Image guided construction of a common coordinate framework for spatial transcriptome data
bioRxiv (Cold Spring Harbor Laboratory)(2023)
摘要
Spatial transcriptomics is a powerful technology for high-resolution mapping of gene expression in tissue samples, enabling a molecular level understanding of tissue architecture. The acquisition entails dissecting and profiling micron-thick tissue slices, with multiple slices often needed for a comprehensive study. However, the lack of a common coordinate framework (CCF) among slices, due to slicing and displacement variations, can hinder data analysis, making data comparison and integration challenging, and potentially compromising analysis accuracy. Here we present a deep learning algorithm STaCker that unifies the coordinates of transcriptomic slices via an image registration process. STaCker derives a composite image representation by integrating tissue image and gene expressions that are transformed to be resilient to noise and batch effects. Trained exclusively on diverse synthetic data, STaCker overcomes the training data scarcity and is applicable to any tissue type. Its performance on various benchmarking datasets shows a significant increase in spatial concordance in aligned slices, surpassing existing methods. STaCker also successfully harmonizes multiple real spatial transcriptome datasets. These results indicate that STaCker is a valuable computational tool for constructing a CCF with spatial transcriptome data.
### Competing Interest Statement
P.L., S.M., Y.B., and G.S.A. have filed a patent application relating to the STaCker computational framework. The remaining authors are employees and shareholders of Regeneron Pharmaceuticals, although the manuscript's subject matter does not have any relationship to any products or services of this corporation.
更多查看译文
AI 理解论文
溯源树
样例
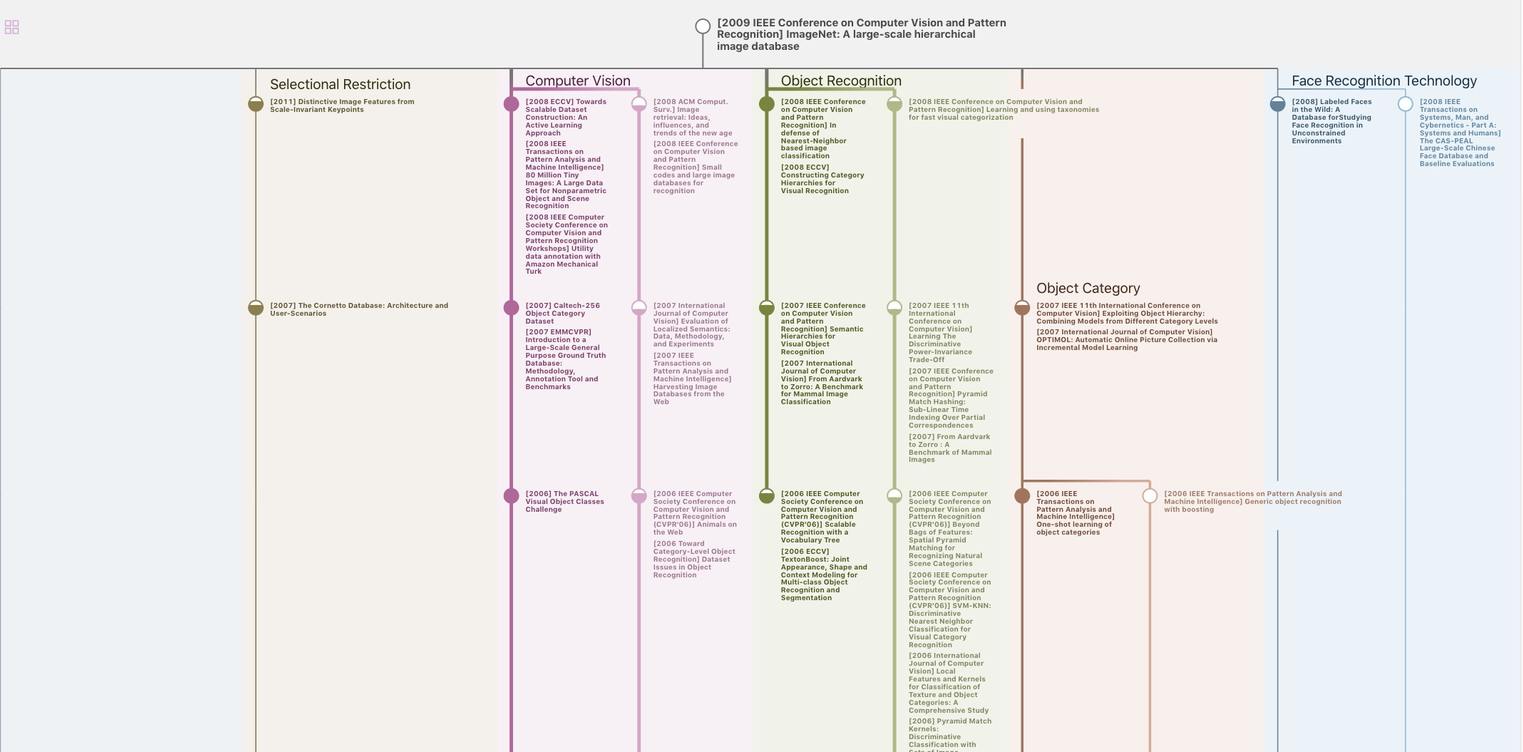
生成溯源树,研究论文发展脉络
Chat Paper
正在生成论文摘要