Drought driving mechanism and risk situation prediction based on machine learning models in the Yellow River Basin, China
Geomatics, Natural Hazards & Risk(2023)
摘要
Under global warming, the acceleration of the water cycle has increased the risk of drought in the Yellow River Basin. Revealing the drought driving mechanisms in the basin and understanding the risk situation of drought have become particularly important. This paper uses wavelet analysis and transfer entropy to analyze the drought driving mechanisms. In addition, an Improved Particle Swarm Optimization (IPSO) coupled with Long Short-Term Memory (LSTM) is used for drought risk prediction. The results are as follows: (1) Hydrological drought lags behind meteorological drought by 2-3 months, and they show two main periods on different time scales, which are 5-6 months and 8-14 months, respectively. (2) Rainfall, runoff, temperature, humidity, and vapor pressure are the main drought driving factors, with rainfall and humidity having the most significant impact. (3) The IPSO-LSTM model has improved the process of selecting model parameters based on empirical experiences in the LSTM model, improving the prediction accuracy by an average of 3.1%. This paper provides a scientific basis for water resource management and drought risk assessment in the basin, to better cope with future climate challenges.
更多查看译文
关键词
Driving factors, Drought risk prediction, Transfer entropy, Machine learning, The yellow river basin
AI 理解论文
溯源树
样例
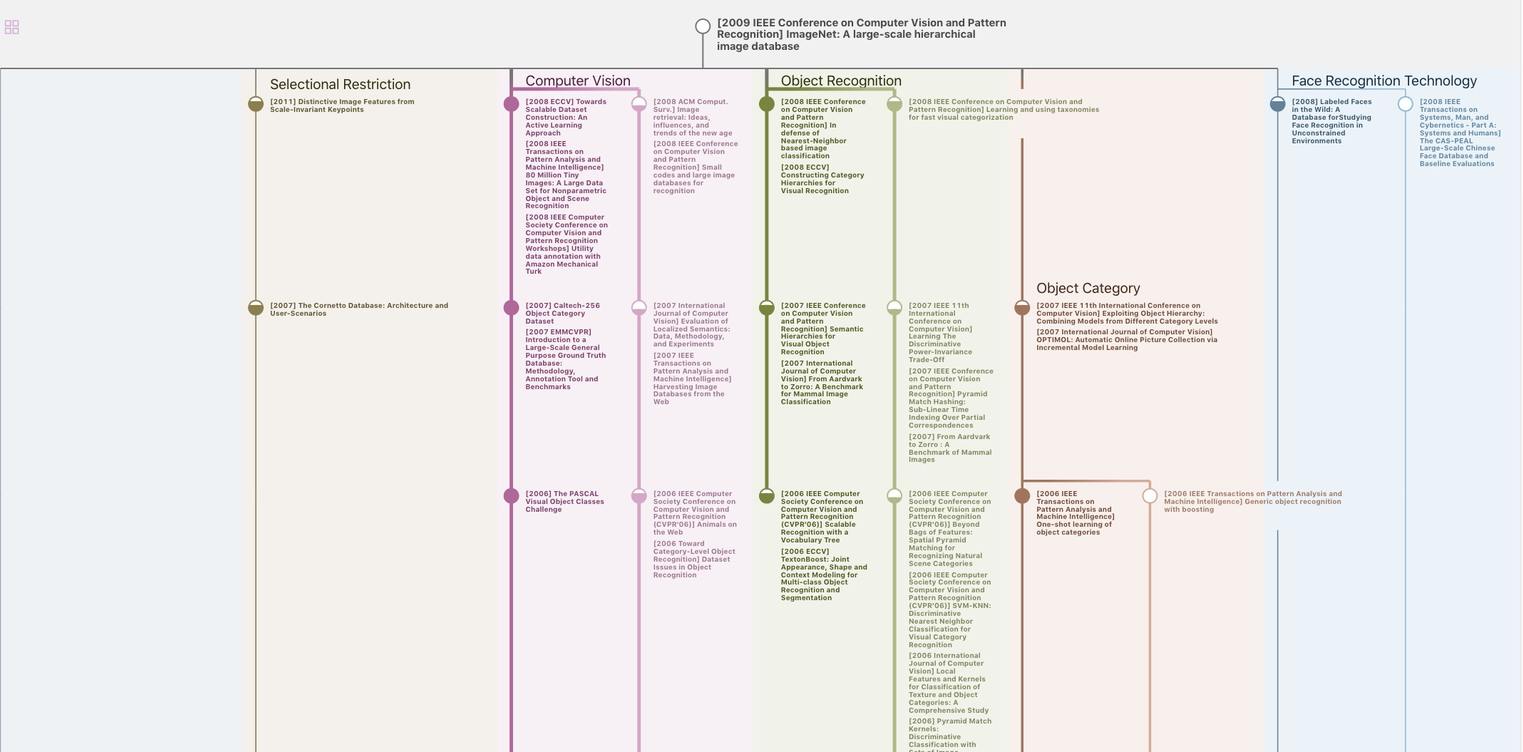
生成溯源树,研究论文发展脉络
Chat Paper
正在生成论文摘要