Machine Learning Applied to Single-Molecule Activity Prediction.
SC-W '23: Proceedings of the SC '23 Workshops of The International Conference on High Performance Computing, Network, Storage, and Analysis(2023)
摘要
Catalytic processes are used in about 1/3 of US manufacturing, from the field of chemical engineering to renewable energy. Assessing the activity of single-molecules, or individual molecules, is necessary to the development of efficient catalysts. Their heterogeneity structure leads to particle-specific catalytic activity. Experimentation with single-molecules can be time consuming and difficult. We purpose a Machine learning (ML) model that allows chemical researchers to run shorter single-molecule experiments to obtain the same level of results. We use common and widely understood ML methods to reduce complexity and enable accessibility to the chemical engineering community. We reduce the experiment time by up to 83%. Our evaluation shows that a small data set is sufficient to train an acceptable model. 300 experiments are needed, including the validation set. We use a well understood multilayer perceptron (MLP) model. We show that more complex models are not necessary and simpler methods are not sufficient.
更多查看译文
AI 理解论文
溯源树
样例
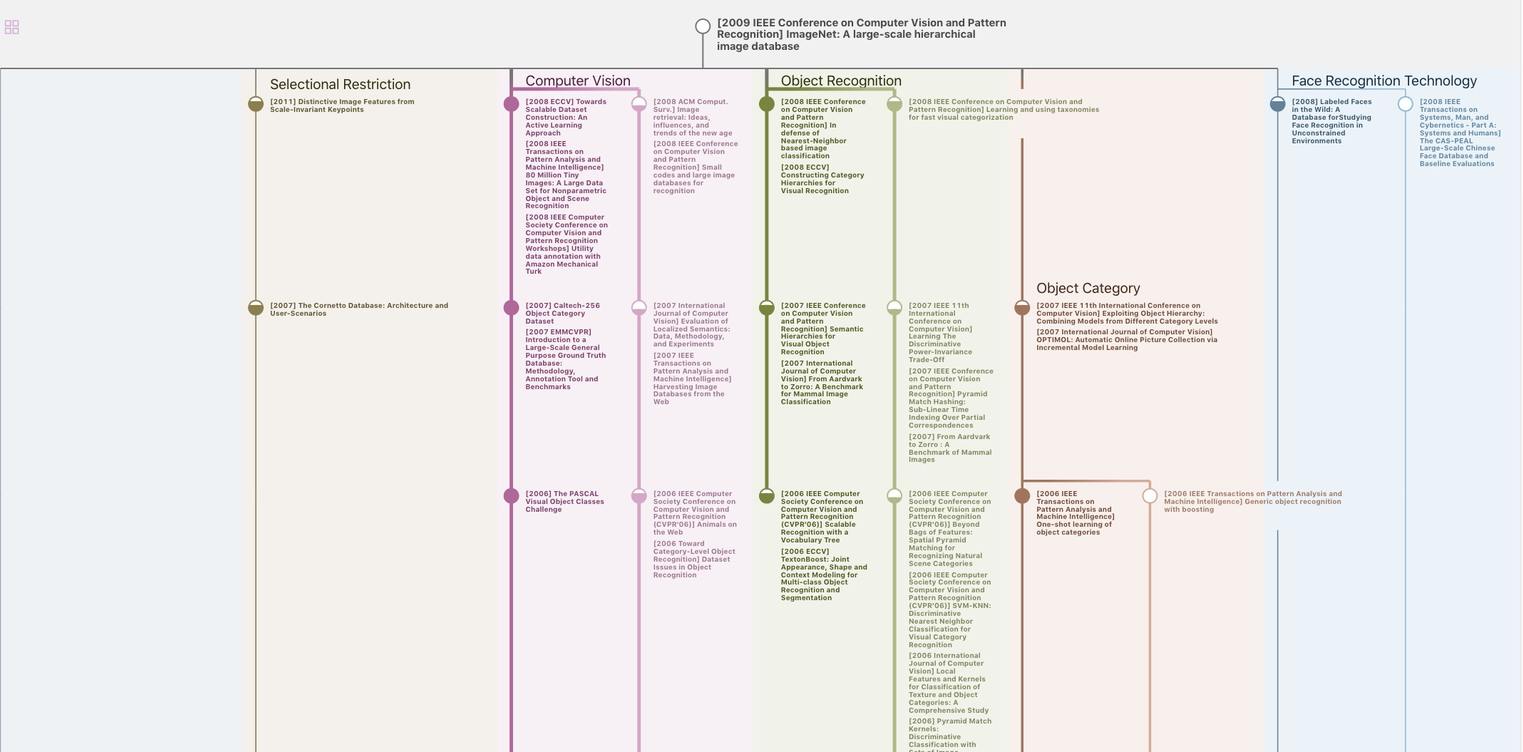
生成溯源树,研究论文发展脉络
Chat Paper
正在生成论文摘要