Advancing Limited Data Text-to-Speech Synthesis: Non-Autoregressive Transformer for High-Quality Parallel Synthesis
2023 International Conference on Speech Technology and Human-Computer Dialogue (SpeD)(2023)
摘要
Despite the impressive results achieved by autoregressive generative models like Tacotron2 in end-to-end speech synthesis, their slow inference speed remains a significant drawback. To overcome this limitation, non-autoregressive Text-to-Speech (TTS) models like FastSpeech2 and neural vocoders like AutoVocoder, have emerged as faster alternatives with comparable quality. In this work, we present a novel lightweight Arabic TTS system based on a transformer architecture that utilizes fewer parameters than Tacotron2. Our approach combines convolutional and transformer-based blocks and is fully based on a non-autoregressive training framework. Our system can accurately reproduce the characteristics of natural speech like tone, pitch, timing and word pronunciation with state-of-the-art quality, making it suitable for practical applications like speech synthesis for low-resource languages and conversational agents. Our method is validated by acoustic analysis and subjective listening tests.
更多查看译文
关键词
speech synthesis,neural vocoder,text-to-speech,generative adversarial network,acoustic analysis
AI 理解论文
溯源树
样例
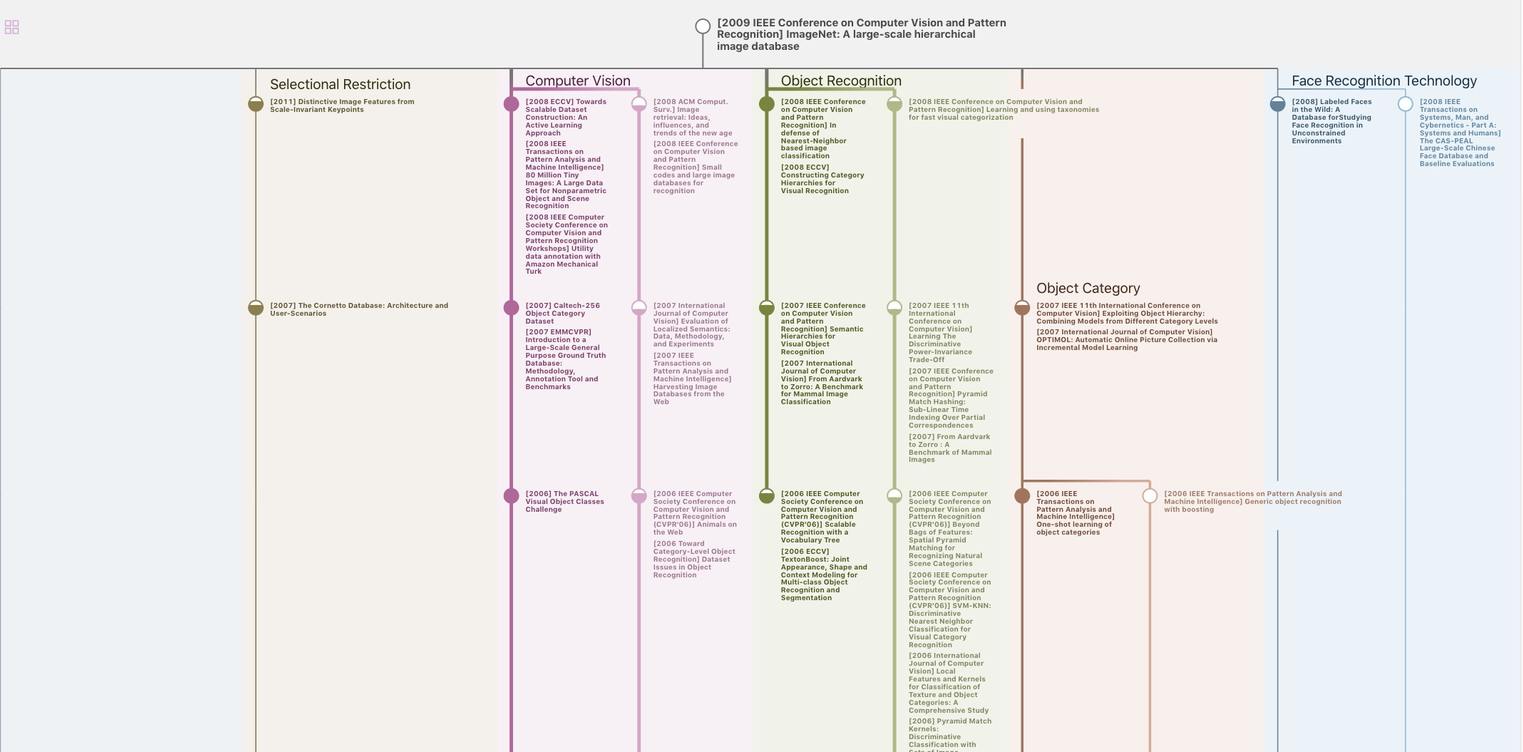
生成溯源树,研究论文发展脉络
Chat Paper
正在生成论文摘要