A method for yarn quality fluctuation prediction based on multi-correlation parameter feature subspace mechanism in spinning process
Journal of Engineered Fibers and Fabrics(2023)
摘要
Yarn quality is a key indicator in spinning production process, which directly determines the processing quality of the finished fabric. Considering the problems that the yarn quality is difficult to predict due to many influencing factors and widely monitoring dimensions existed. This paper proposes a multi-correlation parameters-based feature subspace model for yarn quality fluctuation prediction method. Firstly, Kernel Principal Component Analysis (KPCA) algorithm is used to analyze the relationship between the relevant parameters affecting yarn quality, and the characteristic subspace of yarn quality fluctuation is reconstructed. Then, a feature subspace-oriented deep learning prediction structure based on Deep Belief Network (DBN) is constructed, and the intelligent prediction model of yarn quality is established by using Particle Swarm Optimization (PSO) algorithm to optimize the modeling parameters. Finally, a case for spinning spun yarn process data set is used to validate the effectiveness of the proposed method, result shows that the proposed method can effectively analyze the multi-correlation parameters fluctuation law, which shows good effect for predicting yarn quality accurately.
更多查看译文
关键词
Multi-correlation parameter, feature subspace, Deep Belief Network, quality, yarn
AI 理解论文
溯源树
样例
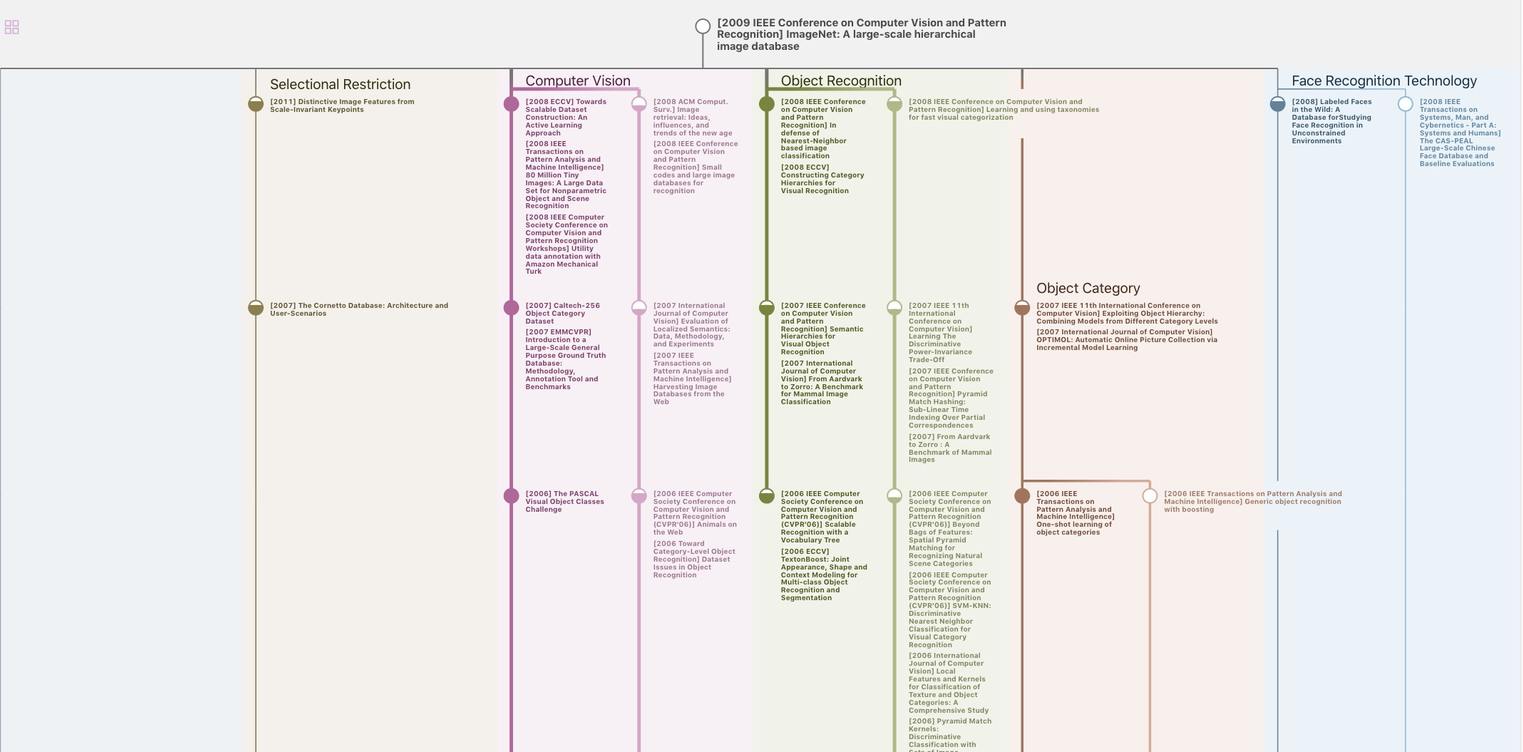
生成溯源树,研究论文发展脉络
Chat Paper
正在生成论文摘要