Deep Learning-Based Depression Detection from Social Media: Comparative Evaluation of ML and Transformer Techniques
Electronics(2023)
摘要
Detecting depression from user-generated content on social media platforms has garnered significant attention due to its potential for the early identification and monitoring of mental health issues. This paper presents a comprehensive approach for depression detection from user tweets using machine learning techniques. The study utilizes a dataset of 632,000 tweets and employs data preprocessing, feature selection, and model training with logistic regression, Bernoulli Naive Bayes, random forests, DistilBERT, SqueezeBERT, DeBERTA, and RoBERTa models. Evaluation metrics such as accuracy, precision, recall, and F1 score are employed to assess the models’ performance. The results indicate that the RoBERTa model achieves the highest accuracy ratio of 0.981 and the highest mean accuracy of 0.97 (across 10 cross-validation folds) in detecting depression from tweets. This research demonstrates the effectiveness of machine learning and advanced transformer-based models in leveraging social media data for mental health analysis. The findings offer valuable insights into the potential for early detection and monitoring of depression using online platforms, contributing to the growing field of mental health analysis based on user-generated content.
更多查看译文
关键词
depression detection,social media analysis,deep learning models,NLP techniques,user tweets,mental health identification
AI 理解论文
溯源树
样例
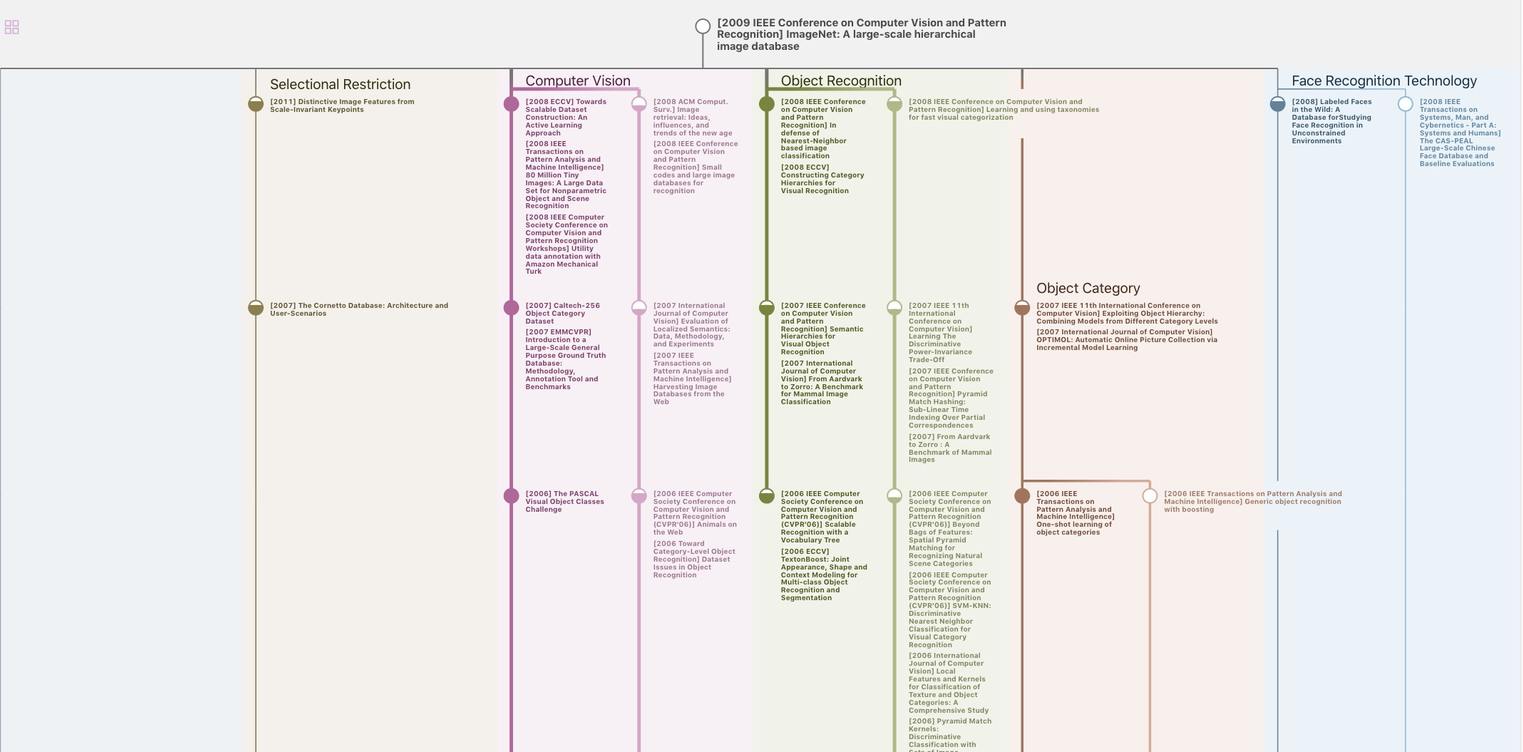
生成溯源树,研究论文发展脉络
Chat Paper
正在生成论文摘要