nonalcoholic fatty liver disease due to slow, chronic progression, the scarce of data with both disease conditions and the sex bias of the diseases. The goal of this study is to investigate the extent to which the aforementioned issues influence deep learning performance, and then devised strategies to tackle these challenges. These strategies were applied to improve hepatocellular carcinoma risk prediction among patients with nonalcoholic fatty liver disease.
Methods
We evaluated two representative deep learning models in the task of predicting the occurrence of hepatocellular carcinoma in a cohort of patients with nonalcoholic fatty liver disease (n = 220,838) from a national EHR database. The disease prediction task was carefully formulated as a classification problem while taking censorship and the length of follow-up into consideration.
Results
We developed a novel backward masking scheme to deal with the issue of delayed diagnosis which is very common in EHR data analysis and evaluate how the length of longitudinal information after the index date affects disease prediction. We observed that modeling time-varying covariates improved the performance of the algorithms and transfer learning mitigated reduced performance caused by the lack of data. In addition, covariate imbalance, such as sex bias in data impaired performance. Deep learning models trained on one sex and evaluated in the other sex showed reduced performance, indicating the importance of assessing covariate imbalance while preparing data for model training.
Conclusions
The strategies developed in this work can significantly improve the performance of hepatocellular carcinoma risk prediction among patients with nonalcoholic fatty liver disease. Furthermore, our novel strategies can be generalized to apply to other disease risk predictions using structured electronic health records, especially for disease risks on condition of another disease.
更多查看译文
关键词
Deep learning,Electronic health records,Sex bias,Hepatocellular carcinoma,Nonalcoholic fatty liver disease
AI 理解论文
溯源树
样例
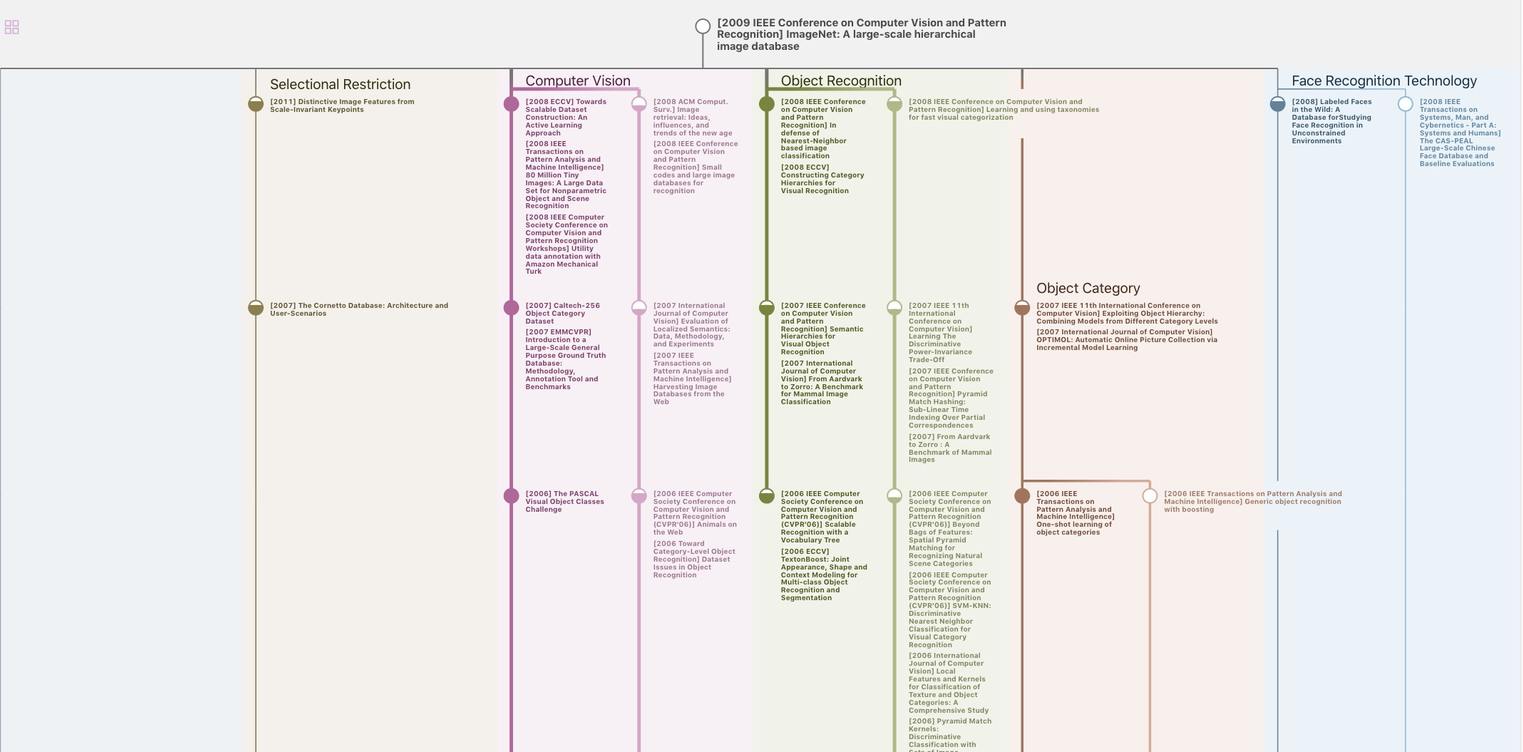
生成溯源树,研究论文发展脉络
Chat Paper
正在生成论文摘要