Future Prediction of Close Contacts in IoT-based Contact Tracing System using a New Real-Life Dataset.
IEEE journal of biomedical and health informatics(2023)
摘要
Contact tracing is an effective method for mitigating the infectious diseases spread and it played a crucial role in reducing COVID-19 outbreak. Since the pandemic, there has been an increased concern regarding people's health in hospital and office settings, as these limited air exchange spaces provide a conductive medium for virus spread. Various technologies were used to recognize close contacts autonomously, in addition, multiple machine learning attempts were carried out to determine proximity in contact tracing. This study, however, proposes a unique concept in contact tracing: forecasting future close contact prior to occurrence in order to regulate and control it rather than tracking past occurrences. For our research, we constructed a completely new real-life dataset that was collected during the pandemic in a hospital infectious ward (Alfred Hospital, Melbourne, Australia) utilizing a Bluetooth Low Energy (BLE) Internet of Things (IoT) system. Our prediction technique considers two types of environments: single transceiver environments and multiple transceivers settings, these transceivers record the nearby tags' BLE received signal strength indicator (RSSI) values. The system employs mathematical models and supervised machine learning (ML) algorithms to solve regression and classification problems for workers' pattern recognition within the environment. The output is compared using different metrics, such as efficiency, which reached more than 80%, root mean square errors and mean absolute errors which were as low as 2.4 and 1.2 respectively in some models.
更多查看译文
关键词
BLE,classification,contact tracing,COVID-19,hospital setting,IoT,pattern recognition/detection,proximity detection,regression,RSSI,supervised learning,wearable tags
AI 理解论文
溯源树
样例
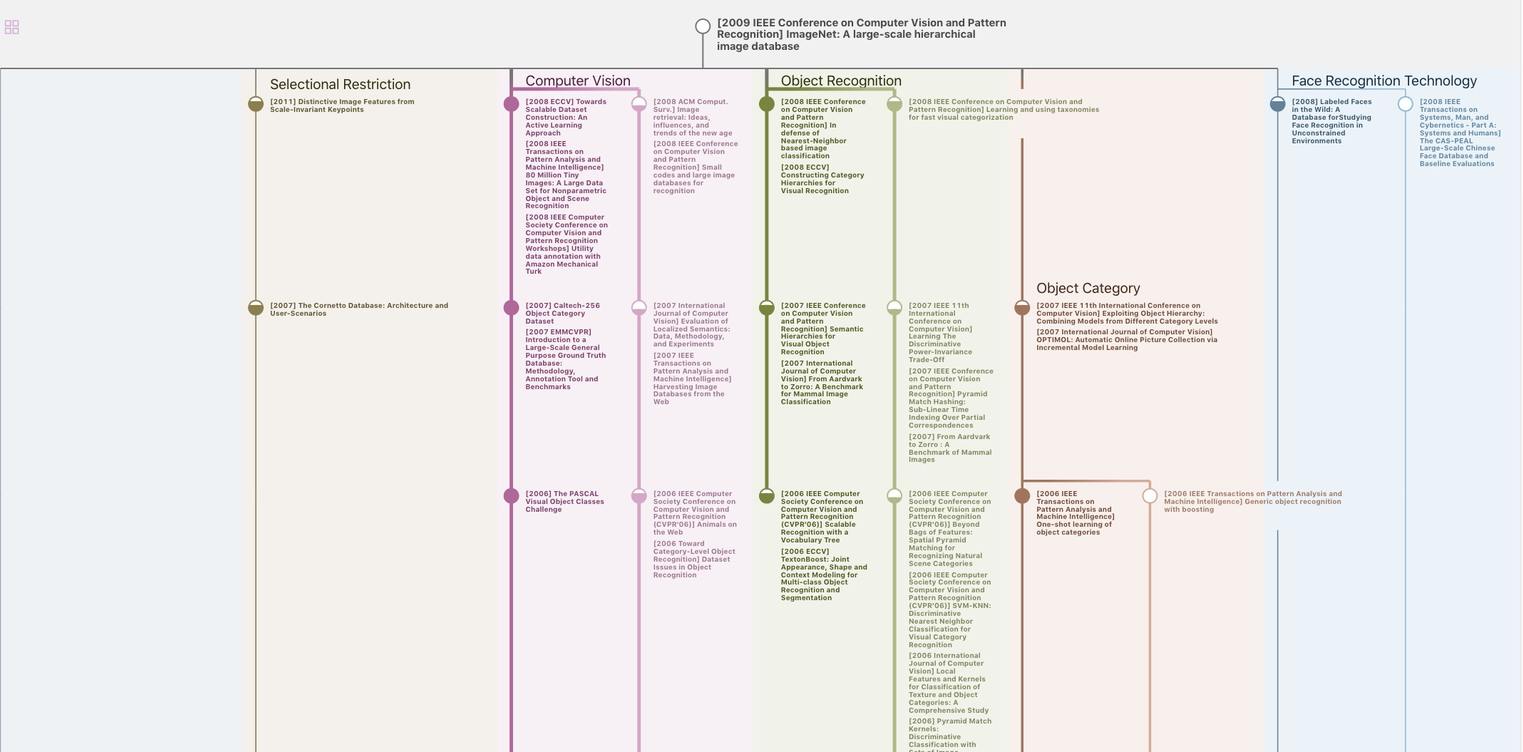
生成溯源树,研究论文发展脉络
Chat Paper
正在生成论文摘要