Understanding Grokking Through A Robustness Viewpoint.
CoRR(2023)
摘要
Recently, an unusual phenomenon called grokking has gained much attention, where sometimes a neural network generalizes long after it perfectly fits the training data. We try to understand this seemingly strange phenomenon using the robustness of the neural network. Using a robustness viewpoint, we show that the popular $l_2$ weight norm (metric) of the neural network is actually a sufficient condition for grokking. As we also empirically find that $l_2$ norm correlates with grokking on the test data not in a timely way, we propose new metrics based on robustness and information theory and find that our new metrics correlate well with the grokking phenomenon. Based on the previous observations, we propose methods to speed up the generalization process. In addition, we examine the standard training process on modulo addition dataset and find that it hardly learns other basic group operations before grokking, including the commutative law. Interestingly, the speed up of generalization when using our proposed method can be partially explained by learning the commutative law, a necessary condition when the model groks on test dataset.
更多查看译文
AI 理解论文
溯源树
样例
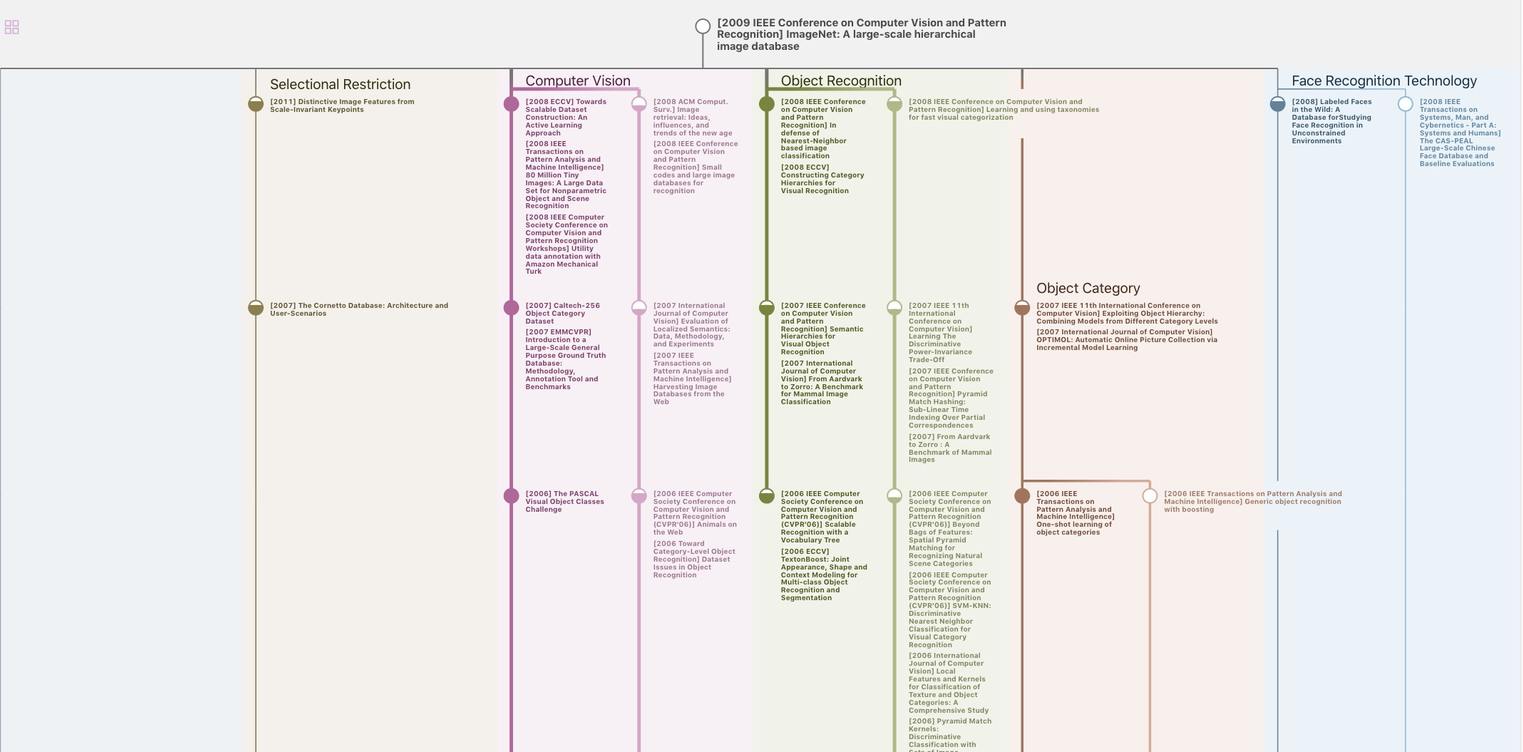
生成溯源树,研究论文发展脉络
Chat Paper
正在生成论文摘要