Teaching a Robot Where to Park: A Scalable Crowdsourcing Approach
2023 32ND IEEE INTERNATIONAL CONFERENCE ON ROBOT AND HUMAN INTERACTIVE COMMUNICATION, RO-MAN(2023)
摘要
For social robots to successfully integrate into daily life in home environments, they will need reliable models of the way people perceive and use space in the home. This paper explores the problem of obtaining annotated training data at scale for subjective judgments about spatial locations. Focusing on the use case of identifying good and bad parking spots for a social robot operating in a home environment, two experiments are presented. The first study shows that the presentation of context-rich 3D images to human annotators yields notably different outcomes from those obtained when using 2D robot navigation maps. We attribute the source of these differences to a set of features visible only in the 3D views and introduce a technique for labeling these features on the 2D maps. The second study reveals that using labeled 2D maps produces annotation data very similar to that obtained using 3D images. Since a labeled 2D map can be generated at a fraction of the cost of a full set of 3D views, we recommend this method as a scalable approach to collecting subjective spatial data annotations in everyday environments.
更多查看译文
AI 理解论文
溯源树
样例
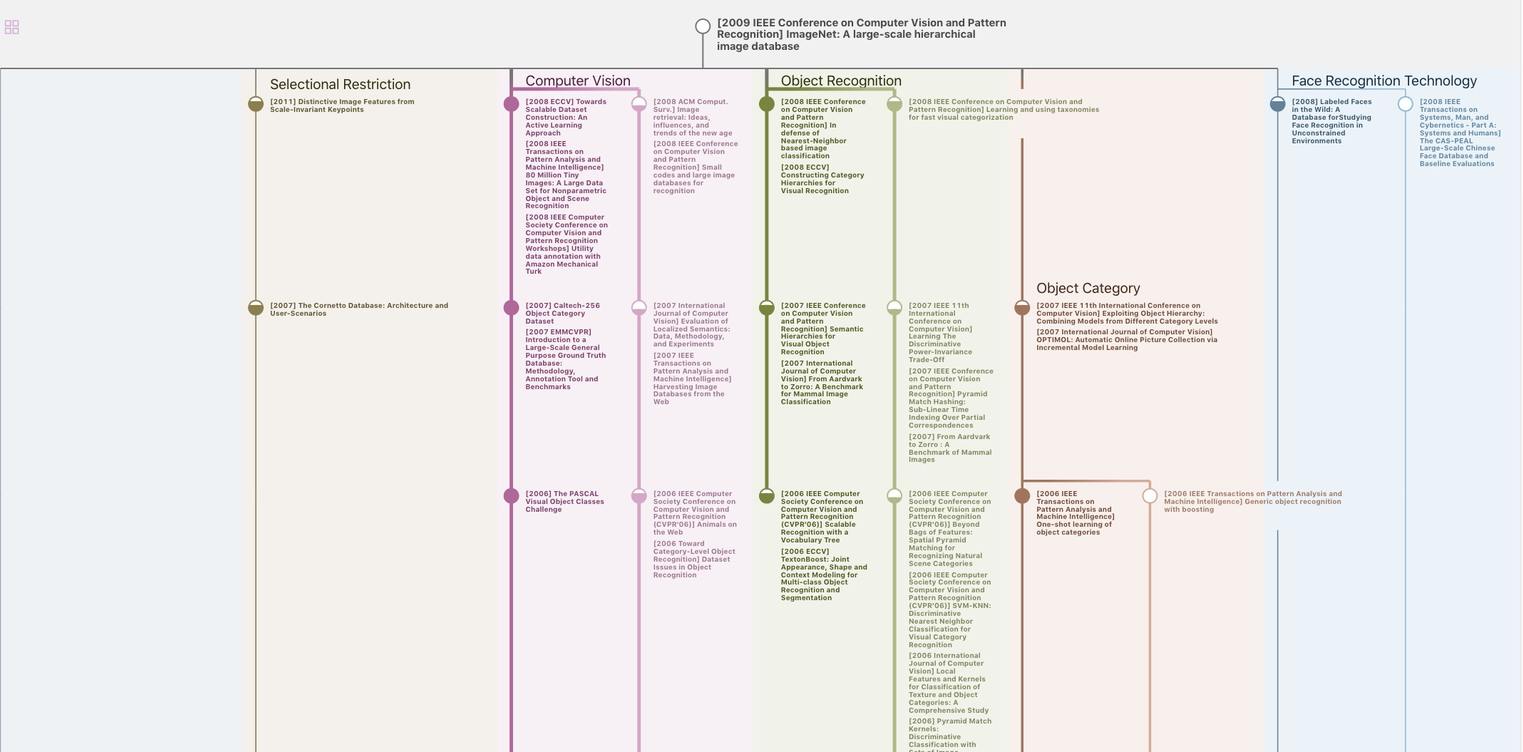
生成溯源树,研究论文发展脉络
Chat Paper
正在生成论文摘要