MALCOM-PSGD: Inexact Proximal Stochastic Gradient Descent for Communication-Efficient Decentralized Machine Learning.
CoRR(2023)
摘要
Recent research indicates that frequent model communication stands as a major bottleneck to the efficiency of decentralized machine learning (ML), particularly for large-scale and over-parameterized neural networks (NNs). In this paper, we introduce MALCOM-PSGD, a new decentralized ML algorithm that strategically integrates gradient compression techniques with model sparsification. MALCOM-PSGD leverages proximal stochastic gradient descent to handle the non-smoothness resulting from the $\ell_1$ regularization in model sparsification. Furthermore, we adapt vector source coding and dithering-based quantization for compressed gradient communication of sparsified models. Our analysis shows that decentralized proximal stochastic gradient descent with compressed communication has a convergence rate of $\mathcal{O}\left(\ln(t)/\sqrt{t}\right)$ assuming a diminishing learning rate and where $t$ denotes the number of iterations. Numerical results verify our theoretical findings and demonstrate that our method reduces communication costs by approximately $75\%$ when compared to the state-of-the-art method.
更多查看译文
关键词
machine learning,malcom-psgd,communication-efficient
AI 理解论文
溯源树
样例
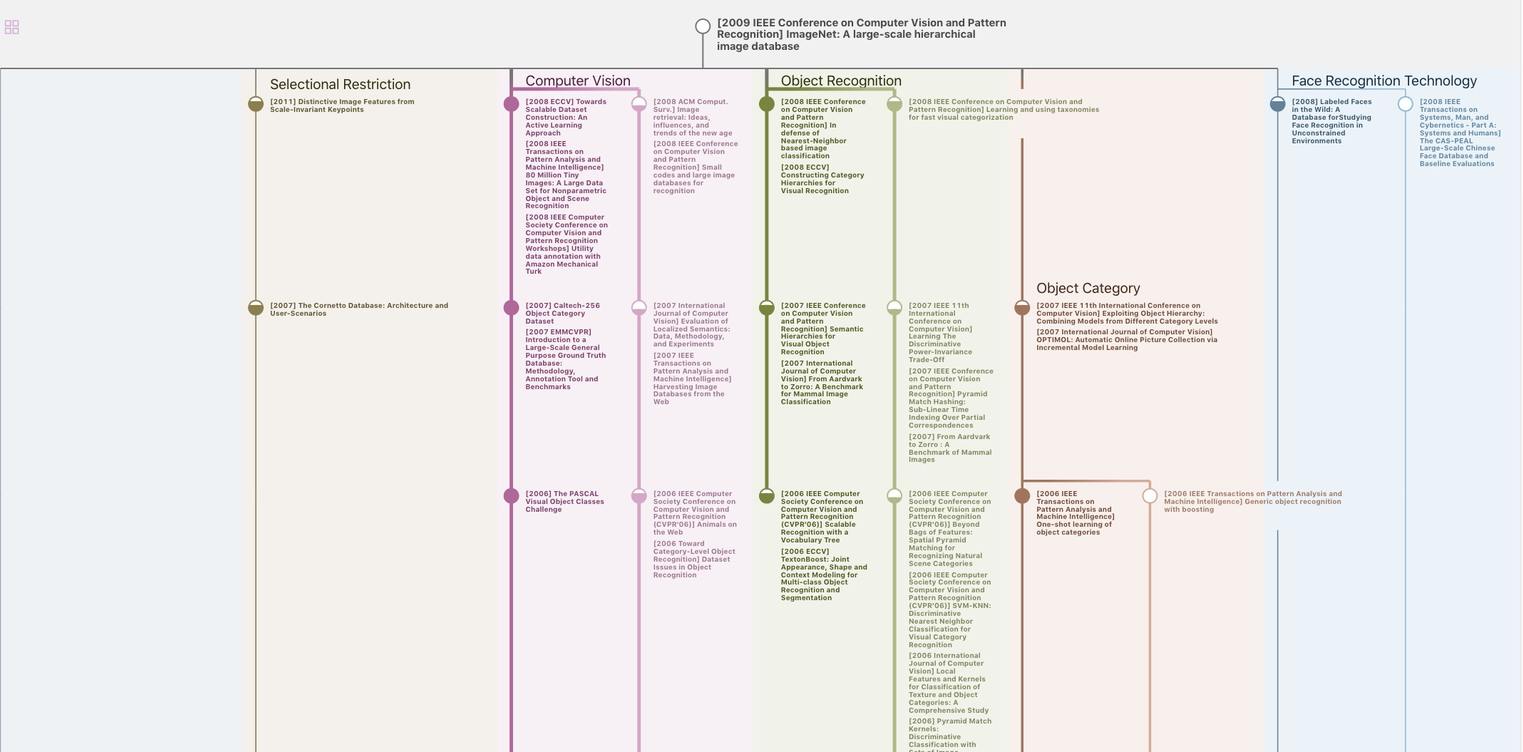
生成溯源树,研究论文发展脉络
Chat Paper
正在生成论文摘要