Task-adaptive physical reservoir computing
Nature Materials(2024)
摘要
Reservoir computing is a neuromorphic architecture that may offer viable solutions to the growing energy costs of machine learning. In software-based machine learning, computing performance can be readily reconfigured to suit different computational tasks by tuning hyperparameters. This critical functionality is missing in ‘physical’ reservoir computing schemes that exploit nonlinear and history-dependent responses of physical systems for data processing. Here we overcome this issue with a ‘task-adaptive’ approach to physical reservoir computing. By leveraging a thermodynamical phase space to reconfigure key reservoir properties, we optimize computational performance across a diverse task set. We use the spin-wave spectra of the chiral magnet Cu 2 OSeO 3 that hosts skyrmion, conical and helical magnetic phases, providing on-demand access to different computational reservoir responses. The task-adaptive approach is applicable to a wide variety of physical systems, which we show in other chiral magnets via above (and near) room-temperature demonstrations in Co 8.5 Zn 8.5 Mn 3 (and FeGe).
更多查看译文
关键词
task-adaptive
AI 理解论文
溯源树
样例
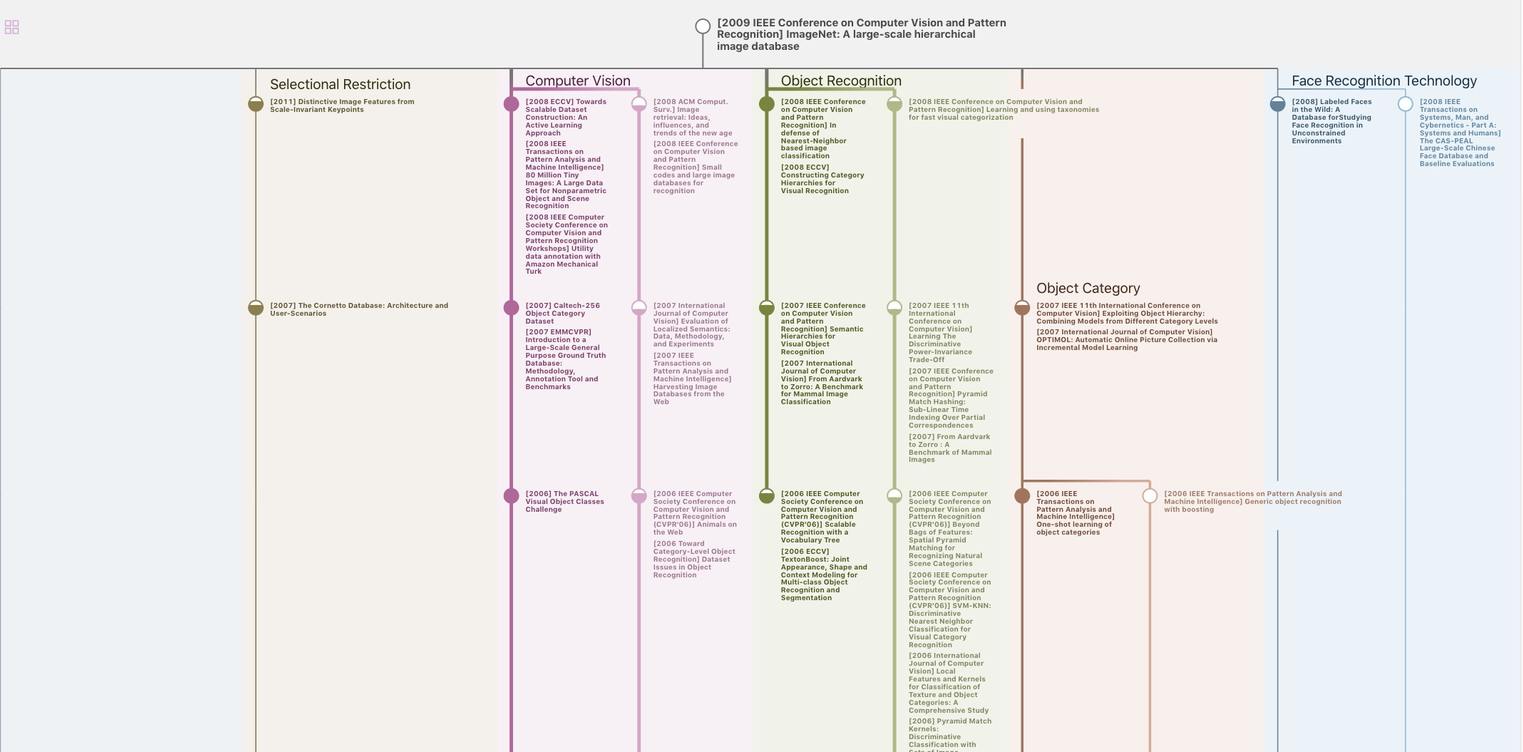
生成溯源树,研究论文发展脉络
Chat Paper
正在生成论文摘要