Transformers can optimally learn regression mixture models
ICLR 2024(2023)
摘要
Mixture models arise in many regression problems, but most methods have seen
limited adoption partly due to these algorithms' highly-tailored and
model-specific nature. On the other hand, transformers are flexible, neural
sequence models that present the intriguing possibility of providing
general-purpose prediction methods, even in this mixture setting. In this work,
we investigate the hypothesis that transformers can learn an optimal predictor
for mixtures of regressions. We construct a generative process for a mixture of
linear regressions for which the decision-theoretic optimal procedure is given
by data-driven exponential weights on a finite set of parameters. We observe
that transformers achieve low mean-squared error on data generated via this
process. By probing the transformer's output at inference time, we also show
that transformers typically make predictions that are close to the optimal
predictor. Our experiments also demonstrate that transformers can learn
mixtures of regressions in a sample-efficient fashion and are somewhat robust
to distribution shifts. We complement our experimental observations by proving
constructively that the decision-theoretic optimal procedure is indeed
implementable by a transformer.
更多查看译文
关键词
transformers,mixture models,linear regression
AI 理解论文
溯源树
样例
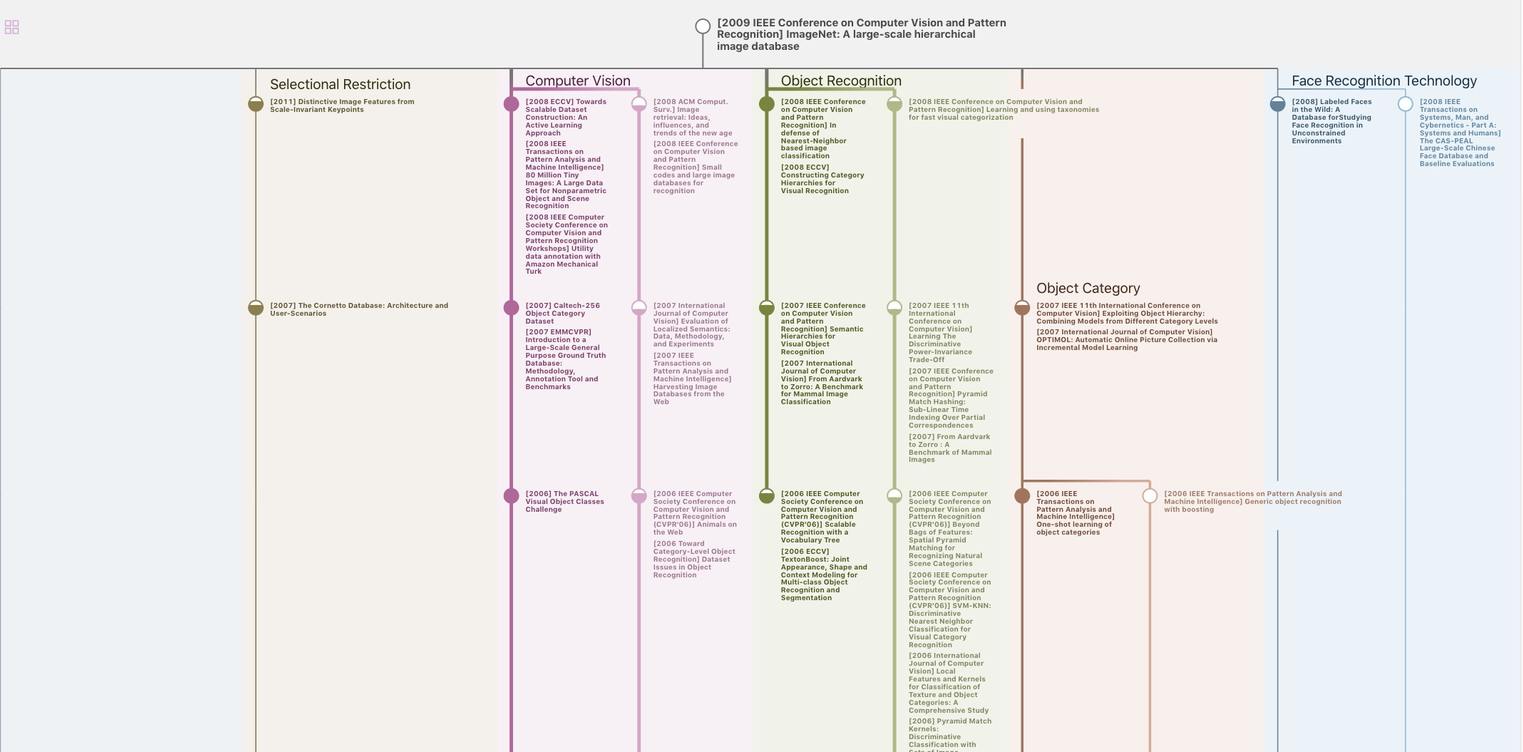
生成溯源树,研究论文发展脉络
Chat Paper
正在生成论文摘要