Cross Device Federated Intrusion Detector for Early Stage Botnet Propagation in IoT.
CoRR(2023)
摘要
A botnet is an army of zombified computers infected with malware and controlled by malicious actors to carry out tasks such as Distributed Denial of Service (DDoS) attacks. Billions of Internet of Things (IoT) devices are primarily targeted to be infected as bots since they are configured with weak credentials or contain common vulnerabilities. Detecting botnet propagation by monitoring the network traffic is difficult as they easily blend in with regular network traffic. The traditional machine learning (ML) based Intrusion Detection System (IDS) requires the raw data to be captured and sent to the ML processor to detect intrusion. In this research, we examine the viability of a cross-device federated intrusion detection mechanism where each device runs the ML model on its data and updates the model weights to the central coordinator. This mechanism ensures the client's data is not shared with any third party, terminating privacy leakage. The model examines each data packet separately and predicts anomalies. We evaluate our proposed mechanism on a real botnet propagation dataset called MedBIoT. Overall, the proposed method produces an average accuracy of 71%, precision 78%, recall 71%, and f1-score 68%. In addition, we also examined whether any device taking part in federated learning can employ a poisoning attack on the overall system.
更多查看译文
AI 理解论文
溯源树
样例
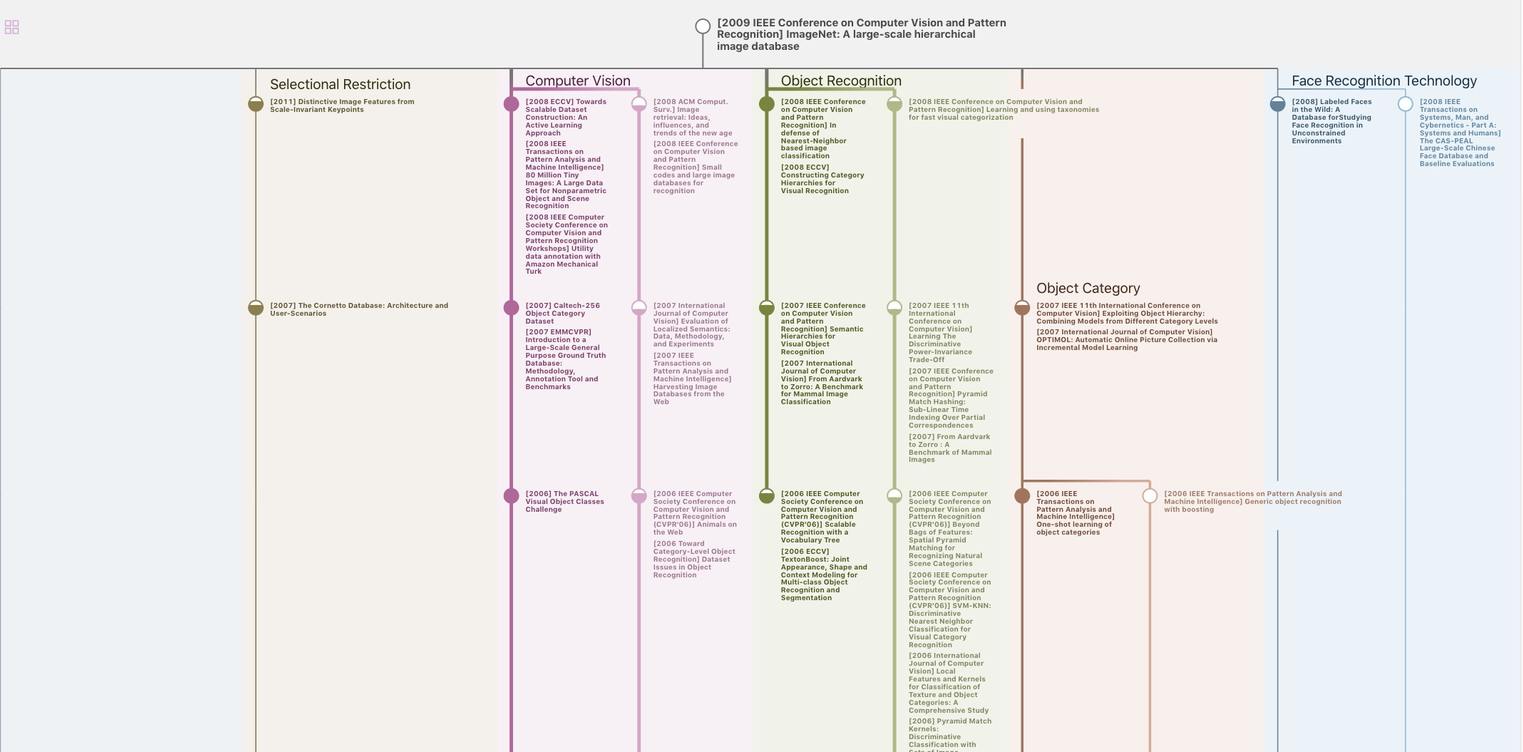
生成溯源树,研究论文发展脉络
Chat Paper
正在生成论文摘要