Generalized Approximate Message Passing Based Bayesian Learning Detectors for Uplink Grant-Free NOMA
IEEE TRANSACTIONS ON VEHICULAR TECHNOLOGY(2023)
摘要
The existing sparse Bayesian learning (SBL) and pattern coupled sparse Bayesian learning (PCSBL) multiuser detection (MUD) algorithms for grant-free non-orthogonal multiple access (GF-NOMA) have high computational complexity, i.e. O(NK2), considering mainly the calculation of posterior distribution of the transmitted signals. In this paper, we embed generalized approximate message passing (GAMP) to SBL and PCSBL, and develop two efficient Bayesian learning algorithms for GF-NOMA systems, that is, generalized approximate message passing sparse Bayesian learning (GAMP-SBL) and generalized approximate message passing pattern coupled sparse Bayesian learning (GAMP-PCSBL). It is shown that the Bayesian algorithms can significantly reduce the computational complexity fromO(NK2) toO(NK). Simulation results show that these two low complexity detectors still have superior recovery performance than the conventional MUD methods, and nearly have the same performance compared with SBL and PCSBL.
更多查看译文
关键词
Multiuser detection,Bayes methods,Uplink,Approximation algorithms,Computational complexity,Message passing,NOMA,GF-NOMA,multiuser detection,GAMP-SBL,GAMP-PCSBL
AI 理解论文
溯源树
样例
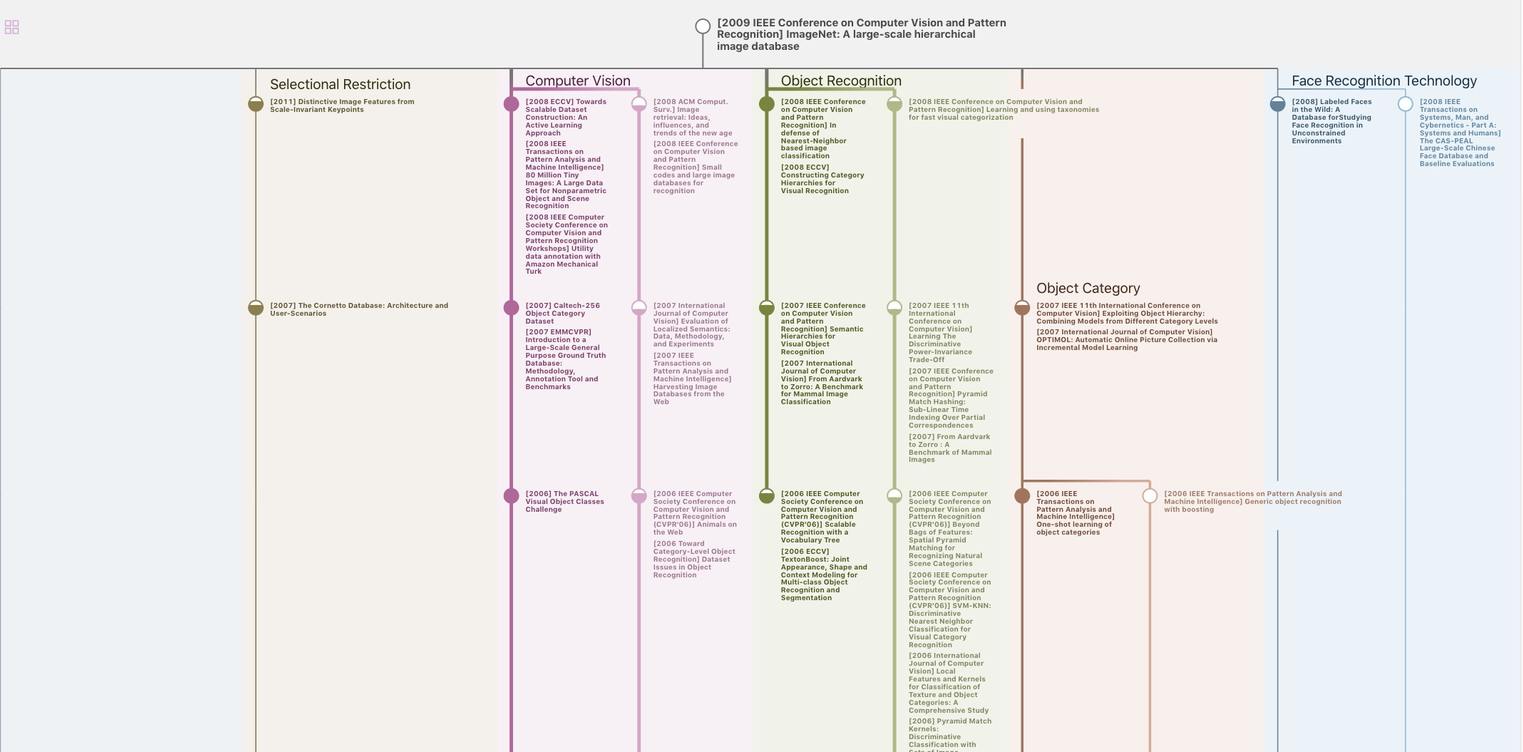
生成溯源树,研究论文发展脉络
Chat Paper
正在生成论文摘要