Steganography Embedding Cost Learning With Generative Multi-Adversarial Network
IEEE TRANSACTIONS ON INFORMATION FORENSICS AND SECURITY(2024)
摘要
Since the generative adversarial network (GAN) was proposed by Ian Goodfellow et al. in 2014, it has been widely used in various fields. However, there are only a few works related to image steganography so far. Existing GAN-based steganographic methods mainly focus on the design of generator, and just assign a relatively poorer steganalyzer in discriminator, which inevitably limits the performances of their models. In this paper, we propose a novel Steganographic method based on Generative Multi-Adversarial Network (Steg-GMAN) to enhance steganography security. Specifically, we first employ multiple steganalyzers rather than a single steganalyzer like existing methods to enhance the performance of discriminator. Furthermore, in order to balance the capabilities of the generator and the discriminator during training stage, we propose an adaptive way to update the parameters of the proposed GAN model according to the discriminant ability of different steganalyzers. In each iteration, we just update the poorest one among all steganalyzers in discriminator, while update the generator with the gradients derived from the strongest one. In this way, the performance of generator and discriminator can be gradually improved, so as to avoid training failure caused by gradient vanishing. Extensive comparative results show that the proposed method can achieve state-of-the-art results compared with the traditional steganography and the modern GAN-based steganographic methods. In addition, a large number of ablation experiments verify the rationality of the proposed model.
更多查看译文
关键词
Generators,Generative adversarial networks,Steganography,Costs,Security,Training,Distortion,generative adversarial network,steganalysis
AI 理解论文
溯源树
样例
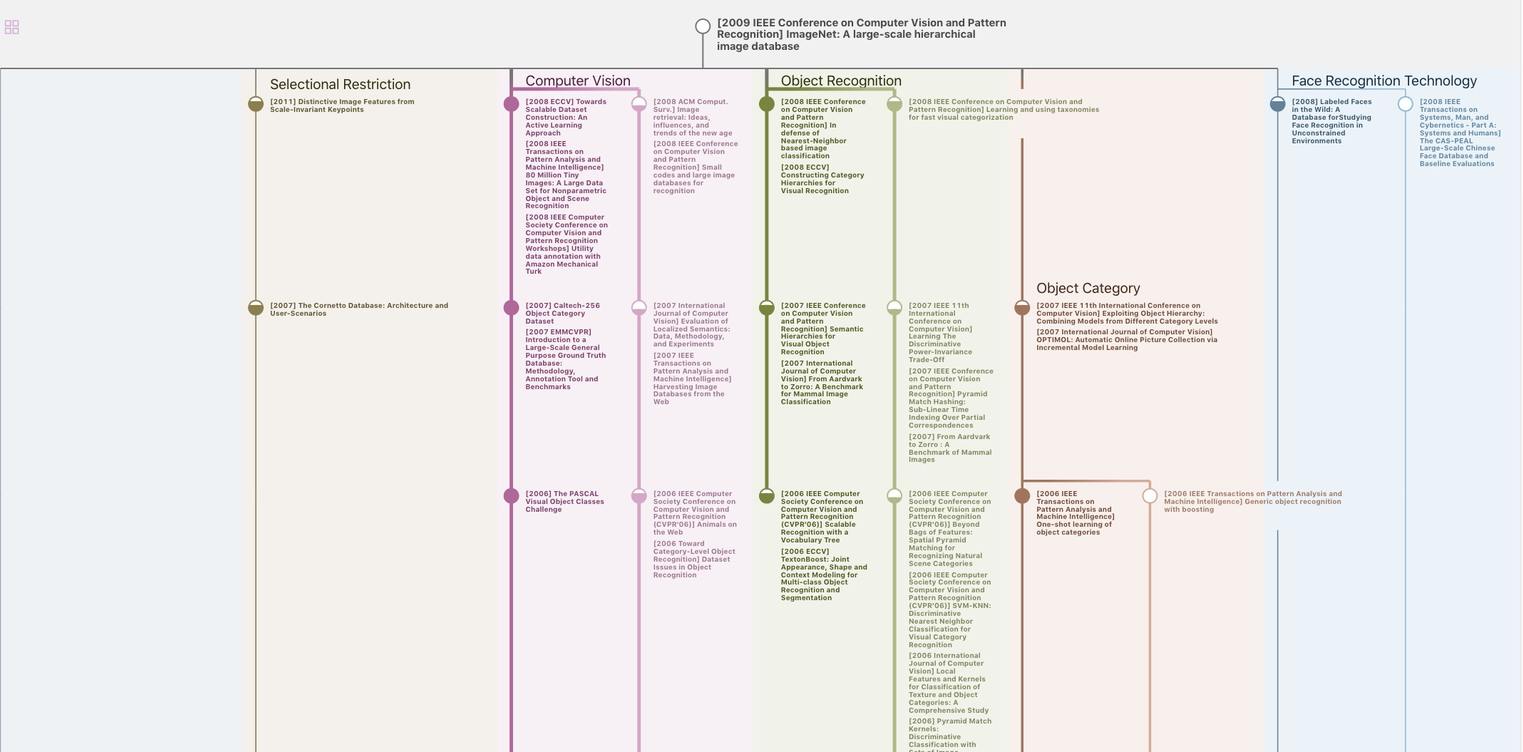
生成溯源树,研究论文发展脉络
Chat Paper
正在生成论文摘要