Computed tomography and guidelines-based human-machine fusion model for predicting resectability of the pancreatic cancer
JOURNAL OF GASTROENTEROLOGY AND HEPATOLOGY(2024)
摘要
Background and AimThe study aims to develop a hybrid machine learning model for predicting resectability of the pancreatic cancer, which is based on computed tomography (CT) and National Comprehensive Cancer Network (NCCN) guidelines.MethodWe retrospectively studied 349 patients. One hundred seventy-one cases from Center 1 and 92 cases from Center 2 were used as the primary training cohort, and 66 cases from Center 3 and 20 cases from Center 4 were used as the independent test dataset.Semi-automatic module of ITK-SNAP software was used to assist CT image segmentation to obtain three-dimensional (3D) imaging region of interest (ROI). There were 788 handcrafted features extracted for 3D ROI using PyRadiomics. The optimal feature subset consists of three features screened by three feature selection methods as the input of the SVM to construct the conventional radiomics-based predictive model (cRad). 3D ROI was used to unify the resolution by 3D spline interpolation method for constructing the 3D tumor imaging tensor. Using 3D tumor image tensor as input, 3D kernelled support tensor machine-based predictive model (KSTM), and 3D ResNet-based deep learning predictive model (ResNet) were constructed. Multi-classifier fusion ML model is constructed by fusing cRad, KSTM, and ResNet using multi-classifier fusion strategy. Two experts with more than 10 years of clinical experience were invited to reevaluate each patient based on their CECT following the NCCN guidelines to obtain resectable, unresectable, and borderline resectable diagnoses. The three results were converted into probability values of 0.25, 0.75, and 0.50, respectively, according to the traditional empirical method. Then it is used as an independent classifier and integrated with multi-classifier fusion machine learning (ML) model to obtain the human-machine fusion ML model (HMfML).MethodWe retrospectively studied 349 patients. One hundred seventy-one cases from Center 1 and 92 cases from Center 2 were used as the primary training cohort, and 66 cases from Center 3 and 20 cases from Center 4 were used as the independent test dataset.Semi-automatic module of ITK-SNAP