A Machine Learning-Based Unenhanced Radiomics Approach to Distinguishing Between Benign and Malignant Breast Lesions Using T2-Weighted and Diffusion-Weighted MRI
Journal of magnetic resonance imaging : JMRI(2023)
摘要
Journal of Magnetic Resonance ImagingEarly View Editorial Editorial for “A Machine Learning-Based Unenhanced Radiomics Approach to Distinguishing Between Benign and Malignant Breast Lesions Using T2-Weighted and Diffusion-Weighted MRI” Weiguo Li PhD, Corresponding Author Weiguo Li PhD [email protected] orcid.org/0000-0001-8125-3824 Department of Radiology, Northwestern University, Evanston, Illinois, USA Department of Biomedical Engineering, University of Illinois at Chicago, Chicago, Illinois, USA Address reprint requests to: W.L., Department of Radiology, Northwestern University, 737 N Michigan Ave, 16th Suite, Chicago, IL 60611, USA. E-mail: [email protected]Search for more papers by this author Weiguo Li PhD, Corresponding Author Weiguo Li PhD [email protected] orcid.org/0000-0001-8125-3824 Department of Radiology, Northwestern University, Evanston, Illinois, USA Department of Biomedical Engineering, University of Illinois at Chicago, Chicago, Illinois, USA Address reprint requests to: W.L., Department of Radiology, Northwestern University, 737 N Michigan Ave, 16th Suite, Chicago, IL 60611, USA. E-mail: [email protected]Search for more papers by this author First published: 10 November 2023 https://doi.org/10.1002/jmri.29132 Evidence Level: 5 Technical Efficacy: Stage 5 Read the full textAboutPDF ToolsRequest permissionExport citationAdd to favoritesTrack citation ShareShare Give accessShare full text accessShare full-text accessPlease review our Terms and Conditions of Use and check box below to share full-text version of article.I have read and accept the Wiley Online Library Terms and Conditions of UseShareable LinkUse the link