Task-Based Polar Decomposition Using SLATE on Massively Parallel Systems with Hardware Accelerators.
SC-W '23: Proceedings of the SC '23 Workshops of The International Conference on High Performance Computing, Network, Storage, and Analysis(2023)
摘要
We investigate a new task-based implementation of the polar decomposition on massively parallel systems augmented with multiple GPUs using SLATE. We implement the iterative QR Dynamically-Weighted Halley (QDWH) algorithm, whose building blocks mainly consist of compute-bound matrix operations, allowing for high levels of parallelism to be exploited on various hardware architectures, such as NVIDIA, AMD, and Intel GPU-based systems. To achieve both performance and portability, we implement our QDWH-based polar decomposition in the SLATE library, which uses efficient techniques in dense linear algebra, such as 2D block cyclic data distribution and communication-avoiding algorithms, as well as modern parallel programming approaches, such as dynamic scheduling and communication overlapping, and uses OpenMP tasks to track data dependencies. We report numerical accuracy and performance results. The benchmarking campaign reveals up to an 18-fold performance speedup of the GPU accelerated implementation compared to the existing state-of-the-art implementation for the polar decomposition.
更多查看译文
关键词
polar decomposition,massively parallel systems,hardware accelerators,slate,task-based
AI 理解论文
溯源树
样例
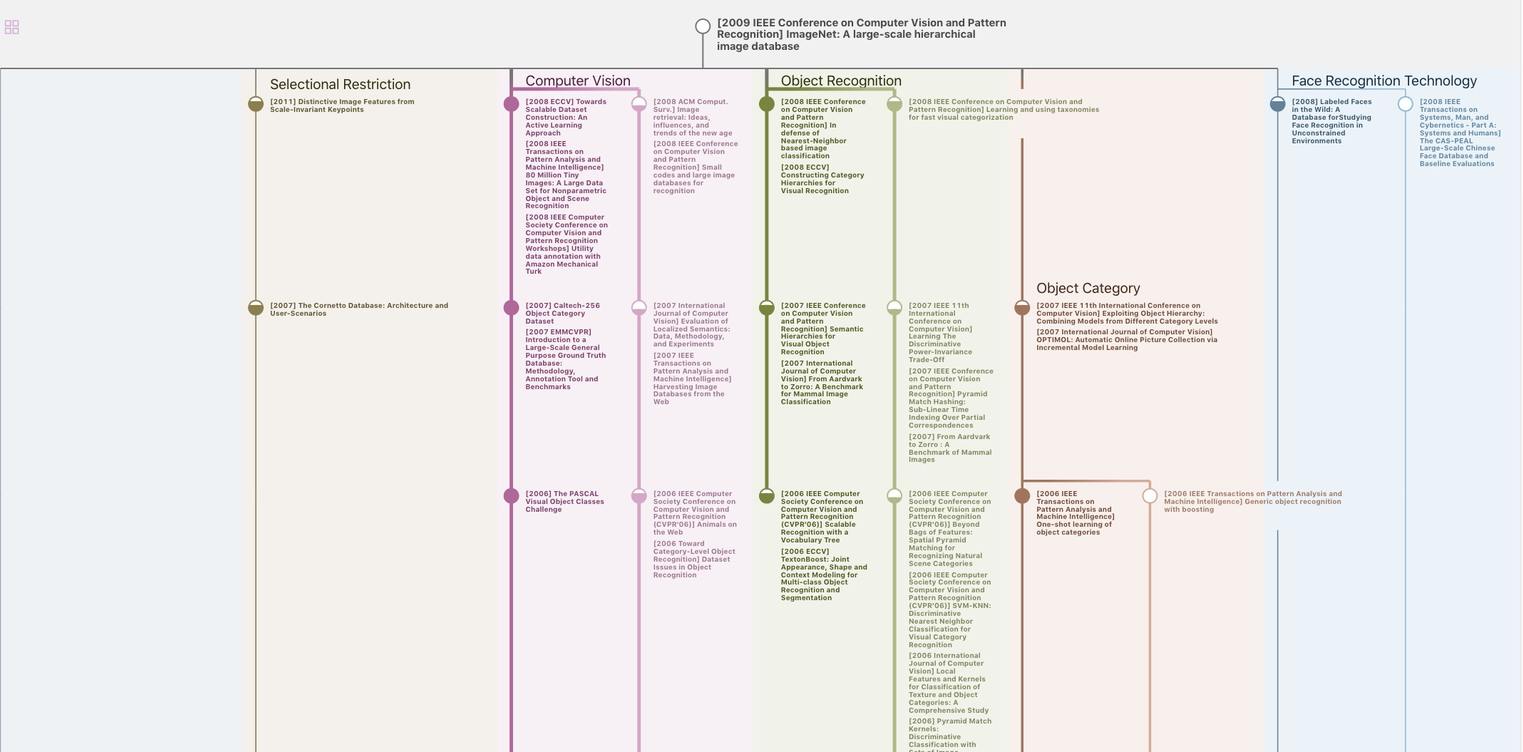
生成溯源树,研究论文发展脉络
Chat Paper
正在生成论文摘要