Privacy Risks Analysis and Mitigation in Federated Learning for Medical Images
CoRR(2023)
摘要
Federated learning (FL) is gaining increasing popularity in the medical
domain for analyzing medical images, which is considered an effective technique
to safeguard sensitive patient data and comply with privacy regulations.
However, several recent studies have revealed that the default settings of FL
may leak private training data under privacy attacks. Thus, it is still unclear
whether and to what extent such privacy risks of FL exist in the medical
domain, and if so, "how to mitigate such risks?". In this paper, first, we
propose a holistic framework for Medical data Privacy risk analysis and
mitigation in Federated Learning (MedPFL) to analyze privacy risks and develop
effective mitigation strategies in FL for protecting private medical data.
Second, we demonstrate the substantial privacy risks of using FL to process
medical images, where adversaries can easily perform privacy attacks to
reconstruct private medical images accurately. Third, we show that the defense
approach of adding random noises may not always work effectively to protect
medical images against privacy attacks in FL, which poses unique and pressing
challenges associated with medical data for privacy protection.
更多查看译文
关键词
Federated Learning,Gradient Leakage Attack,Medical Image Analysis,Privacy Risk
AI 理解论文
溯源树
样例
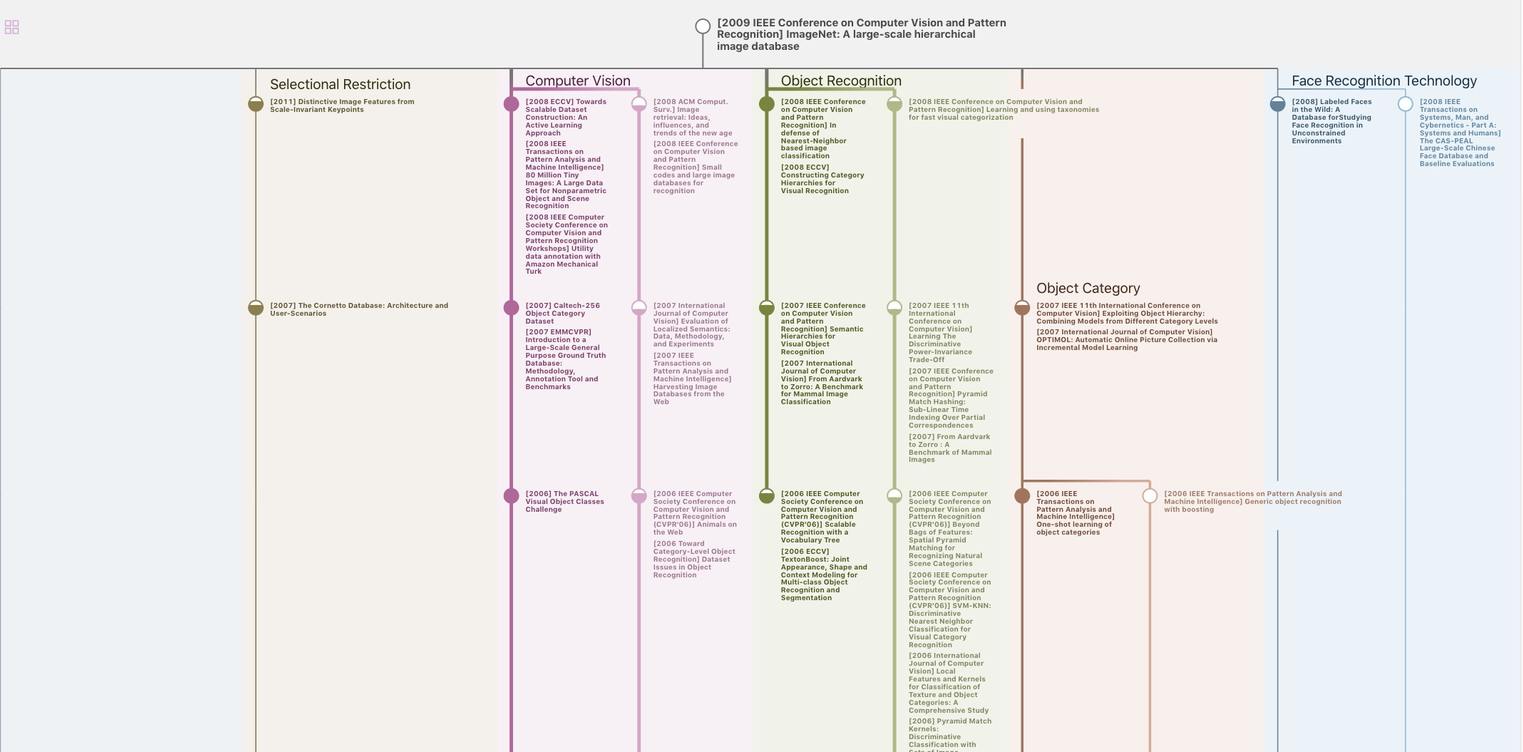
生成溯源树,研究论文发展脉络
Chat Paper
正在生成论文摘要