below to share a full-text version of this article with your friends and colleagues. Learn more.Copy URL Share a linkShare onEmailFacebookTwitterLinkedInRedditWechat No abstract is available for this article. References 1Warner E, Messersmith H, Causer P, Eisen A, Shumak R, Plewes D. Systematic review: Using magnetic resonance imaging to screen women at high risk for breast cancer. Ann Intern Med 2008; 148(9): 671-679. 2Fraum TJ, Ludwig DR, Bashir MR, Fowler KJ. Gadolinium-based contrast agents: A comprehensive risk assessment. J Magn Reson Imaging 2017; 46(2): 338-353. 3Brünjes R, Hofmann T. Anthropogenic gadolinium in freshwater and drinking water systems. Water Res 2020; 182:115966. 4Leithner D, Moy L, Morris EA, Marino MA, Helbich TH, Pinker K. Abbreviated mri of the breast: Does it provide value? J Magn Reson Imaging 2019; 49(7): e85-e100. 5Liu Y, Jia X, Zhao J, et al. A machine learning–based unenhanced radiomics approach to distinguishing between benign and malignant breast lesions using T2-weighted and diffusion-weighted MRI. J Magn Reson Imaging 2023. https://doi.org/10.1002/jmri.29111. 6Zhu W, Huang H, Zhou Y, et al. Automatic segmentation of white matter hyperintensities in routine clinical brain mri by 2d vb-net: A large-scale study. Front Aging Neurosci 2022; 14:915009. 7Sui H, Ma R, Liu L, Gao Y, Zhang W, Mo Z. Detection of incidental esophageal cancers on chest ct by deep learning. Front Oncol 2021; 11:700210. 8Beňačka R, Szabóová D, Guľašová Z, Hertelyová Z, Radoňák J. Classic and new markers in diagnostics and classification of breast cancer. Cancers (Basel) 2022; 14(21):5444. Early ViewOnline Version of Record before inclusion in an issue ReferencesRelatedInformation
更多查看译文
关键词
malignant breast lesions,radiomics,mri</scp>”,<scp>diffusion‐weighted
AI 理解论文
溯源树
样例
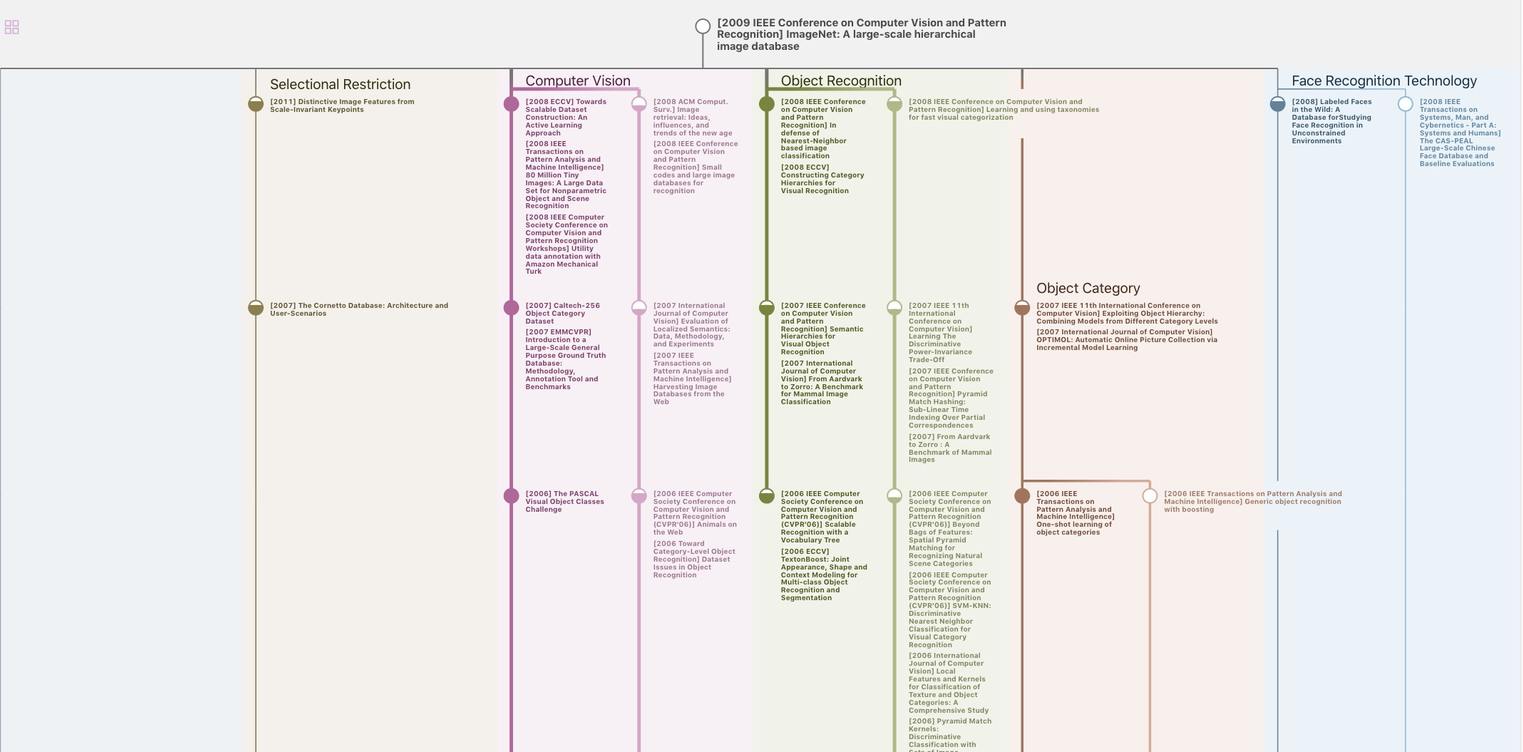
生成溯源树,研究论文发展脉络
Chat Paper
正在生成论文摘要