Towards Multi-Functional ECG Smart System Based on a Client-Edge-Cloud Architecture
2023 IEEE EMBS International Conference on Biomedical and Health Informatics (BHI)(2023)
摘要
This paper presents a novel client-edge-cloud-based framework that integrates the learning of task-invariant ECG feature representations from ultra-short ECG segments (<10 sec) and subsequent training of task-specific machine learning (ML) classifiers for different applications. Our proposed framework removes the need for application-specific ECG processing by training a general ECG representation learner in a self-supervised manner. The ECG representation learner is then used for generating feature inputs for the different task-specific applications. The proposed framework distributes the computation across cloud, edge, and client components depending on the resource requirement and time criticality. We demonstrate the feasibility and promise of the proposed approach on two different applications, that is, acute stress type classification, and biometric user identification and authentication. The use cases were analyzed using the computational parameters for the different models and computational tasks along with the overall performance. Our analyses show that the application-specific ML models can perform real-time inference in less than a second and the training time of the ML classifiers at the edge devices are in the order of 10-20 seconds. In the future, the proposed framework can be utilized for developing reliable, secure, and multi-functional ECG-based smart systems.
更多查看译文
关键词
Acute stress,Electrocardiogram (ECG),Contrastive Learning,Smart Healthcare,Biometrics,convolutional neural network (CNN),Client-Edge-Cloud,Wearable Computing
AI 理解论文
溯源树
样例
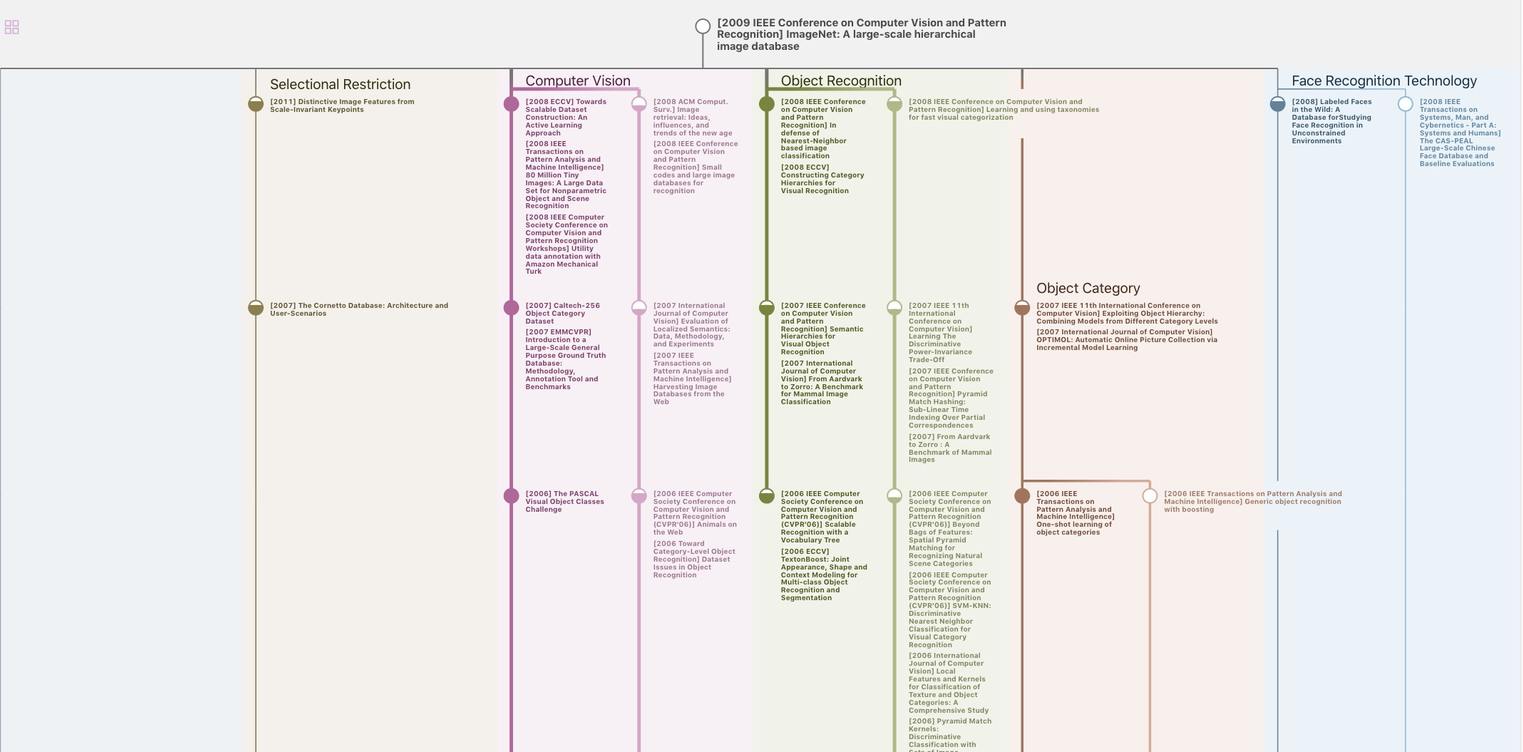
生成溯源树,研究论文发展脉络
Chat Paper
正在生成论文摘要