Extractive Conversation Summarization Driven by Textual Entailment Prediction
2023 IEEE 17th International Conference on Application of Information and Communication Technologies (AICT)(2023)
摘要
Summarizing conversations like meetings, email threads or discussion forums poses relevant challenges on how to model the dialogue structure. Existing approaches mainly focus on premise-claim entailment relationships while neglecting contrasting or uncertain assertions. Furthermore, existing techniques are abstractive, thus requiring a training set consisting of humanly generated summaries. With the twofold aim of enriching the dialogue representation and addressing conversation summarization in the absence of training data, we present an extractive conversation summarization pipeline. We explore the use of contradictions and neutral premise-claim relations, both in the same document or in different documents. The results achieved on four datasets covering different domains show that applying unsupervised methods on top of a refined premise-claim selection achieves competitive performance in most domains.
更多查看译文
关键词
Conversation Summarization,Extractive Summarization,Textual Entailment,Conversational AI
AI 理解论文
溯源树
样例
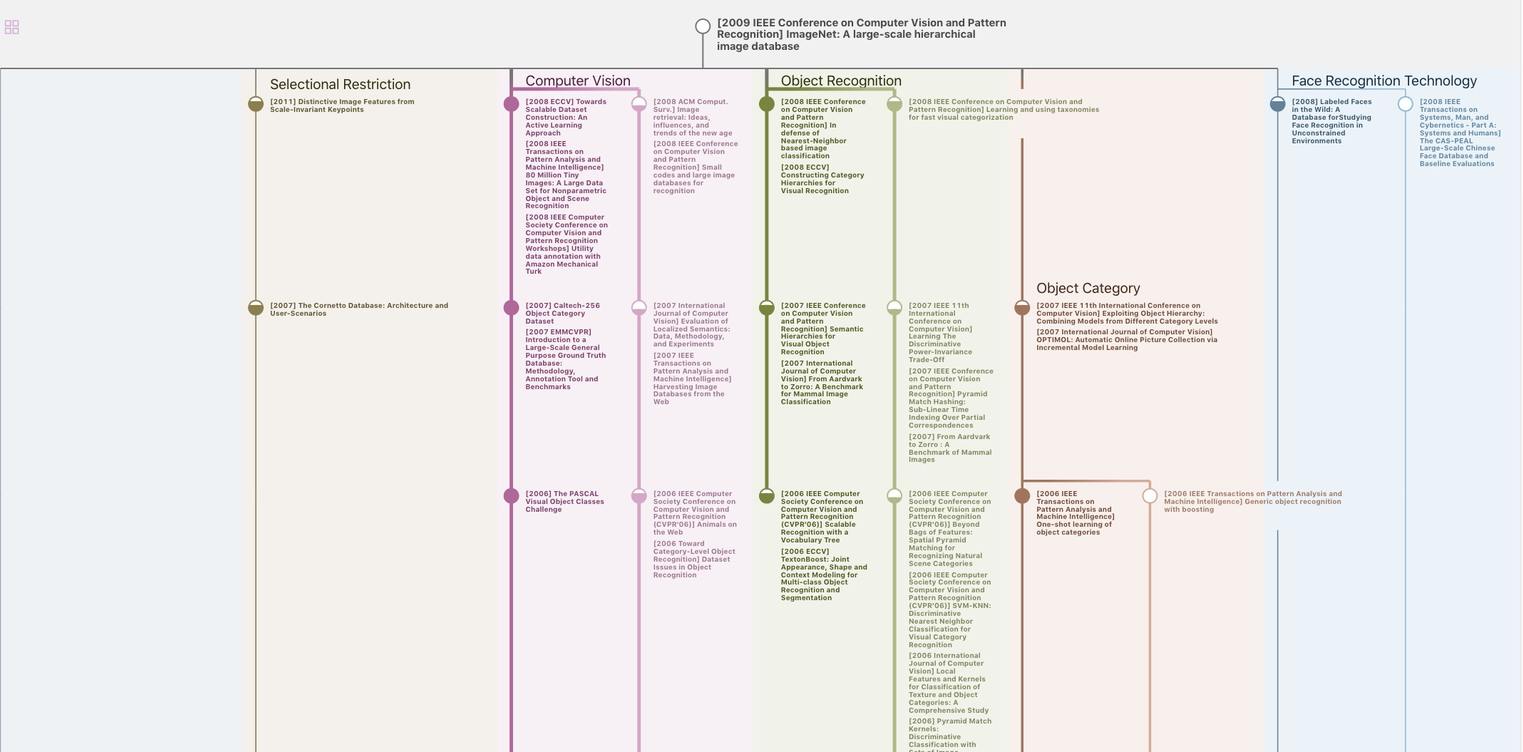
生成溯源树,研究论文发展脉络
Chat Paper
正在生成论文摘要