MaSk-LMM: A Matrix Sketching Framework for Linear Mixed Models in Association Studies
medRxiv (Cold Spring Harbor Laboratory)(2023)
摘要
Linear mixed models (LMMs) have been widely used in genome-wide association studies (GWAS) to control for population stratification and cryptic relatedness. Unfortunately, estimating LMM parameters is computationally expensive, necessitating large-scale matrix operations to build the genetic relatedness matrix (GRM). Over the past 25 years, Randomized Linear Algebra has provided alternative approaches to such matrix operations by leveraging matrix sketching, which often results in provably accurate fast and efficient approximations. We leverage matrix sketching to develop a fast and efficient LMM method called Matrix-Sketching LMM (MaSk-LMM) by sketching the genotype matrix to reduce its dimensions and speed up computations. Our framework comes with both theoretical guarantees and a strong empirical performance compared to current state-of-the-art.
### Competing Interest Statement
The authors have declared no competing interest.
### Funding Statement
PD and MB were partially supported by NSF 10001674, NSF 10001225, an IBM Faculty Award to PD, and an NSF GRFP to MB. AB and LP were supported by IBM Research.
### Author Declarations
I confirm all relevant ethical guidelines have been followed, and any necessary IRB and/or ethics committee approvals have been obtained.
Yes
The details of the IRB/oversight body that provided approval or exemption for the research described are given below:
Data analysis was performed under UK Biobank application 50658 using existing publicly available and deidentified data and was IRB exempt. I confirm that all necessary patient/participant consent has been obtained and the appropriate institutional forms have been archived, and that any patient/participant/sample identifiers included were not known to anyone (e.g., hospital staff, patients or participants themselves) outside the research group so cannot be used to identify individuals.
I confirm that all necessary patient/participant consent has been obtained and the appropriate institutional forms have been archived, and that any patient/participant/sample identifiers included were not known to anyone (e.g., hospital staff, patients or participants themselves) outside the research group so cannot be used to identify individuals.
Yes
I understand that all clinical trials and any other prospective interventional studies must be registered with an ICMJE-approved registry, such as ClinicalTrials.gov. I confirm that any such study reported in the manuscript has been registered and the trial registration ID is provided (note: if posting a prospective study registered retrospectively, please provide a statement in the trial ID field explaining why the study was not registered in advance).
Yes
I have followed all appropriate research reporting guidelines, such as any relevant EQUATOR Network research reporting checklist(s) and other pertinent material, if applicable.
Yes
Data analysis was performed under UK Biobank application 50658 using existing publicly available and deidentified data and was IRB exempt.
更多查看译文
关键词
association studies,linear mixed models,matrix sketching framework,mask-lmm
AI 理解论文
溯源树
样例
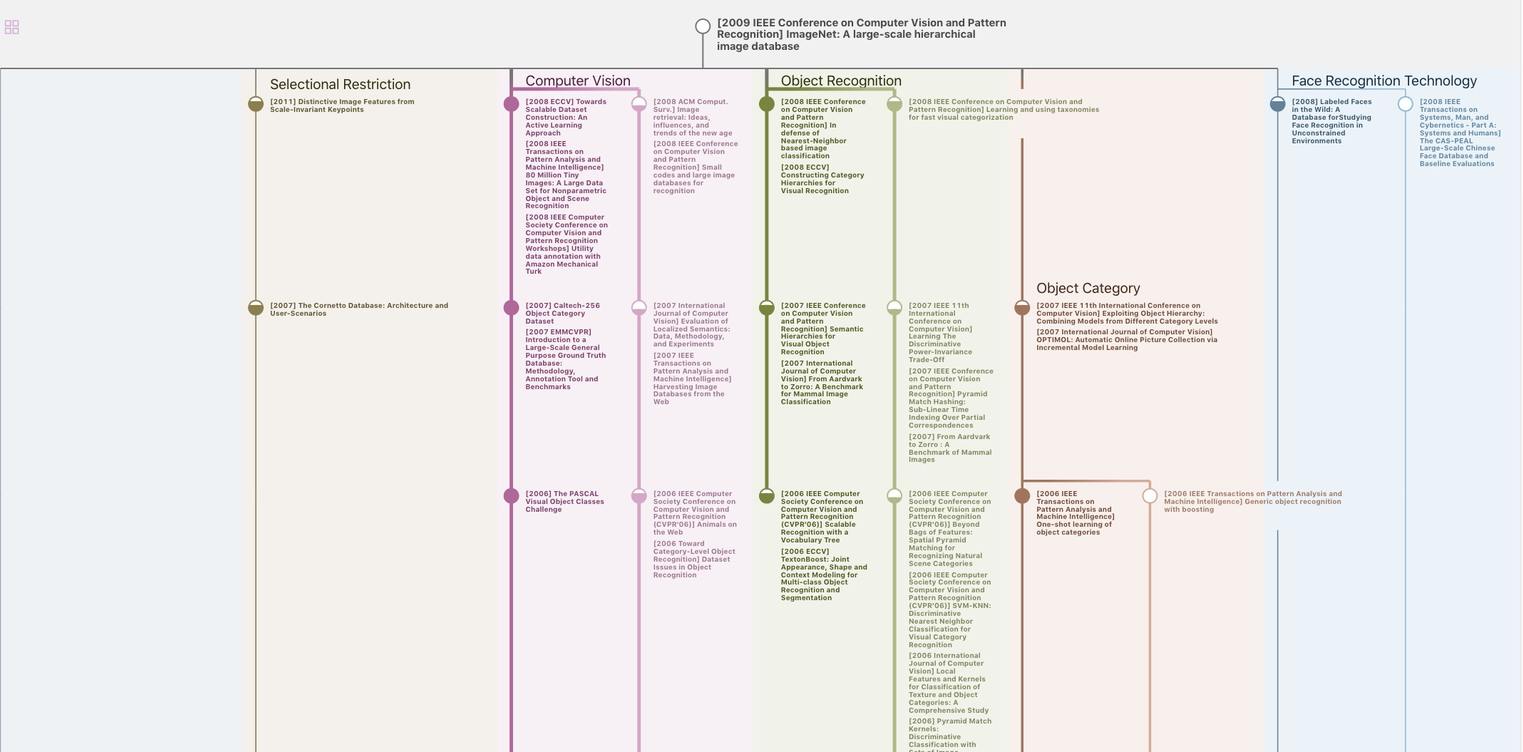
生成溯源树,研究论文发展脉络
Chat Paper
正在生成论文摘要