STCA-SNN: self-attention-based temporal-channel joint attention for spiking neural networks
Frontiers in Neuroscience(2023)
摘要
Spiking Neural Networks (SNNs) have shown great promise in processing spatio-temporal information compared to Artificial Neural Networks (ANNs). However, there remains a performance gap between SNNs and ANNs, which impedes the practical application of SNNs. With intrinsic event-triggered property and temporal dynamics, SNNs have the potential to effectively extract spatio-temporal features from event streams. To leverage the temporal potential of SNNs, we propose a self-attention-based temporal-channel joint attention SNN (STCA-SNN) with end-to-end training, which infers attention weights along both temporal and channel dimensions concurrently. It models global temporal and channel information correlations with self-attention, enabling the network to learn 'what' and 'when' to attend simultaneously. Our experimental results show that STCA-SNNs achieve better performance on N-MNIST (99.67%), CIFAR10-DVS (81.6%), and N-Caltech 101 (80.88%) compared with the state-of-the-art SNNs. Meanwhile, our ablation study demonstrates that STCA-SNNs improve the accuracy of event stream classification tasks.
更多查看译文
关键词
spiking neural networks,self-attention,temporal-channel,neuromorphic computing,event streams
AI 理解论文
溯源树
样例
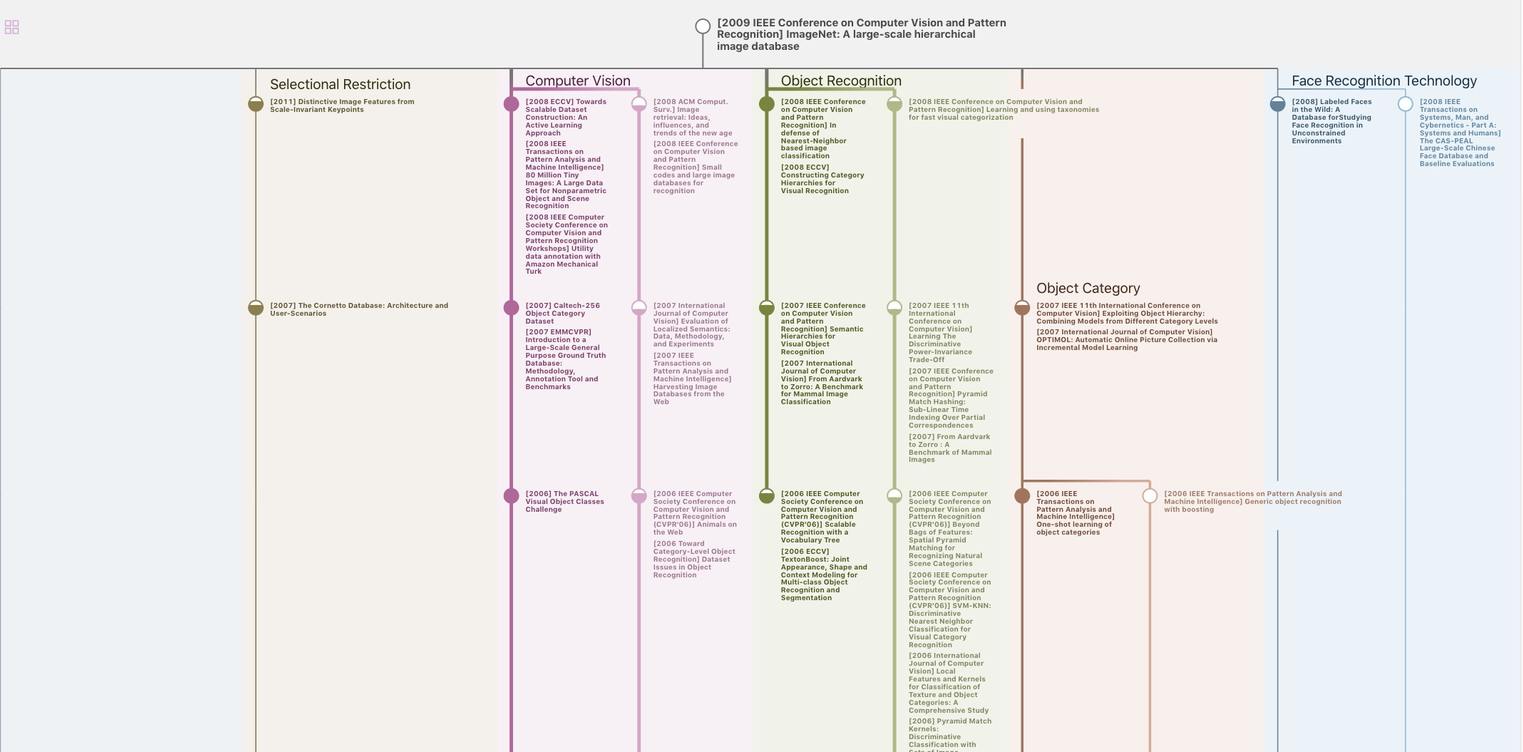
生成溯源树,研究论文发展脉络
Chat Paper
正在生成论文摘要