Learning with privileged and sensitive information: a gradient-boosting approach.
Frontiers in artificial intelligence(2023)
摘要
We consider the problem of learning with sensitive features under the privileged information setting where the goal is to learn a classifier that uses features not available (or too sensitive to collect) at test/deployment time to learn a better model at training time. We focus on tree-based learners, specifically gradient-boosted decision trees for learning with privileged information. Our methods use privileged features as knowledge to guide the algorithm when learning from fully observed (usable) features. We derive the theory, empirically validate the effectiveness of our algorithms, and verify them on standard fairness metrics.
更多查看译文
关键词
sensitive information,learning,gradient-boosting
AI 理解论文
溯源树
样例
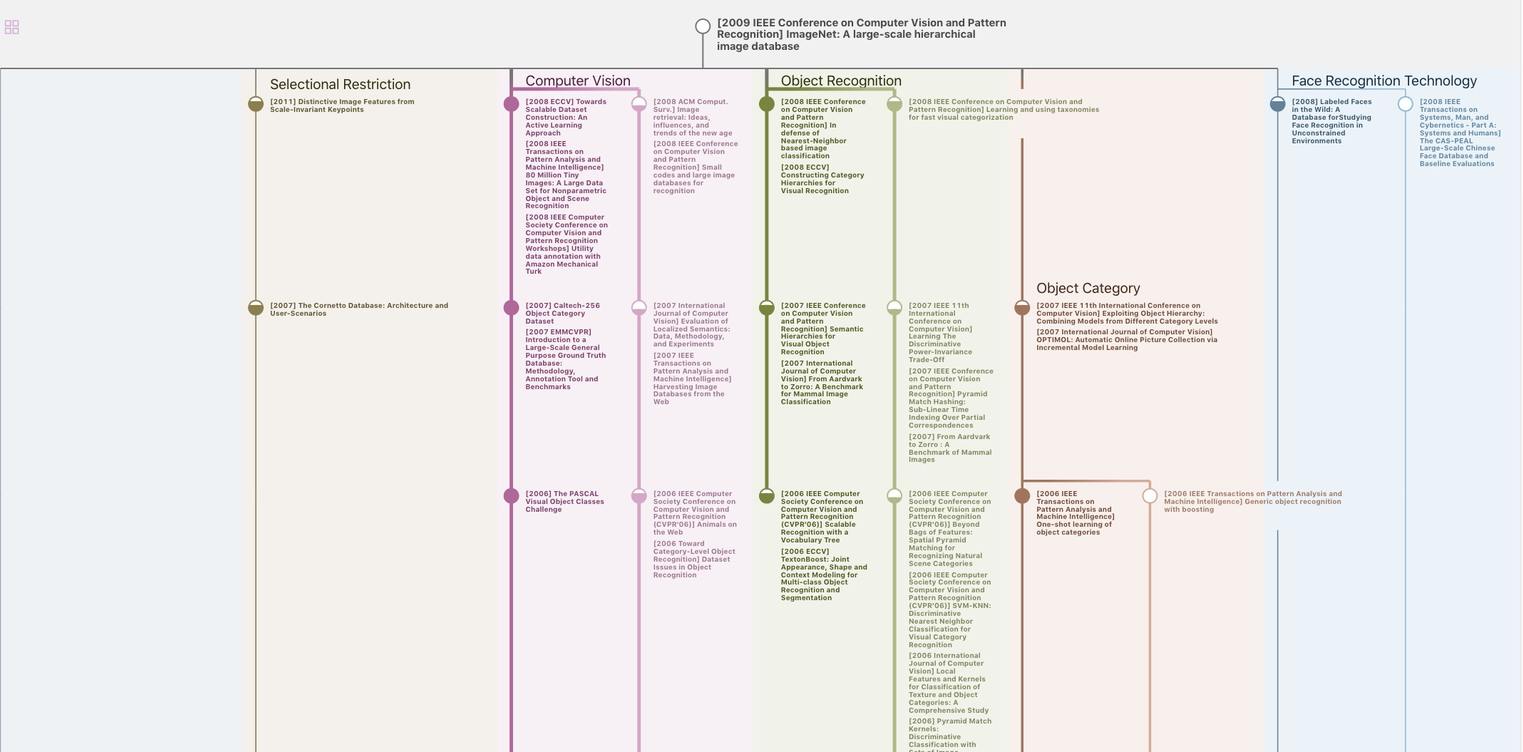
生成溯源树,研究论文发展脉络
Chat Paper
正在生成论文摘要