Red Giants Search Method Based on Convolutional Neural Networks
The Astronomical Journal(2023)
摘要
Red giants play a crucial role in astronomical exploration. However, the current search for these stars heavily relies on spectrum analysis, making it a cumbersome process. In this paper, we propose a novel red giant search approach called CBAMResNets. CBAMResNets enhances feature extraction from images by incorporating the Convolutional Block Attention Module into the ResNets model. To train and validate our model, we utilize accurately labeled stellar image data obtained by combining the Sky Mapper Southern Survey (SMSS) and the Large Sky Area Multi-Object Fiber Spectroscopic Telescope. Our experiments demonstrate that CBAMResNets outperforms other networks such as VGG16 and TPC across most performance metrics and remains competitive with traditional machine-learning methods. Specifically, for stars within the magnitude range of 11-19 in the u band, our model achieves a precision rate of 0.92 and a recall rate of 0.9194. Similarly, for stars within the magnitude range of 11-15 in the u band, the precision rate is 0.92, and the recall rate is 0.9813. Furthermore, we apply CBAMResNets to the SMSS subregion, resulting in the identification of 20,243 potential red giant candidates out of the 304,477 observed stars. We validate the authenticity of these candidates by analyzing their stellar absolute magnitudes and temperatures, estimating a contamination rate of approximately 6.4%. Additionally, we examine the approximate distribution of their metallicity. The catalog containing the identified red giant candidates can be accessed at Zenodo. 3 3 doi:10.5281/zenodo.8352420
更多查看译文
关键词
convolutional neural networks,search,red
AI 理解论文
溯源树
样例
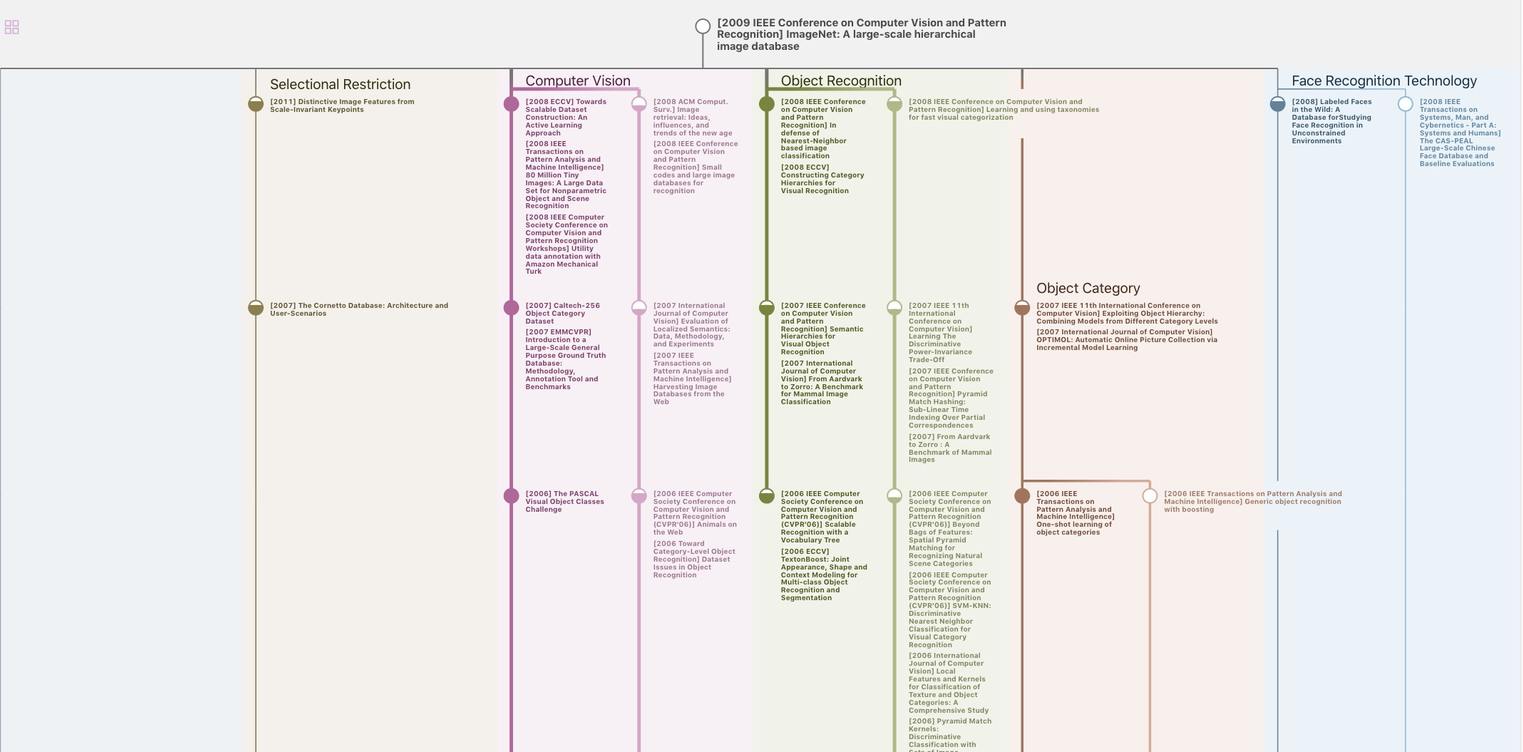
生成溯源树,研究论文发展脉络
Chat Paper
正在生成论文摘要