Improving Social Bot Detection Through Aid and Training
HUMAN FACTORS(2023)
摘要
Objective: We test the effects of three aids on individuals' ability to detect social bots among Twitter personas: a bot indicator score, a training video, and a warning. Background: Detecting social bots can prevent online deception. We use a simulated social media task to evaluate three aids. Method: Lay participants judged whether each of 60 Twitter personas was a human or social bot in a simulated online environment, using agreement between three machine learning algorithms to estimate the probability of each persona being a bot. Experiment 1 compared a control group and two intervention groups, one provided a bot indicator score for each tweet; the other provided a warning about social bots. Experiment 2 compared a control group and two intervention groups, one receiving the bot indicator scores and the other a training video, focused on heuristics for identifying social bots. Results: The bot indicator score intervention improved predictive performance and reduced overconfidence in both experiments. The training video was also effective, although somewhat less so. The warning had no effect. Participants rarely reported willingness to share content for a persona that they labeled as a bot, even when they agreed with it. Conclusions: Informative interventions improved social bot detection; warning alone did not. Application: We offer an experimental testbed and methodology that can be used to evaluate and refine interventions designed to reduce vulnerability to social bots. We show the value of two interventions that could be applied in many settings.
更多查看译文
关键词
signal detection theory,social bots,social media,decision support,training
AI 理解论文
溯源树
样例
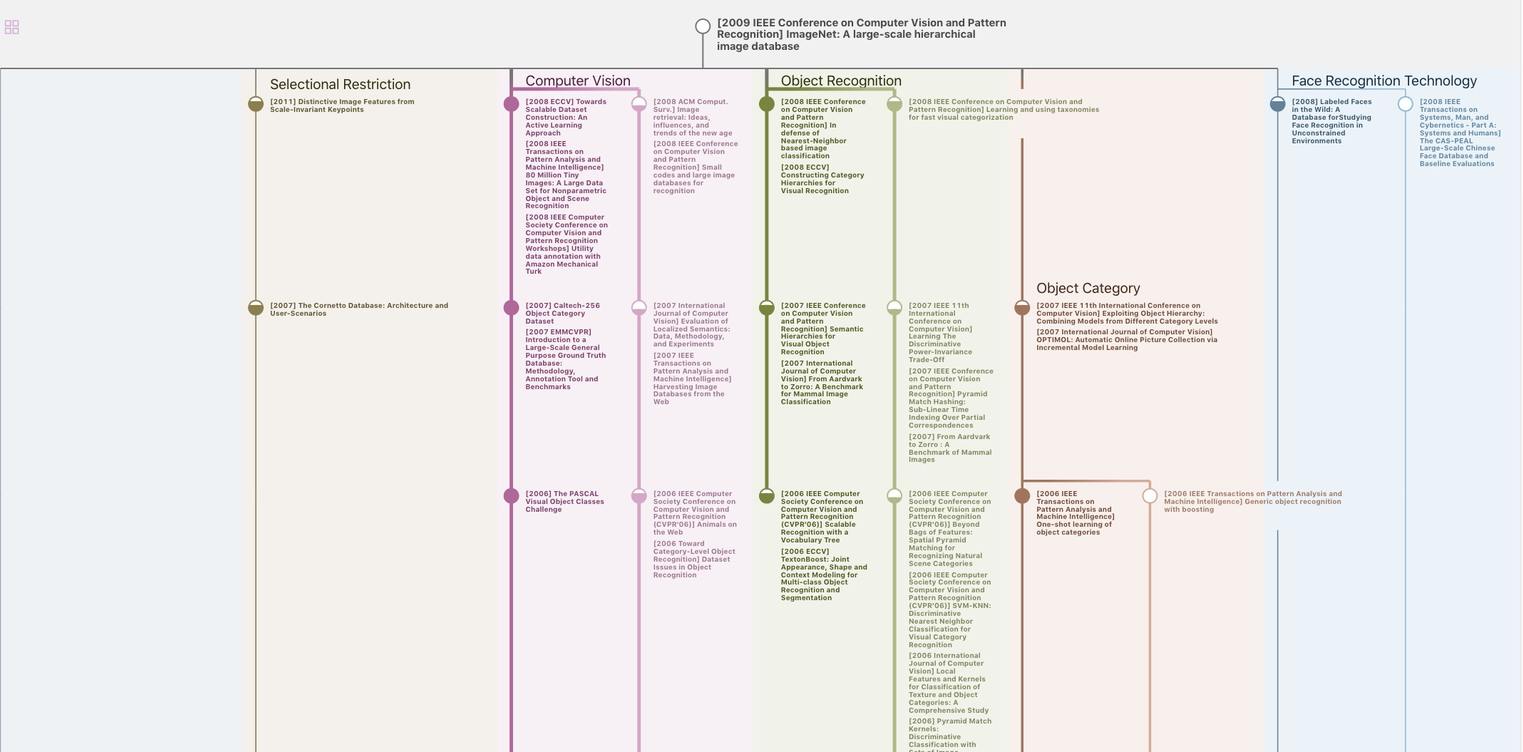
生成溯源树,研究论文发展脉络
Chat Paper
正在生成论文摘要