OR Residual Connection Achieving Comparable Accuracy to ADD Residual Connection in Deep Residual Spiking Neural Networks.
CoRR(2023)
摘要
Spiking Neural Networks (SNNs) have garnered substantial attention in brain-like computing for their biological fidelity and the capacity to execute energy-efficient spike-driven operations. As the demand for heightened performance in SNNs surges, the trend towards training deeper networks becomes imperative, while residual learning stands as a pivotal method for training deep neural networks. In our investigation, we identified that the SEW-ResNet, a prominent representative of deep residual spiking neural networks, incorporates non-event-driven operations. To rectify this, we introduce the OR Residual connection (ORRC) to the architecture. Additionally, we propose the Synergistic Attention (SynA) module, an amalgamation of the Inhibitory Attention (IA) module and the Multi-dimensional Attention (MA) module, to offset energy loss stemming from high quantization. When integrating SynA into the network, we observed the phenomenon of "natural pruning", where after training, some or all of the shortcuts in the network naturally drop out without affecting the model's classification accuracy. This significantly reduces computational overhead and makes it more suitable for deployment on edge devices. Experimental results on various public datasets confirmed that the SynA enhanced OR-Spiking ResNet achieved single-sample classification with as little as 0.8 spikes per neuron. Moreover, when compared to other spike residual models, it exhibited higher accuracy and lower power consumption. Codes are available at https://github.com/Ym-Shan/ORRC-SynA-natural-pruning.
更多查看译文
AI 理解论文
溯源树
样例
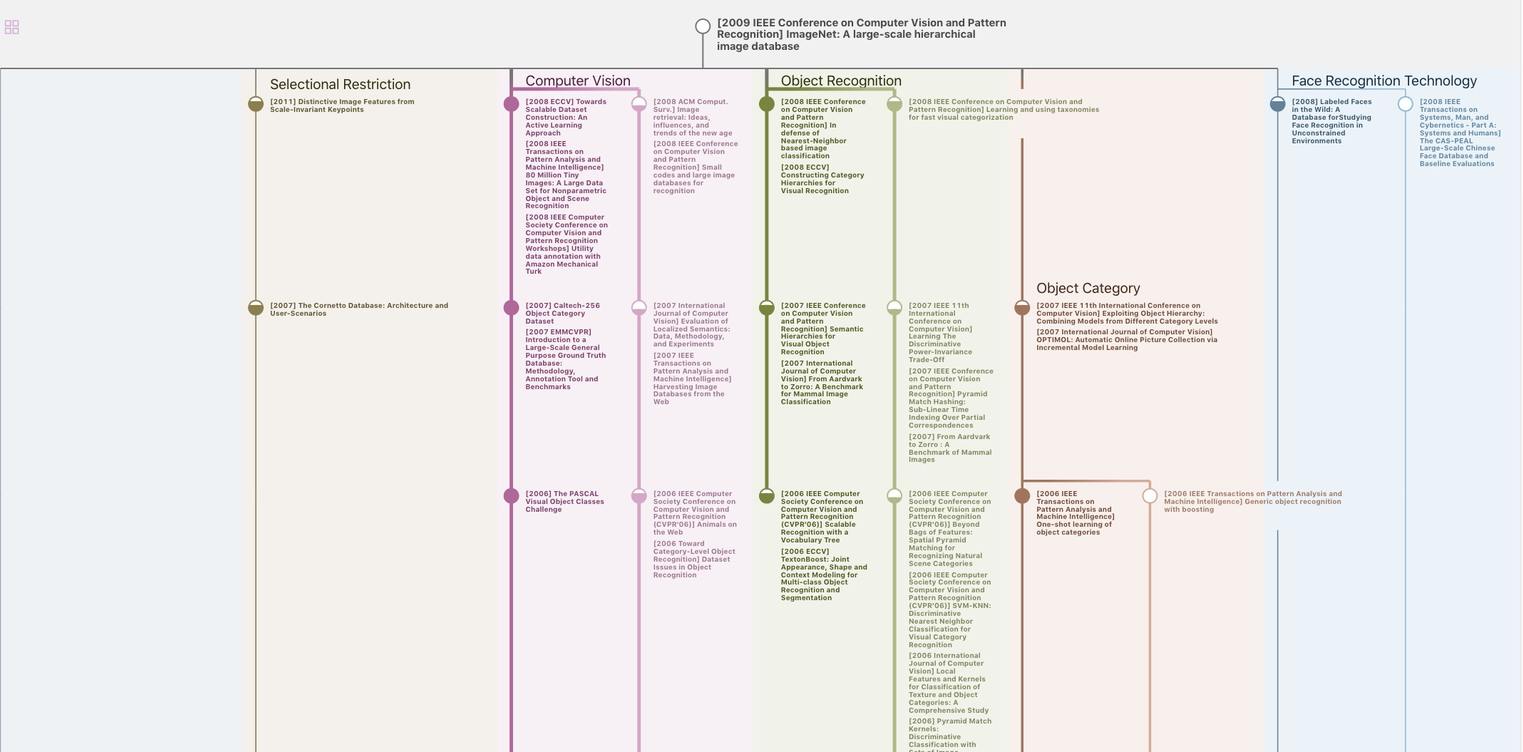
生成溯源树,研究论文发展脉络
Chat Paper
正在生成论文摘要