Vision Impairment prediction for patients diagnosed with Multiple Sclerosis: Cosmos based model training and evaluation
medRxiv (Cold Spring Harbor Laboratory)(2023)
摘要
Objectives Multiple sclerosis (MS) is a complex autoimmune neurological disorder that frequently impacts vision. One of the most frequent initial presentations of MS is acute vision loss due to optic neuritis, an acute disorder caused by MS involvement with the optic nerve. While vision impairment is often the first sign of MS, it can occur or recur at any time during the patient's course. In this study, we aim to develop and evaluate machine learning models to predict vision impairment in patients with MS, both at the time of first MS diagnosis and throughout their course of care. Early awareness and intervention in patients likely to have vision loss can help preserve patient quality of life. Materials and Methods Using the Epic Cosmos de-identified electronic health record (EHR) dataset, we queried 213+ million patients to extract our MS cohort. Cases were defined as MS patients with vision impairment or optic neuritis (VI) following their first MS diagnosis, while controls were MS patients without VI. We trained logistic regression (LR), light gradient boosting machine (LGBM), and recurrent neural network (RNN) models to predict future VI in MS patients. The models were evaluated for two distinct clinical tasks: prediction of VI at the time of the first MS diagnosis and prediction of VI at the most recent visit. Similarly, we trained the models on different segments of the patient trajectory including up until the first MS diagnosis (MS-First Diagnosis), or until the most recent visit before developing the outcome (MS-Progress) as well as the combination of both (MS-General). Finally, we trained a survival model with the goal of predicting patient likelihood of vision loss over time. We compared the models' performance using AUROC, AUPRC, and Brier scores. Results We extracted a cohort of 377,097 patients with MS, including 42,281 VI cases. Our trained models achieved ~80% AUROC, with RNN-based models outperforming LGBM and LR (79.6% vs 72.8% and 68.6%, respectively) when considering the full patient trajectory. The MS-General RNN model had the highest AUROC (64.4%) for predicting VI at the first MS diagnosis. The MS-Progress survival model achieved a 75% concordance index on the full trajectory, while the more clinically relevant MS-First Diagnosis model achieved 63.1% at initial diagnosis. Discussion and Conclusion The MS-Progress and MS-General RNN models performed best in both prediction scenarios. While MS-General achieved the best performance at the time of first MS diagnosis with around 1% AUROC increase compared to the MS-First Diagnosis model, it showed around 1% AUROC decrease on the MS progress scenario. All RNN survival models performed the best when they were trained on data corresponding to the evaluation use-case scenarios. RNN based models showed promising performance that demonstrates that they can be useful clinical tools to predict risk of future VI events in patients with MS. Further development of these models will focus on expanding to predict other comorbidities associated with MS relapse or progression.
### Competing Interest Statement
The authors have declared no competing interest.
### Funding Statement
This study did not receive any funding.
### Author Declarations
I confirm all relevant ethical guidelines have been followed, and any necessary IRB and/or ethics committee approvals have been obtained.
Yes
The details of the IRB/oversight body that provided approval or exemption for the research described are given below:
Both the University of Texas Health Science Center, as well as the Western Michigan University School of Medicine Institutional review boards, waived ethical approval for this work.
I confirm that all necessary patient/participant consent has been obtained and the appropriate institutional forms have been archived, and that any patient/participant/sample identifiers included were not known to anyone (e.g., hospital staff, patients or participants themselves) outside the research group so cannot be used to identify individuals.
Yes
I understand that all clinical trials and any other prospective interventional studies must be registered with an ICMJE-approved registry, such as ClinicalTrials.gov. I confirm that any such study reported in the manuscript has been registered and the trial registration ID is provided (note: if posting a prospective study registered retrospectively, please provide a statement in the trial ID field explaining why the study was not registered in advance).
Yes
I have followed all appropriate research reporting guidelines, such as any relevant EQUATOR Network research reporting checklist(s) and other pertinent material, if applicable.
Yes
data used in this study came from Epic Cosmos, a community collaboration of health systems representing over 220 Million patient records from over 1277 hospitals and 27200 clinics. The current count values for patients, hospitals, and clinics are available on cosmos.epic.com. All data produced in the present work are contained in the manuscript.
