Rankitect: Ranking Architecture Search Battling World-class Engineers at Meta Scale.
CoRR(2023)
摘要
Neural Architecture Search (NAS) has demonstrated its efficacy in computer vision and potential for ranking systems. However, prior work focused on academic problems, which are evaluated at small scale under well-controlled fixed baselines. In industry system, such as ranking system in Meta, it is unclear whether NAS algorithms from the literature can outperform production baselines because of: (1) scale - Meta ranking systems serve billions of users, (2) strong baselines - the baselines are production models optimized by hundreds to thousands of world-class engineers for years since the rise of deep learning, (3) dynamic baselines - engineers may have established new and stronger baselines during NAS search, and (4) efficiency - the search pipeline must yield results quickly in alignment with the productionization life cycle. In this paper, we present Rankitect, a NAS software framework for ranking systems at Meta. Rankitect seeks to build brand new architectures by composing low level building blocks from scratch. Rankitect implements and improves state-of-the-art (SOTA) NAS methods for comprehensive and fair comparison under the same search space, including sampling-based NAS, one-shot NAS, and Differentiable NAS (DNAS). We evaluate Rankitect by comparing to multiple production ranking models at Meta. We find that Rankitect can discover new models from scratch achieving competitive tradeoff between Normalized Entropy loss and FLOPs. When utilizing search space designed by engineers, Rankitect can generate better models than engineers, achieving positive offline evaluation and online A/B test at Meta scale.
更多查看译文
AI 理解论文
溯源树
样例
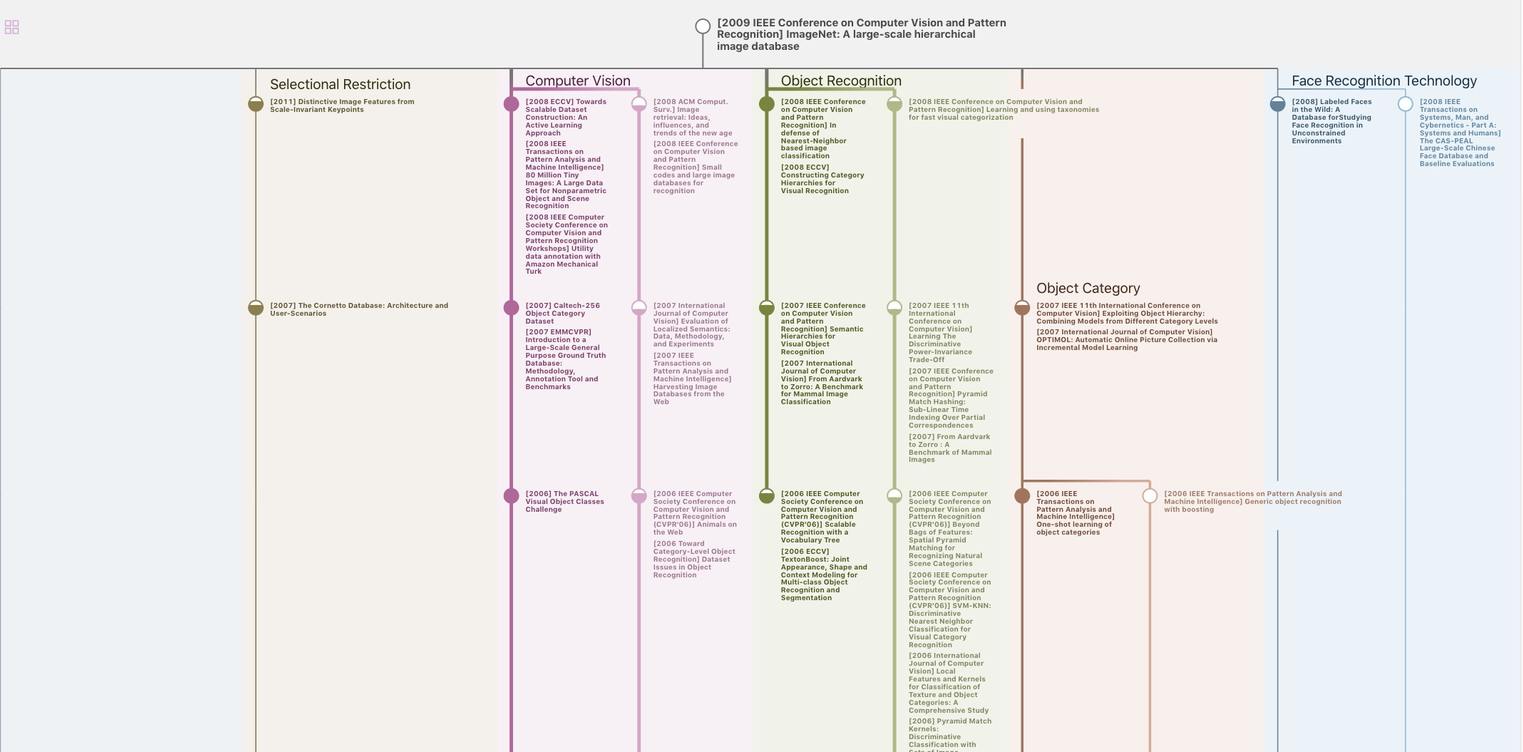
生成溯源树,研究论文发展脉络
Chat Paper
正在生成论文摘要