Open-Sourcing Highly Capable Foundation Models: An evaluation of risks, benefits, and alternative methods for pursuing open-source objectives.
CoRR(2023)
摘要
Recent decisions by leading AI labs to either open-source their models or to restrict access to their models has sparked debate about whether, and how, increasingly capable AI models should be shared. Open-sourcing in AI typically refers to making model architecture and weights freely and publicly accessible for anyone to modify, study, build on, and use. This offers advantages such as enabling external oversight, accelerating progress, and decentralizing control over AI development and use. However, it also presents a growing potential for misuse and unintended consequences. This paper offers an examination of the risks and benefits of open-sourcing highly capable foundation models. While open-sourcing has historically provided substantial net benefits for most software and AI development processes, we argue that for some highly capable foundation models likely to be developed in the near future, open-sourcing may pose sufficiently extreme risks to outweigh the benefits. In such a case, highly capable foundation models should not be open-sourced, at least not initially. Alternative strategies, including non-open-source model sharing options, are explored. The paper concludes with recommendations for developers, standard-setting bodies, and governments for establishing safe and responsible model sharing practices and preserving open-source benefits where safe.
更多查看译文
关键词
capable foundation models,risks,open-sourcing,open-source
AI 理解论文
溯源树
样例
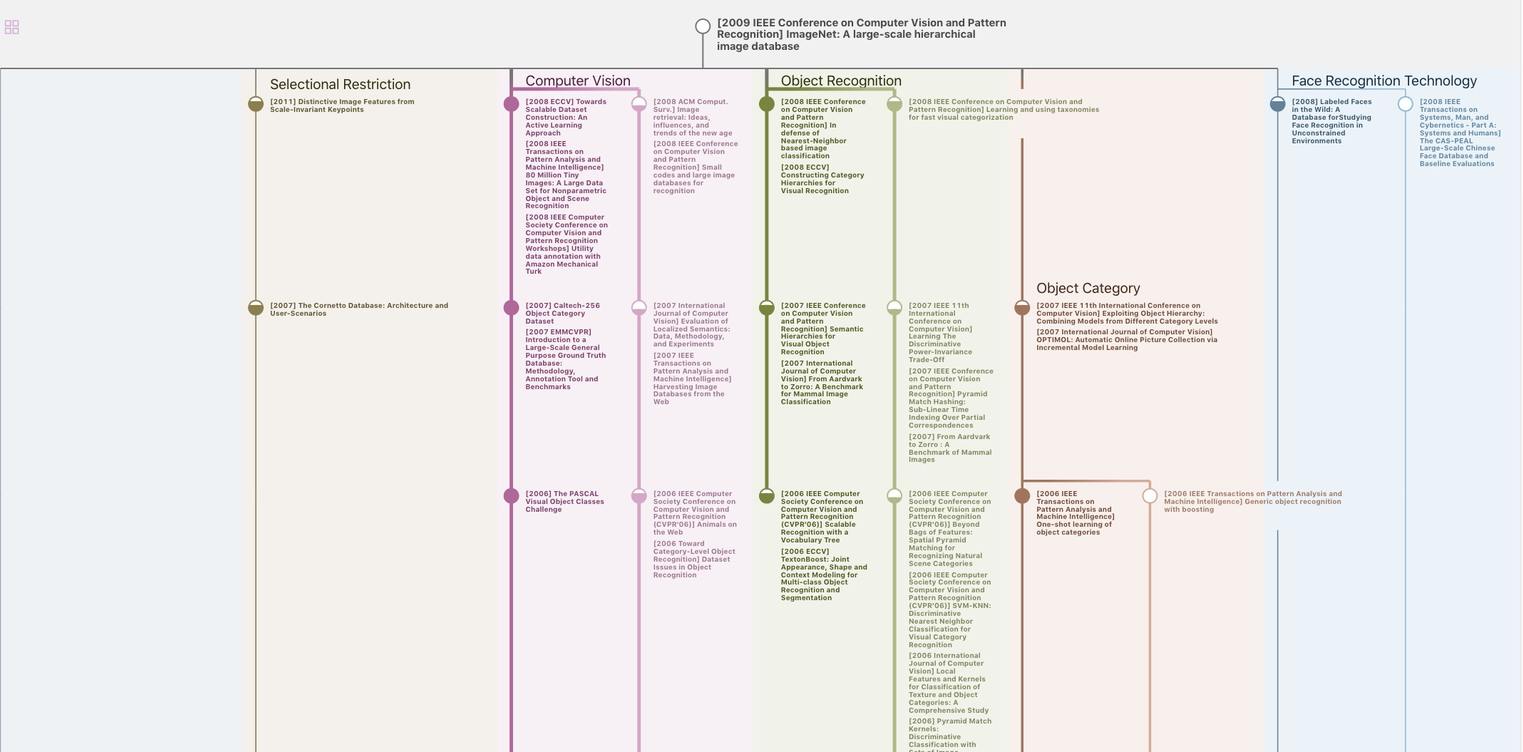
生成溯源树,研究论文发展脉络
Chat Paper
正在生成论文摘要