Noise reduction as a unified mechanism of perceptual learning in humans, macaques, and convolutional neural networks
bioRxiv (Cold Spring Harbor Laboratory)(2023)
摘要
It is established that perceptual learning enhances the signal-to-noise ratios of sensory processing. However, the specific mechanisms behind this improvement remain enigmatic. Here, we systematically investigated these mechanisms by analyzing population activity changes in deep convolutional neural networks (DCNNs), humans, and macaques. We first trained DCNNs on orientation and motion direction discrimination tasks. Our models successfully replicated the behavioral signature of enhanced signal-to-noise ratio, and the individual and population response changes observed in numerous empirical studies. We then devised a method to quantify all scenarios in which learning modifies the tuning and covariance structure of neuronal populations to enhance sensory discrimination. A groundbreaking revelation is that enhanced signal-to-noise ratios are primarily driven by reduced trial-by-trial response variability. This finding was strongly supported by population response changes measured in visual areas of both humans and macaques. These results elucidate the shared mechanisms of neural plasticity in biological and artificial visual systems.
### Competing Interest Statement
Dr. Xing Chen a cofounder and shareholder of a neurotechnology start-up, Phosphoenix (Netherlands). Other authors declare no competing financial interests.
更多查看译文
AI 理解论文
溯源树
样例
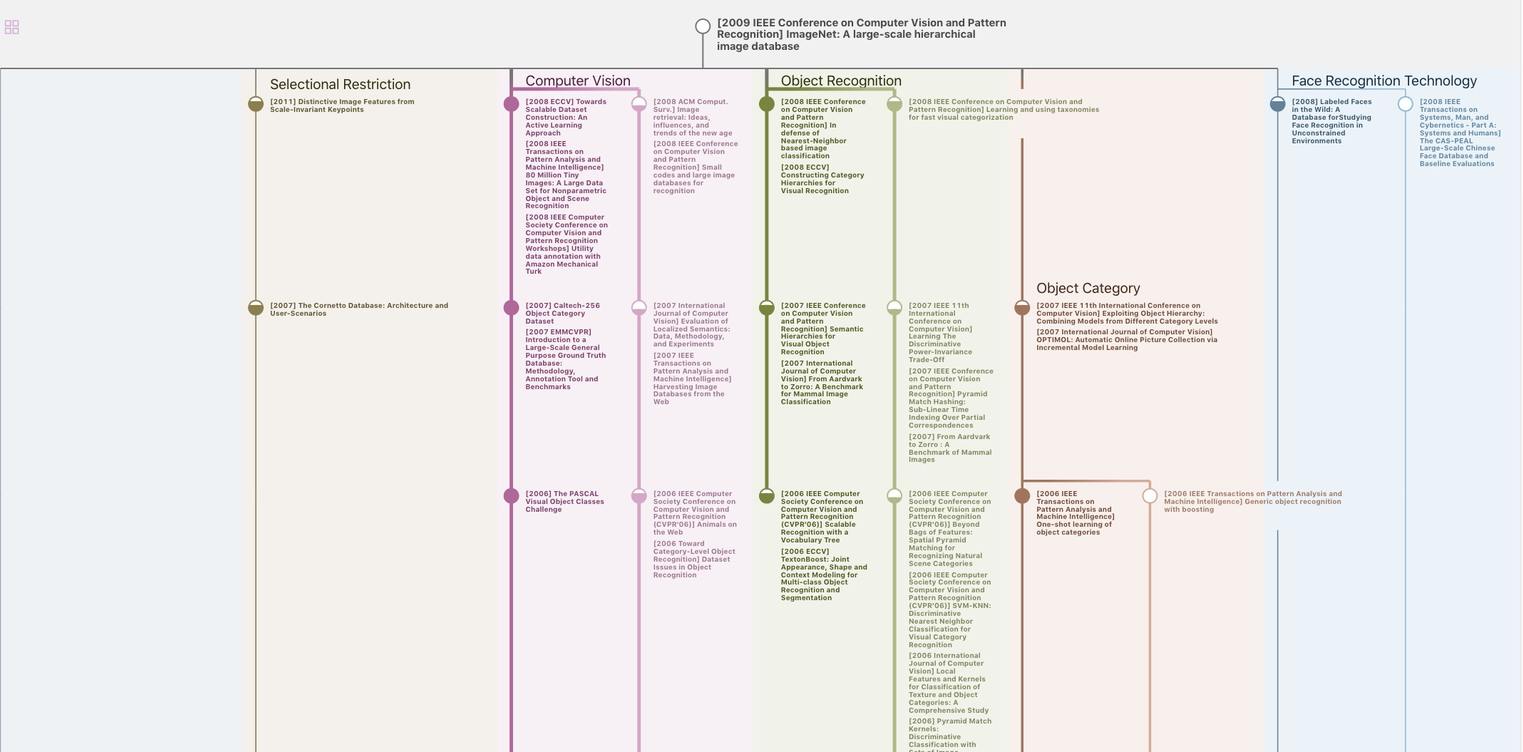
生成溯源树,研究论文发展脉络
Chat Paper
正在生成论文摘要