SiaKey: A Method for Improving Few-shot Learning with Clinical Domain Information
2023 IEEE EMBS INTERNATIONAL CONFERENCE ON BIOMEDICAL AND HEALTH INFORMATICS, BHI(2023)
摘要
Supervised Natural Language Processing (NLP) models can achieve high accuracy, but they often require a significant amount of annotated data for training, which can be expensive and time-consuming. This is especially true for clinical NLP, where annotations on large-scale electronic health records (EHRs) and online posts necessitate specialists with clinical expertise. On the other hand, fine-tuning Pretrained Language Models (PLMs) may yield poor performance due to limited training data. Few-Shot Learning (FSL) methods offer a promising solution as they can significantly improve clinical NLP with only a small amount of labeled data. In this paper, we introduce a novel FSL technique named SiaKey, which utilizes Siamese Networks and integrates Keyphrases Extraction and Domain Knowledge, for the task of online post classification. This task is challenging since online posts typically contain a greater amount of irrelevant information compared to traditional EHRs. By incorporating Keyphrases using domain knowledge, we extract essential information and reduce distractions, enhancing the classification process. To evaluate SiaKey's performance, we conducted tests with 5, 10, 15, and 20-shot learning on health-related online post-classification tasks. The results of our experiments demonstrate SiaKey's effectiveness in capturing text features, showcasing its superior performance compared to BioBERT on similar FSL tasks.
更多查看译文
关键词
few-shot learning,keyphrases extraction,clinical domain knowledge,social media
AI 理解论文
溯源树
样例
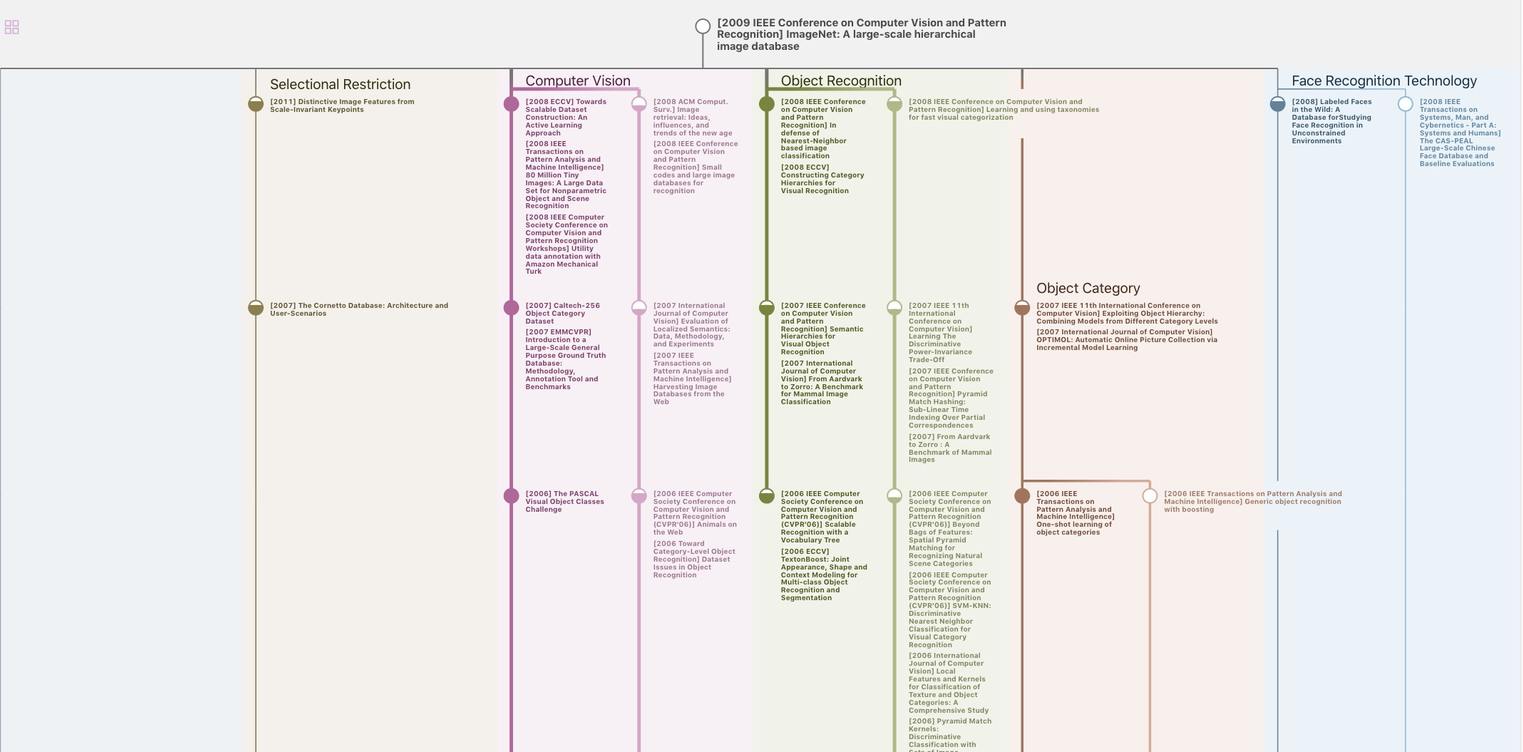
生成溯源树,研究论文发展脉络
Chat Paper
正在生成论文摘要