Forecasting Treatment Outcomes Over Time Using Alternating Deep Sequential Models
IEEE TRANSACTIONS ON BIOMEDICAL ENGINEERING(2024)
摘要
Medical decision making often relies on accurately forecasting future patient trajectories. Conventional approaches for patient progression modeling often do not explicitly model treatments when predicting patient trajectories and outcomes. In this paper, we propose Alternating Transformer (AL-Transformer) to jointly model treatment and clinical outcomes over time as alternating sequential models. We leverage causal convolution in the self-attention mechanism of AL-Transformer to incorporate local spatial information in the sequence, thus enhancing the model's ability to capture local contextual information of the sequence. Additionally, to predict the sparse treatment, a constraint learned by a convolutional neural network (CNN) is used to constrain the sparse treatment output. Experimental results on two datasets from patients with sepsis and respiratory failure extracted from the Medical Information Mart for Intensive Care (MIMIC) database demonstrate the effectiveness of the proposed approach, outperforming existing state-of-the-art methods.
更多查看译文
关键词
Machine learning,sequential models,time series forecasting,treatment outcome prediction,clinical decision making
AI 理解论文
溯源树
样例
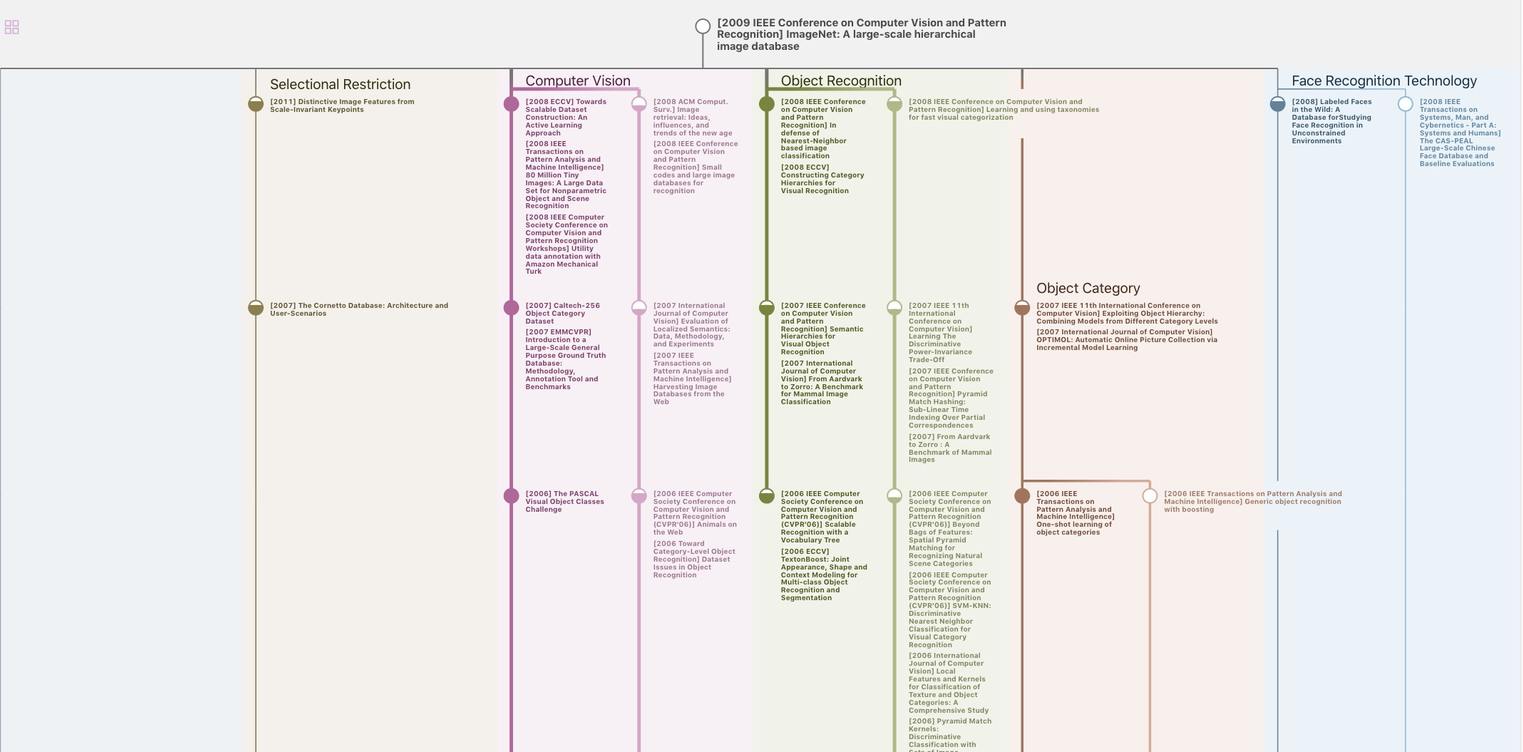
生成溯源树,研究论文发展脉络
Chat Paper
正在生成论文摘要