CBF-IDS: Addressing Class Imbalance Using CNN-BiLSTM with Focal Loss in Network Intrusion Detection System
Applied Sciences(2023)
摘要
The importance of network security has become increasingly prominent due to the rapid development of network technology. Network intrusion detection systems (NIDSs) play a crucial role in safeguarding networks from malicious attacks and intrusions. However, the issue of class imbalance in the dataset presents a significant challenge to NIDSs. In order to address this concern, this paper proposes a new NIDS called CBF-IDS, which combines convolutional neural networks (CNNs) and bidirectional long short-term memory networks (BiLSTMs) while employing the focal loss function. By utilizing CBF-IDS, spatial and temporal features can be extracted from network traffic. Moreover, during model training, CBF-IDS applies the focal loss function to give more weight to minority class samples, thereby mitigating the impact of class imbalance on model performance. In order to evaluate the effectiveness of CBF-IDS, experiments were conducted on three benchmark datasets: NSL-KDD, UNSW-NB15, and CIC-IDS2017. The experimental results demonstrate that CBF-IDS outperforms other classification models, achieving superior detection performance.
更多查看译文
关键词
intrusion detection system,class imbalance,convolutional neural network,bidirectional long short-term memory,focal loss
AI 理解论文
溯源树
样例
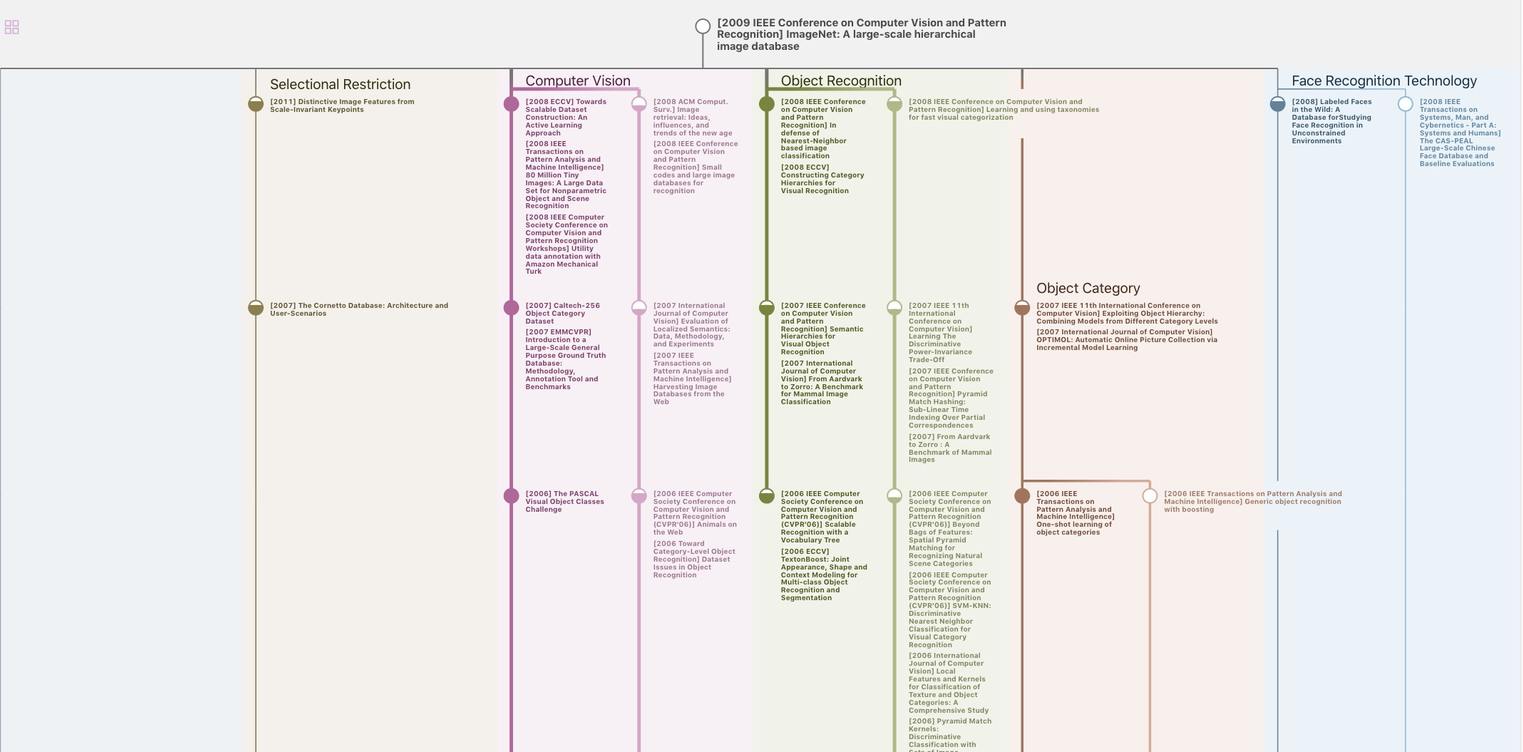
生成溯源树,研究论文发展脉络
Chat Paper
正在生成论文摘要