Identifying relapse predictors in individual participant data with decision trees
BMC Psychiatry(2023)
摘要
Depression is a highly common and recurrent condition. Predicting who is at most risk of relapse or recurrence can inform clinical practice. Applying machine-learning methods to Individual Participant Data (IPD) can be promising to improve the accuracy of risk predictions. Individual data of four Randomized Controlled Trials (RCTs) evaluating antidepressant treatment compared to psychological interventions with tapering ( N=714 ) were used to identify predictors of relapse and/or recurrence. Ten baseline predictors were assessed. Decision trees with and without gradient boosting were applied. To study the robustness of decision-tree classifications, we also performed a complementary logistic regression analysis. The combination of age, age of onset of depression, and depression severity significantly enhances the prediction of relapse risk when compared to classifiers solely based on depression severity. The studied decision trees can (i) identify relapse patients at intake with an accuracy, specificity, and sensitivity of about 55
更多查看译文
关键词
relapse predictors,decision trees,individual participant data
AI 理解论文
溯源树
样例
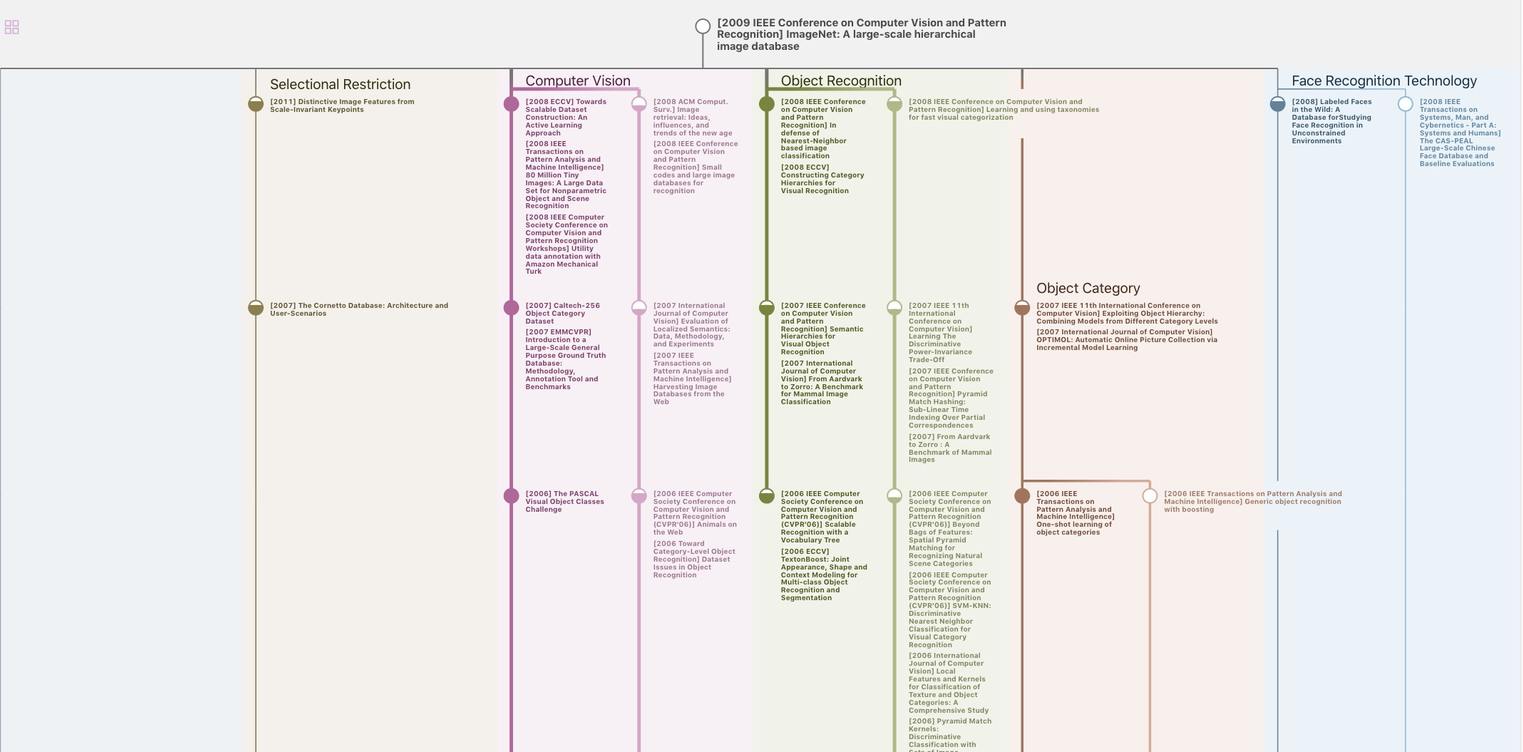
生成溯源树,研究论文发展脉络
Chat Paper
正在生成论文摘要