Cosine Multilinear Principal Component Analysis for Recognition
IEEE TRANSACTIONS ON BIG DATA(2023)
摘要
Existing two-dimensional principal component analysis methods can only handle second-order tensors (i.e., matrices). However, with the advancement of technology, tensors of order three and higher are gradually increasing. This brings new challenges to dimensionality reduction. Thus, a multilinear method called MPCA was proposed. Although MPCA can be applied to all tensors, using the square of the F-norm makes it very sensitive to outliers. Several two-dimensional methods, such as Angle 2DPCA, have good robustness but cannot be applied to all tensors. We extend the robust Angle 2DPCA method to a multilinear method and propose Cosine Multilinear Principal Component Analysis (CosMPCA) for tensor representation. Our CosMPCA method considers the relationship between the reconstruction error and projection scatter and selects the cosine metric. In addition, our method naturally uses the F-norm to reduce the impact of outliers. We introduce an iterative algorithm to solve CosMPCA. We provide detailed theoretical analysis in both the proposed method and the analysis of the algorithm. Experiments show that our method is robust to outliers and is suitable for tensors of any order.
更多查看译文
关键词
Tensors,Principal component analysis,Mathematical models,Linear programming,Iterative methods,Robustness,Matrix decomposition,Multilinear principal component analysis,angle,tensor analysis,pattern recognition
AI 理解论文
溯源树
样例
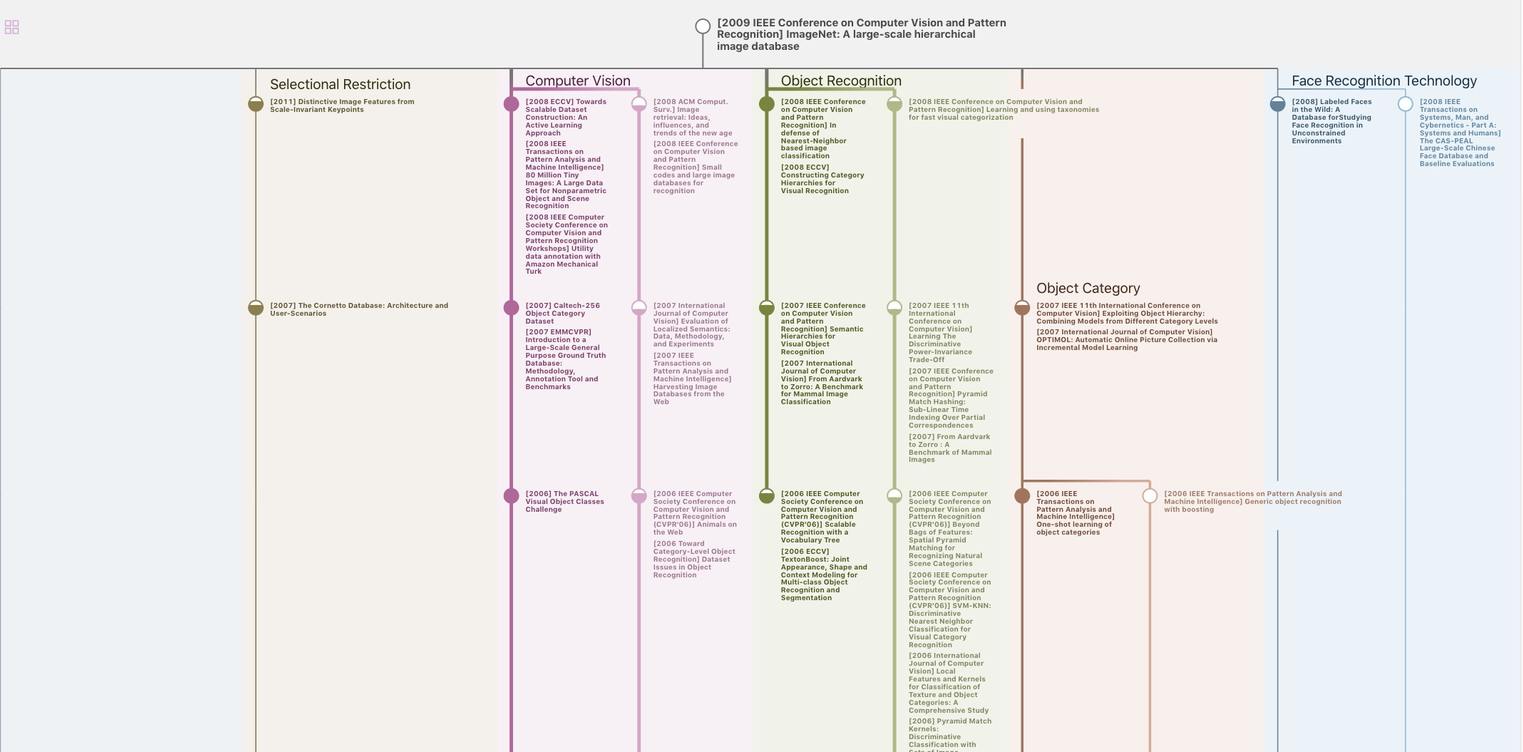
生成溯源树,研究论文发展脉络
Chat Paper
正在生成论文摘要