Intelligent phenotype-detection and gene expression profile generation with generative adversarial networks
JOURNAL OF THEORETICAL BIOLOGY(2024)
摘要
Gene expression analysis is valuable for cancer type classification and identifying diverse cancer phenotypes. The latest high-throughput RNA sequencing devices have enabled access to large volumes of gene expression data. However, we face several challenges, such as data security and privacy, when we develop machine learning-based classifiers for categorizing cancer types with these datasets. To address these issues, we propose IP3G (Intelligent Phenotype-detection and Gene expression profile Generation with Generative adversarial network), a model based on Generative Adversarial Networks. IP3G tackles two major problems: augmenting gene expression data and unsupervised phenotype discovery. By converting gene expression profiles into 2-Dimensional images and leveraging IP3G, we generate new profiles for specific phenotypes. IP3G learns disentangled representations of gene expression patterns and identifies phenotypes without labeled data. We improve the objective function of the GAN used in IP3G by employing the earth mover distance and a novel mutual information function. IP3G outperforms clustering methods like k-Means, DBSCAN, and GMM in unsupervised phenotype discovery, while also surpassing SVM and CNN classification accuracy by up to 6% through gene expression profile augmentation. The source code for the developed IP3G is accessible to the public on GitHub.
更多查看译文
关键词
Generative adversarial networks,Gene expression,Augmentation of RNA-seq data,Cancer diagnosis,Cancer phenotype detection
AI 理解论文
溯源树
样例
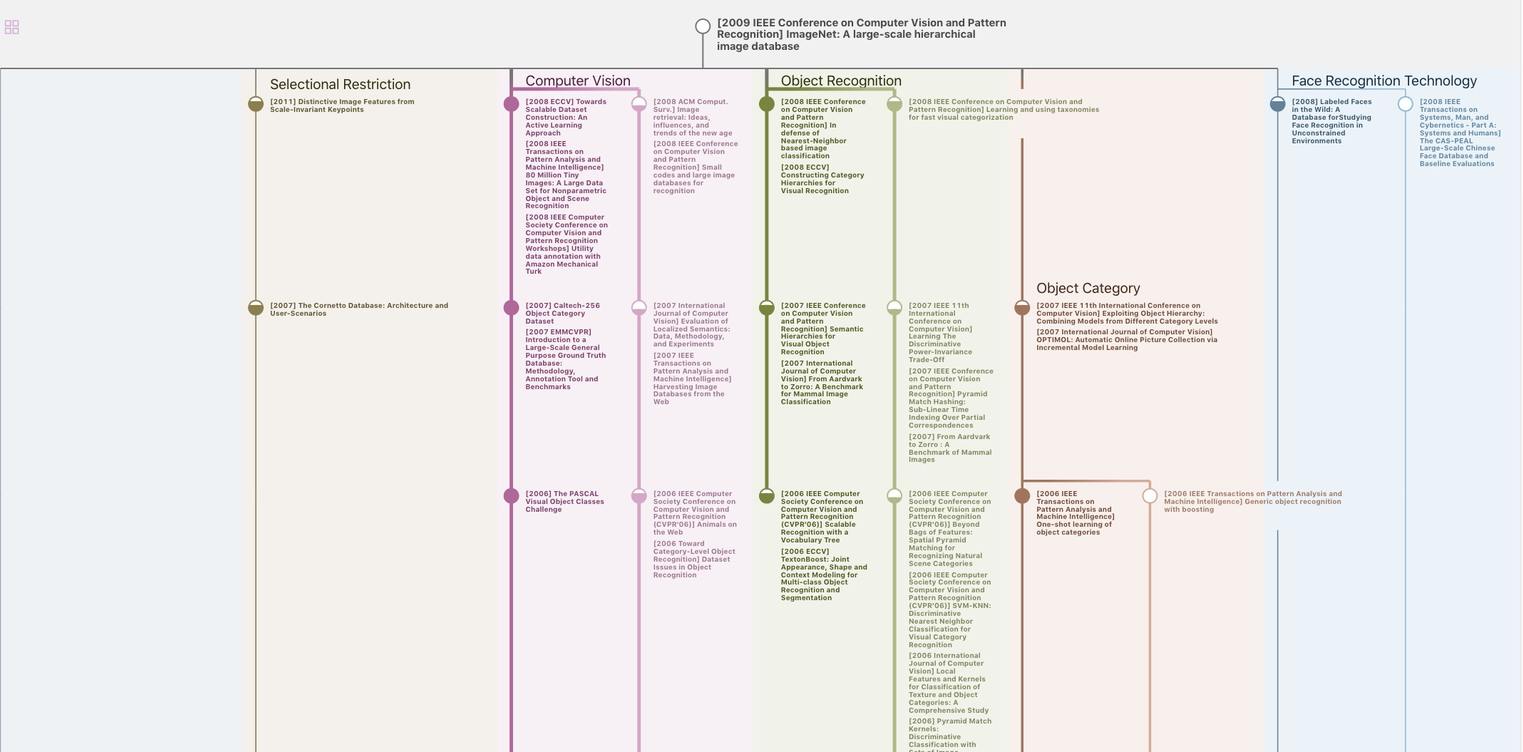
生成溯源树,研究论文发展脉络
Chat Paper
正在生成论文摘要