On the Predictive Power of Objective Intelligibility Metrics for the Subjective Performance of Deep Complex Convolutional Recurrent Speech Enhancement Networks
IEEE-ACM TRANSACTIONS ON AUDIO SPEECH AND LANGUAGE PROCESSING(2024)
摘要
Speech enhancement (SE) systems aim to improve the quality and intelligibility of degraded speech signals obtained from far-field microphones. Subjective evaluation of the intelligibility performance of these SE systems is uncommon. Instead, objective intelligibility measures (OIMs) are generally used to predict subjective performance increases. Many recent deep learning (DL) based SE systems, are expected to improve the intelligibility of degraded speech as measured by OIMs. However, validation of the ability of these OIMs to predict subjective intelligibility when enhancing a speech signal using DL-based systems is lacking. Therefore, in this study, we evaluate the predictive performance of five popular OIMs. We compare the metrics' predictions with subjective results. For this purpose, we recruited 50 human listeners, and subjectively tested both single channel and multi-channel Deep Complex Convolutional Recurrent Network (DCCRN) based speech enhancement systems. We found that none of the OIMs gave reliable predictions, and that all OIMs overestimated the intelligibility of 'enhanced' speech signals.
更多查看译文
关键词
Intelligibility,objective metrics,speech enhancement,subjective evaluation
AI 理解论文
溯源树
样例
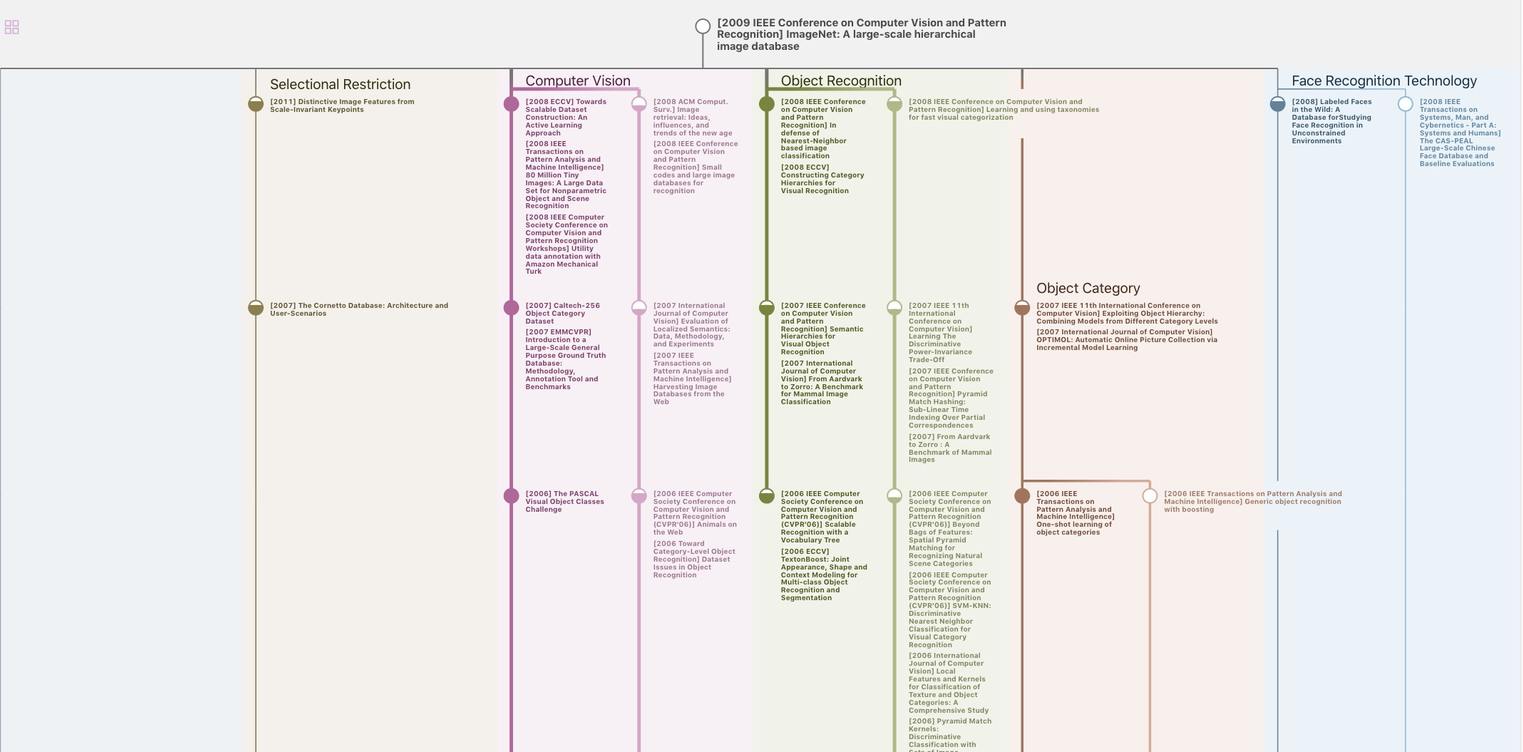
生成溯源树,研究论文发展脉络
Chat Paper
正在生成论文摘要