How Far Can We Extract Diverse Perspectives from Large Language Models?
CoRR(2023)
摘要
Collecting diverse human opinions is costly and challenging. This leads to a
recent trend in collaborative efforts between humans and Large Language Models
(LLMs) for generating diverse data, offering potential scalable and efficient
solutions. However, the extent of LLMs' capability to generate diverse
perspectives on subjective topics remains an unexplored question. In this
study, we investigate LLMs' capacity for generating diverse perspectives and
rationales on subjective topics, such as social norms and argumentative texts.
We formulate a new problem of maximum diversity extraction from LLMs. Motivated
by how humans develop their opinions through their values, we propose a
criteria-based prompting technique to ground diverse opinions. To see how far
we can extract diverse perspectives from LLMs, or called diversity coverage, we
employ a step-by-step recall prompting for generating more outputs from the
model in an iterative manner. As we apply our methods to various tasks, indeed
we find that LLMs can generate diverse opinions according to the degree of task
subjectivity
更多查看译文
AI 理解论文
溯源树
样例
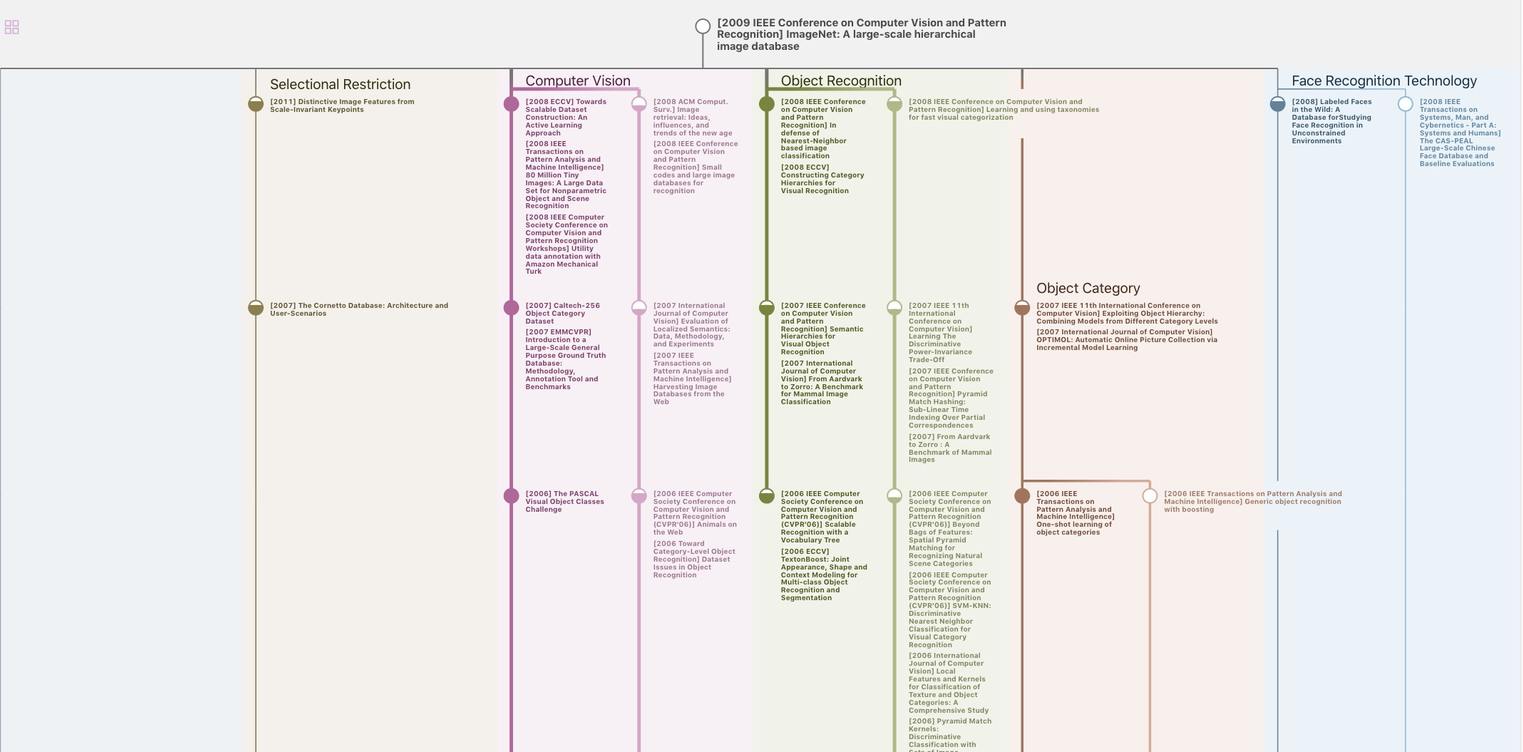
生成溯源树,研究论文发展脉络
Chat Paper
正在生成论文摘要