Extracting symptoms from free-text responses using ChatGPT among COVID-19 cases in Hong Kong
CLINICAL MICROBIOLOGY AND INFECTION(2024)
摘要
Objectives: To investigate the feasibility and performance of Chat Generative Pretrained Transformer (ChatGPT) in converting symptom narratives into structured symptom labels. Methods: We extracted symptoms from 300 deidentified symptom narratives of COVID-19 patients by a computer-based matching algorithm (the standard), and prompt engineering in ChatGPT. Common symptoms were those with a prevalence >10% according to the standard, and similarly less common symptoms were those with a prevalence of 2-10%. The precision of ChatGPT was compared with the standard using sensitivity and specificity with 95% exact binomial CIs (95% binCIs). In ChatGPT, we prompted without examples (zero-shot prompting) and with examples (few-shot prompting). Results: In zero-shot prompting, GPT-4 achieved high specificity (0.947 [95% binCI: 0.894-0.978]-1.000 [95% binCI: 0.965-0.988, 1.000]) for all symptoms, high sensitivity for common symptoms (0.853 [95% binCI: 0.689-0.950]-1.000 [95% binCI: 0.951-1.00 0]), and moderate sensitivity for less common symptoms (0.200 [95% binCI: 0.043-0.481]-1.000 [95% binCI: 0.590-0.815, 1.000]). Few-shot prompting increased the sensitivity and specificity. GPT-4 outperformed GPT-3.5 in response accuracy and consistent labelling. Discussion: This work substantiates ChatGPT's role as a research tool in medical fields. Its performance in converting symptom narratives to structured symptom labels was encouraging, saving time and effort in compiling the task-specific training data. It potentially accelerates free-text data compilation and synthesis in future disease outbreaks and improves the accuracy of symptom checkers. Focused prompt training addressing ambiguous descriptions impacts medical research positively. Wan In Wei, Clin Microbiol Infect 2024;30:142.e1-142.e3 (c) 2023 European Society of Clinical Microbiology and Infectious Diseases. Published by Elsevier Ltd. All rights reserved.
更多查看译文
关键词
ChatGPT,Entity recognition,Large language model,Symptom extraction,Symptom narratives,Symptom science
AI 理解论文
溯源树
样例
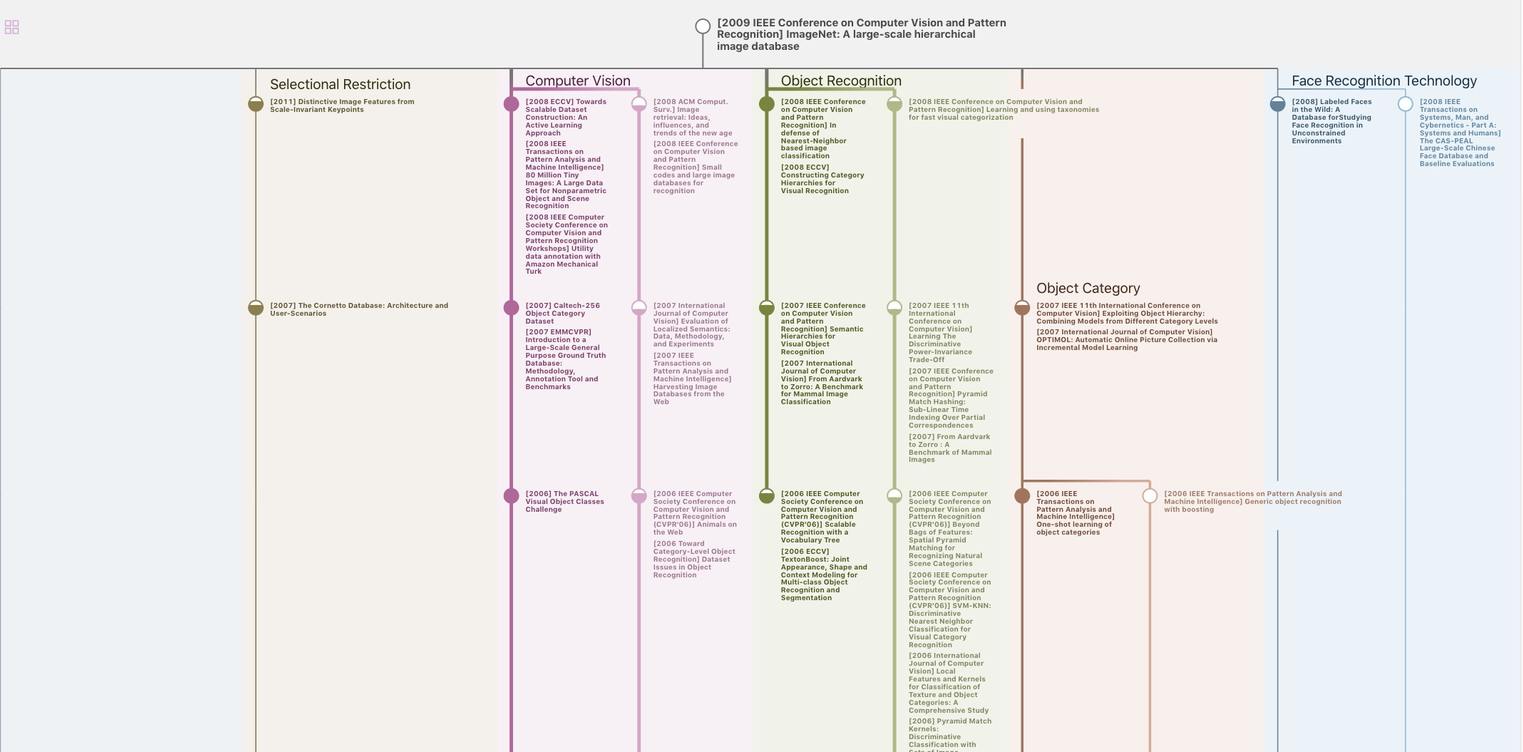
生成溯源树,研究论文发展脉络
Chat Paper
正在生成论文摘要