更多查看译文
关键词
multiple sclerosis,model training,vision,prediction,cosmos
AI 理解论文
溯源树
样例
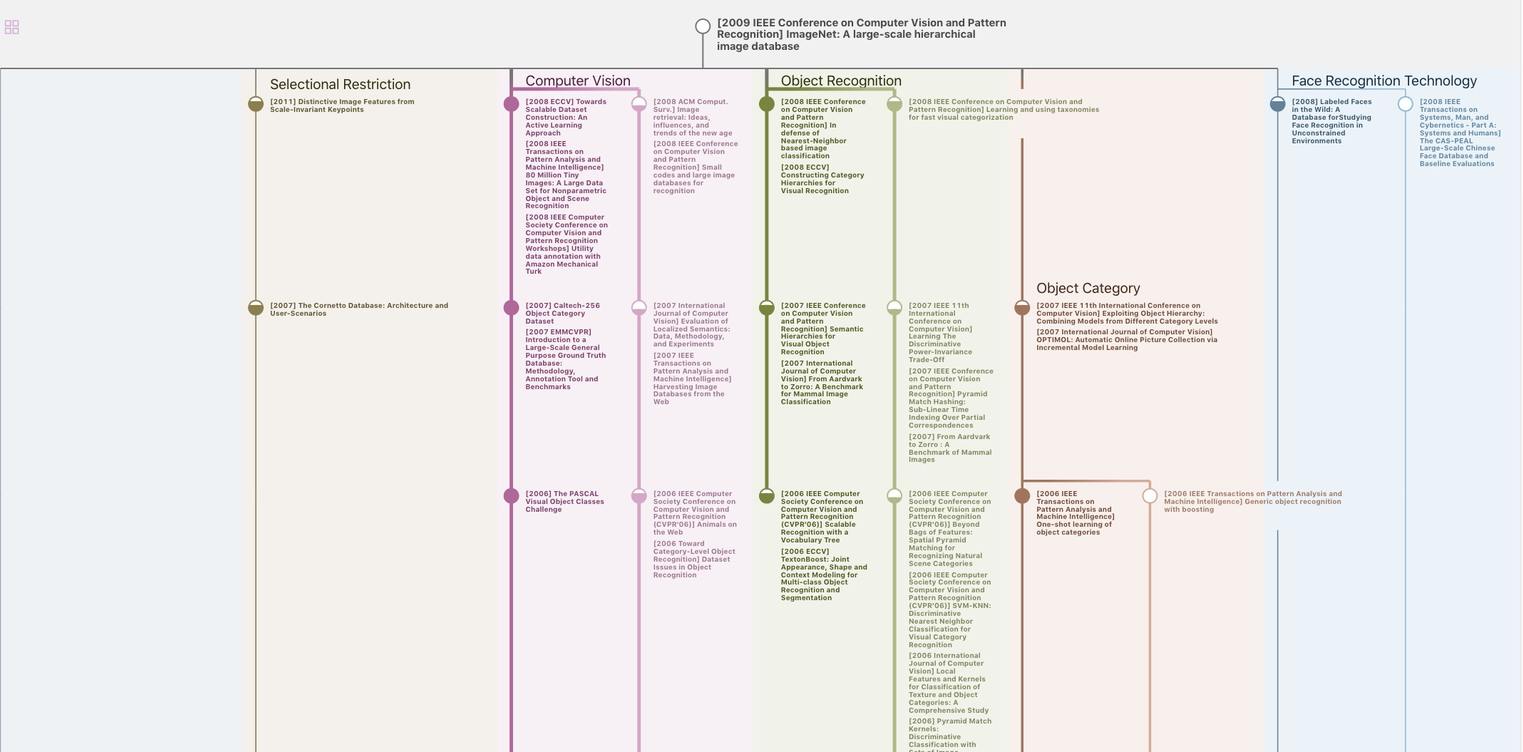
生成溯源树,研究论文发展脉络
Chat Paper
正在生成论文摘要