Fine-Grained Spatio-Temporal Parsing Network for Action Quality Assessment
IEEE TRANSACTIONS ON IMAGE PROCESSING(2023)
摘要
Action Quality Assessment (AQA) plays an important role in video analysis, which is applied to evaluate the quality of specific actions, i.e., sports activities. However, it is still challenging because there are lots of small action discrepancies with similar backgrounds, but current approaches mostly adopt holistic video representations. So that fine-grained intra-class variations are unable to be captured. To address the aforementioned challenge, we propose a Fine-grained Spatio-temporal Parsing Network (FSPN) which is composed of the intra-sequence action parsing module and spatiotemporal multiscale transformer module to learn fine-grained spatiotemporal sub-action representations for more reliable AQA. The intra-sequence action parsing module performs semantical sub-action parsing by mining sub-actions at fine-grained levels. It enables a correct description of the subtle differences between action sequences. The spatiotemporal multiscale transformer module learns motion-oriented action features and obtains their long-range dependencies among sub-actions at different scales. Furthermore, we design a group contrastive loss to train the model and learn more discriminative feature representations for sub-actions without explicit supervision. We exhaustively evaluate our proposed approach in the FineDiving, AQA-7, and MTL-AQA datasets. Extensive experiment results demonstrate the effectiveness and feasibility of our proposed approach, which outperforms the state-of-the-art methods by a significant margin.
更多查看译文
关键词
Transformers,Spatiotemporal phenomena,Semantics,Decoding,Task analysis,Sports,Quality assessment,Action quality assessment,fine-grained representation,multiscale transformer,action parsing
AI 理解论文
溯源树
样例
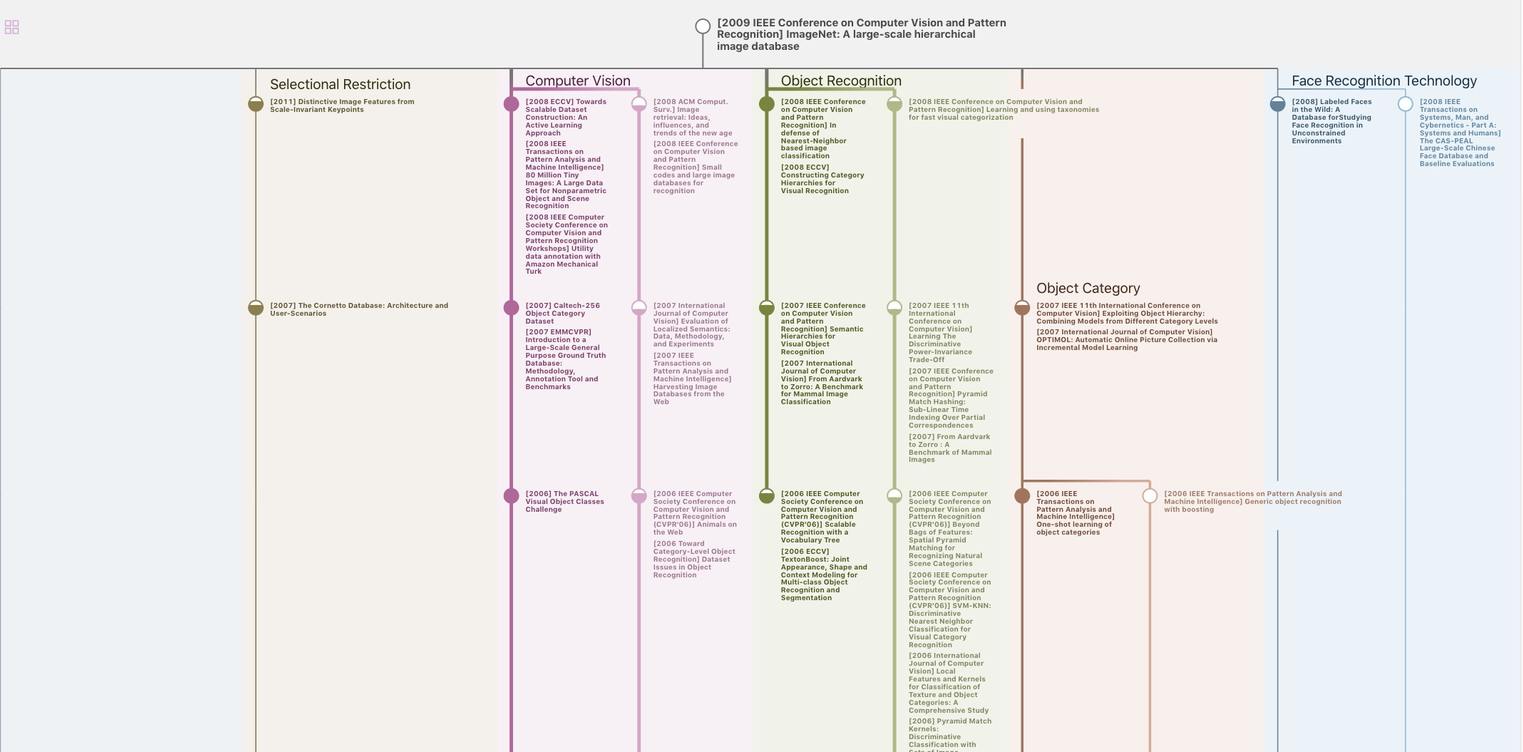
生成溯源树,研究论文发展脉络
Chat Paper
正在生成论文摘要