Perspective on Nanoscaled Magnonic Networks
arXiv (Cornell University)(2023)
摘要
With the rapid development of artificial intelligence in recent years, mankind is facing an unprecedented demand for data processing. Today, almost all data processing is performed using electrons in conventional complementary metal-oxide-semiconductor (CMOS) circuits. Over the past few decades, scientists have been searching for faster and more efficient ways to process data. Now, magnons, the quanta of spin waves, show the potential for higher efficiency and lower energy consumption in solving some specific problems. While magnonics remains predominantly in the realm of academia, significant efforts are being made to explore the scientific and technological challenges of the field. Numerous proof-of-concept prototypes have already been successfully developed and tested in laboratories. In this article, we review the developed magnonic devices and discuss the current challenges in realizing magnonic circuits based on these building blocks. We look at the application of spin waves in neuromorphic networks, stochastic and reservoir computing and discuss the advantages over conventional electronics in these areas. We then introduce a new powerful tool, inverse design magnonics, which has the potential to revolutionize the field by enabling the precise design and optimization of magnonic devices in a short time. Finally, we provide a theoretical prediction of energy consumption and propose benchmarks for universal magnonic circuits.
更多查看译文
关键词
networks
AI 理解论文
溯源树
样例
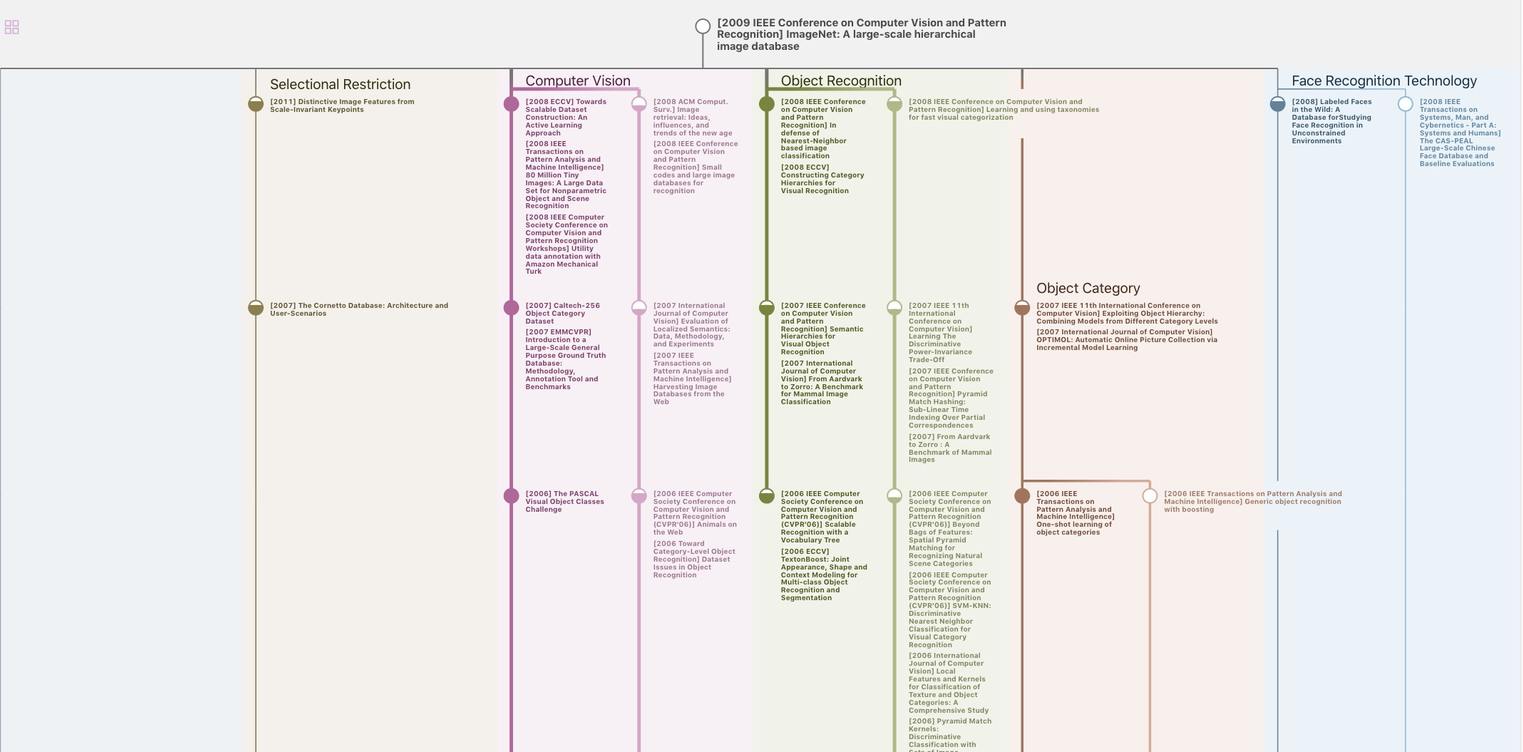
生成溯源树,研究论文发展脉络
Chat Paper
正在生成论文摘要