ADAMM: Anomaly Detection of Attributed Multi-graphs with Metadata: A Unified Neural Network Approach.
CoRR(2023)
摘要
Given a complex graph database of node- and edge-attributed multi-graphs as well as associated metadata for each graph, how can we spot the anomalous instances? Many real-world problems can be cast as graph inference tasks where the graph representation could capture complex relational phenomena (e.g., transactions among financial accounts in a journal entry), along with metadata reflecting tabular features (e.g. approver, effective date, etc.). While numerous anomaly detectors based on Graph Neural Networks (GNNs) have been proposed, none are capable of directly handling directed graphs with multi-edges and self-loops. Furthermore, the simultaneous handling of relational and tabular features remains an unexplored area. In this work we propose ADAMM, a novel graph neural network model that handles directed multi-graphs, providing a unified end-to-end architecture that fuses metadata and graph-level representation learning through an unsupervised anomaly detection objective. Experiments on datasets from two different domains, namely, general-ledger journal entries from different firms (accounting) as well as human GPS trajectories from thousands of individuals (urban mobility) validate ADAMM's generality and detection effectiveness of expert-guided and ground-truth anomalies. Notably, ADAMM outperforms existing baselines that handle the two data modalities (graph and metadata) separately with post hoc synthesis efforts.
更多查看译文
关键词
anomaly detection,complex graphs,graph neural networks,multi-edges,node and edge attributes,metadata
AI 理解论文
溯源树
样例
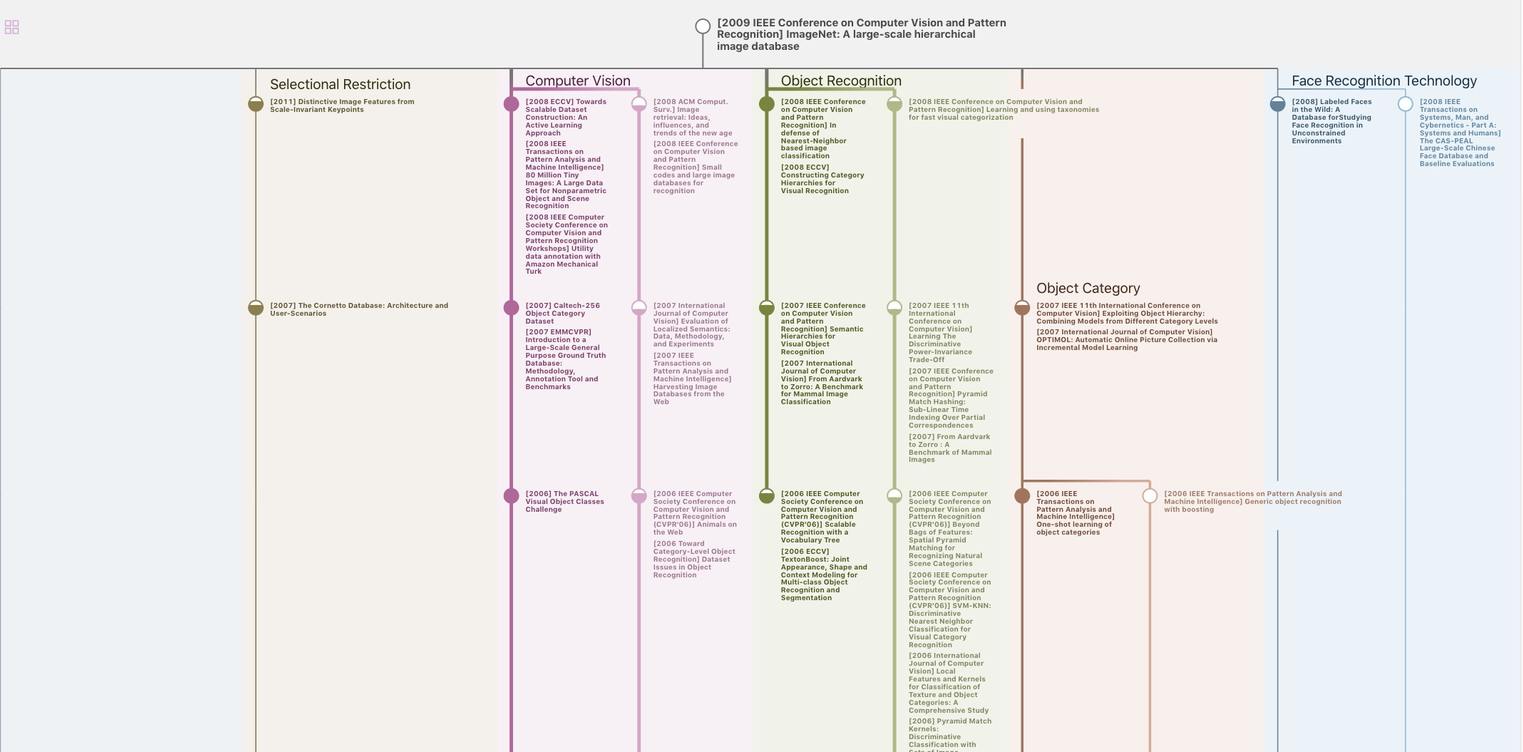
生成溯源树,研究论文发展脉络
Chat Paper
正在生成论文摘要