EEG-Based Functional Connectivity Analysis for Cognitive Impairment Classification
Electronics(2023)
摘要
Understanding how mild cognitive impairment affects global neural networks may explain changes in brain electrophysiology. Using graph theory and the visual oddball paradigm, we evaluated the functional connectivity of neuronal networks in brain lobes. The study involved 30 participants: 14 with mild cognitive impairment (MCI) and 16 healthy control (HC) participants. We conducted an examination using the visual oddball paradigm, focusing on electroencephalography signals with targeted stimuli. Our analysis employed functional connectivity utilizing the change point detection method. Additionally, we implemented training for linear discriminant analysis, K-nearest neighbor, and decision tree techniques to classify brain activity, distinguishing between subjects with mild cognitive impairment and those in the healthy control group. Our results demonstrate the efficacy of combining functional connectivity measurements derived from electroencephalography with machine learning for cognitive impairment classification. This research opens avenues for further exploration, including the potential for real-time detection of cognitive decline in complex real-world scenarios.
更多查看译文
关键词
brain networks,computational modeling,EEG,neurodegenerative disease,machine learning,change point detection
AI 理解论文
溯源树
样例
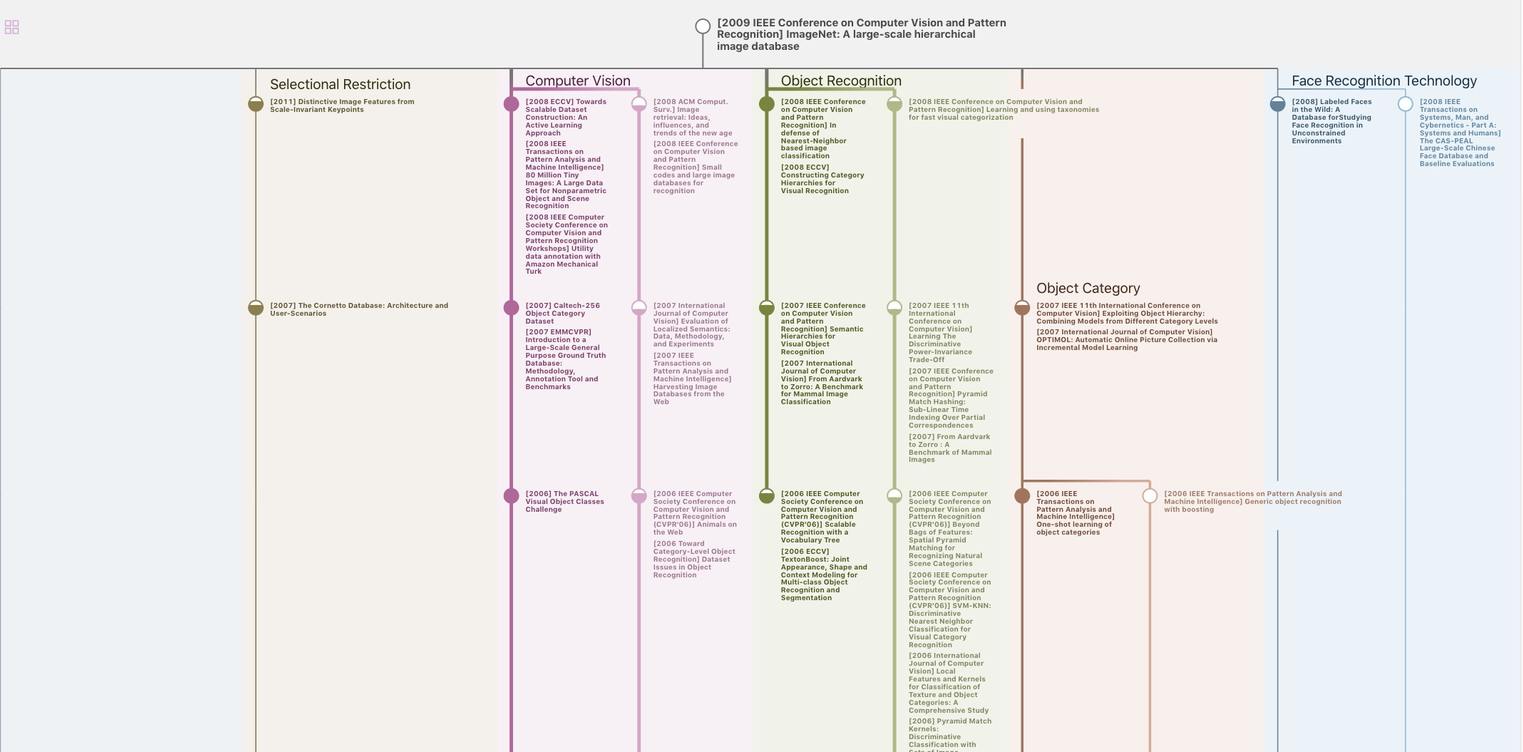
生成溯源树,研究论文发展脉络
Chat Paper
正在生成论文摘要