Hippocampal networks support reinforcement learning in partially observable environments
bioRxiv (Cold Spring Harbor Laboratory)(2023)
摘要
Mastering navigation in environments with limited visibility is crucial for survival. While the hippocampus has been associated with goal-oriented navigation, its specific role in real-world behaviour, particularly in scenarios with partial observability, remains elusive. To investigate this, we combined deep reinforcement learning (RL) modelling with behavioural and neural data analysis. First, we trained RL agents to perform reward-based navigational tasks in partially observable environments. We show that agents equipped with recurrent hippocampal circuitry, as opposed to a purely feedforward network, successfully learned the tasks, resembling animal behaviour. By employing neural dimensionality reduction, our models predicted reward, strategy and temporal representations, which we validated using large-scale hippocampal neuronal recordings. Moreover, hippocampal RL agents predicted state-specific trajectories and action certainty, which mirror empirical findings. In contrast, agents trained in fully observable environments failed to capture experimental data, implying that partial observability is an intrinsic aspect of goal-driven experimental research. Crucially, hippocampal-like RL agents demonstrated improved generalisation across novel task conditions. In summary, our findings suggest a key role of hippocampal networks in facilitating learning in naturalistic environments.
### Competing Interest Statement
The authors have declared no competing interest.
更多查看译文
关键词
reinforcement learning,environments,networks
AI 理解论文
溯源树
样例
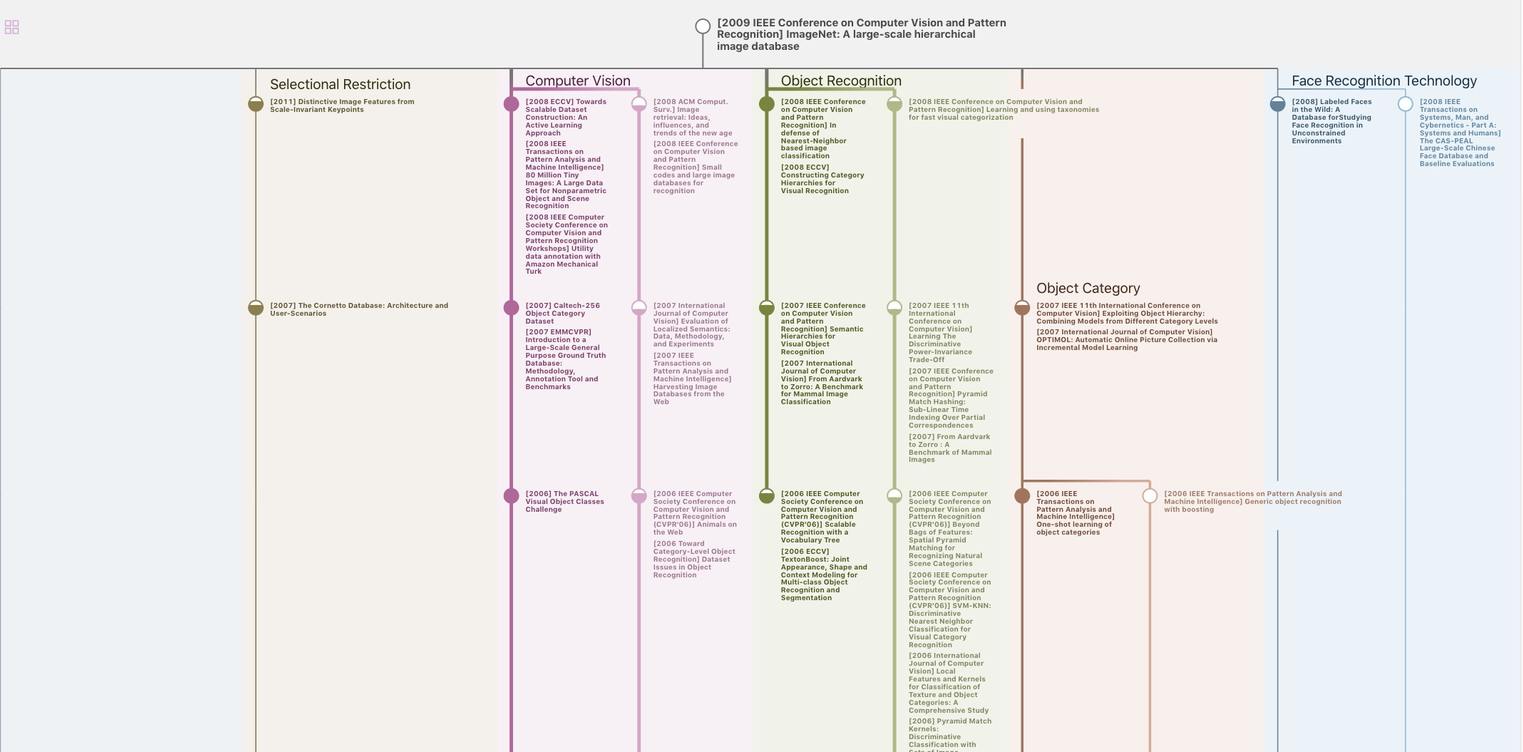
生成溯源树,研究论文发展脉络
Chat Paper
正在生成论文摘要