software was used to assist CT image segmentation to obtain three-dimensional (3D) imaging region of interest (ROI). There were 788 handcrafted features extracted for 3D ROI using PyRadiomics. The optimal feature subset consists of three features screened by three feature selection methods as the input of the SVM to construct the conventional radiomics-based predictive model (cRad). 3D ROI was used to unify the resolution by 3D spline interpolation method for constructing the 3D tumor imaging tensor. Using 3D tumor image tensor as input, 3D kernelled support tensor machine-based predictive model (KSTM), and 3D ResNet-based deep learning predictive model (ResNet) were constructed. Multi-classifier fusion ML model is constructed by fusing cRad, KSTM, and ResNet using multi-classifier fusion strategy. Two experts with more than 10 years of clinical experience were invited to reevaluate each patient based on their CECT following the NCCN guidelines to obtain resectable, unresectable, and borderline resectable diagnoses. The three results were converted into probability values of 0.25, 0.75, and 0.50, respectively, according to the traditional empirical method. Then it is used as an independent classifier and integrated with multi-classifier fusion machine learning (ML) model to obtain the human-machine fusion ML model (HMfML). ResultsMulti-classifier fusion ML model's area under receiver operating characteristic curve (AUC; 0.8610), predictive accuracy (ACC: 80.23%), sensitivity (SEN: 78.95%), and specificity (SPE: 80.60%) is better than cRad, KSTM, and ResNet-based single-classifier models and their two-classifier fusion models. This means that three different models have mined complementary CECT feature expression from different perspectives and can be integrated through CFS-ER, so that the fusion model has better performance. HMfML's AUC (0.8845), ACC (82.56%), SEN (84.21%), SPE (82.09%). This means that ML models might learn extra information from CECT that experts cannot distinguish, thus complementing expert experience and improving the performance of hybrid ML models.ConclusionHMfML can predict PC resectability with high accuracy. 1image
更多查看译文
关键词
CECT,CFS-ER,HMfML,Machine learning,Pancreatic cancer,PDAC
AI 理解论文
溯源树
样例
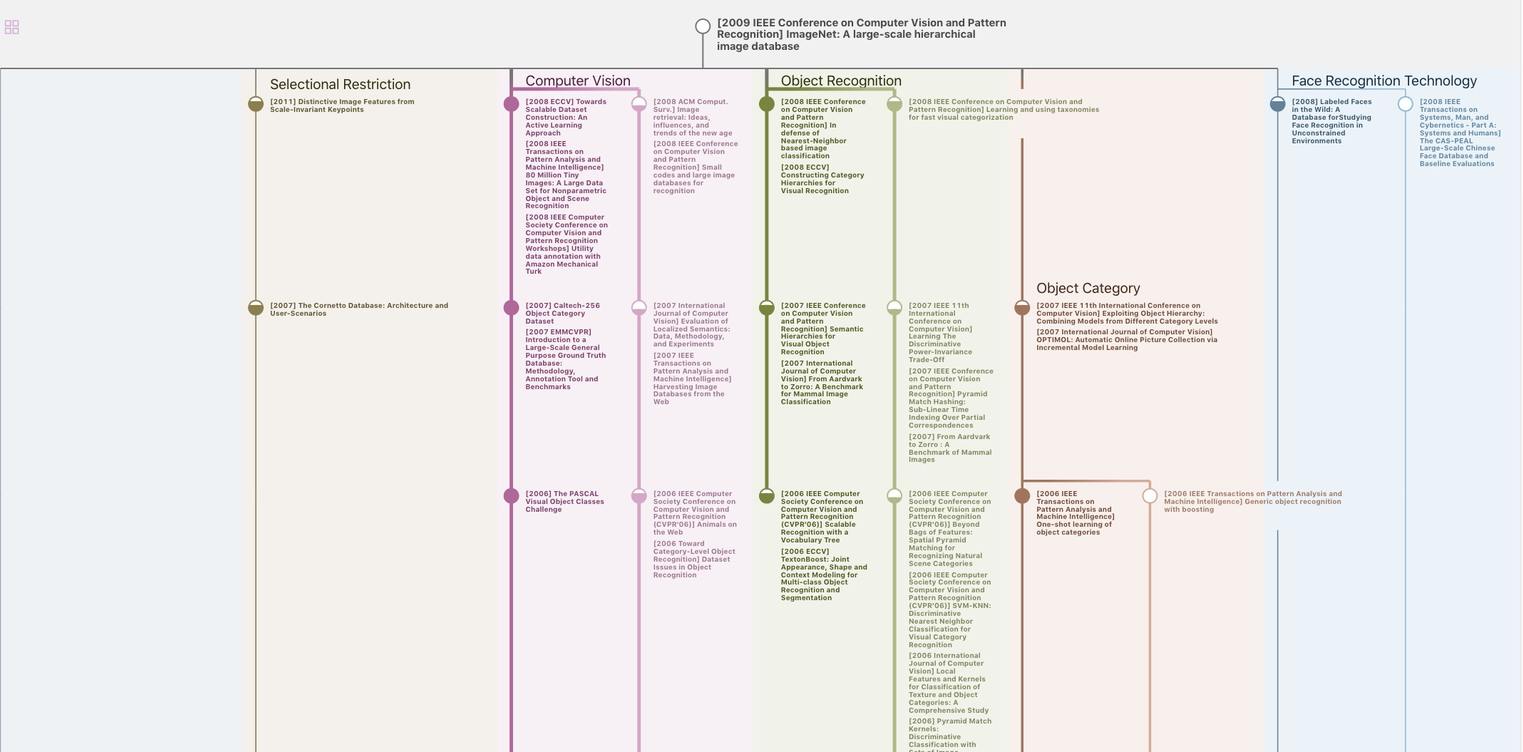
生成溯源树,研究论文发展脉络
Chat Paper
正在生成论文摘要